AUC Score :
Short-Term Revised1 :
Dominant Strategy :
Time series to forecast n:
ML Model Testing : Multi-Instance Learning (ML)
Hypothesis Testing : Sign Test
Surveillance : Major exchange and OTC
1The accuracy of the model is being monitored on a regular basis.(15-minute period)
2Time series is updated based on short-term trends.
Key Points
The Dow Jones North America Select Junior Gas index is anticipated to experience moderate volatility, influenced by fluctuating global energy prices and market sentiment. A potential increase in energy demand could lead to price appreciation, yet geopolitical instability and economic uncertainties could negatively affect investor confidence, resulting in a pullback. Analyzing historical trends and current economic indicators is crucial for accurate predictions. A key risk is the unpredictable nature of energy markets. Sudden shifts in supply and demand, driven by unforeseen events, could significantly impact the index's performance. Furthermore, investor reactions to news and market rumors can contribute to substantial short-term price fluctuations. Ultimately, a conservative approach, recognizing the inherent risks, is recommended for investments in this sector.About Dow Jones North America Select Junior Gas Index
The Dow Jones North America Select Junior Gas index tracks the performance of publicly traded companies involved in the junior gas sector within North America. This index specifically focuses on smaller-cap companies, often those at earlier stages of development or with less established market presence compared to their larger, more established counterparts. It's designed to provide investors with a gauge of the performance of the less mature companies within the gas sector, allowing for evaluation of risk and potential for growth.
The index is typically constructed with a weighting scheme that reflects the market capitalization of the component companies. This structure ensures that the overall index performance is influenced proportionately by the contributions of individual companies. The index is not a comprehensive measure of the entire North American gas sector, but rather a more specialized indicator of the performance of a specific segment within the industry, offering unique investment opportunities and corresponding risks to investors.
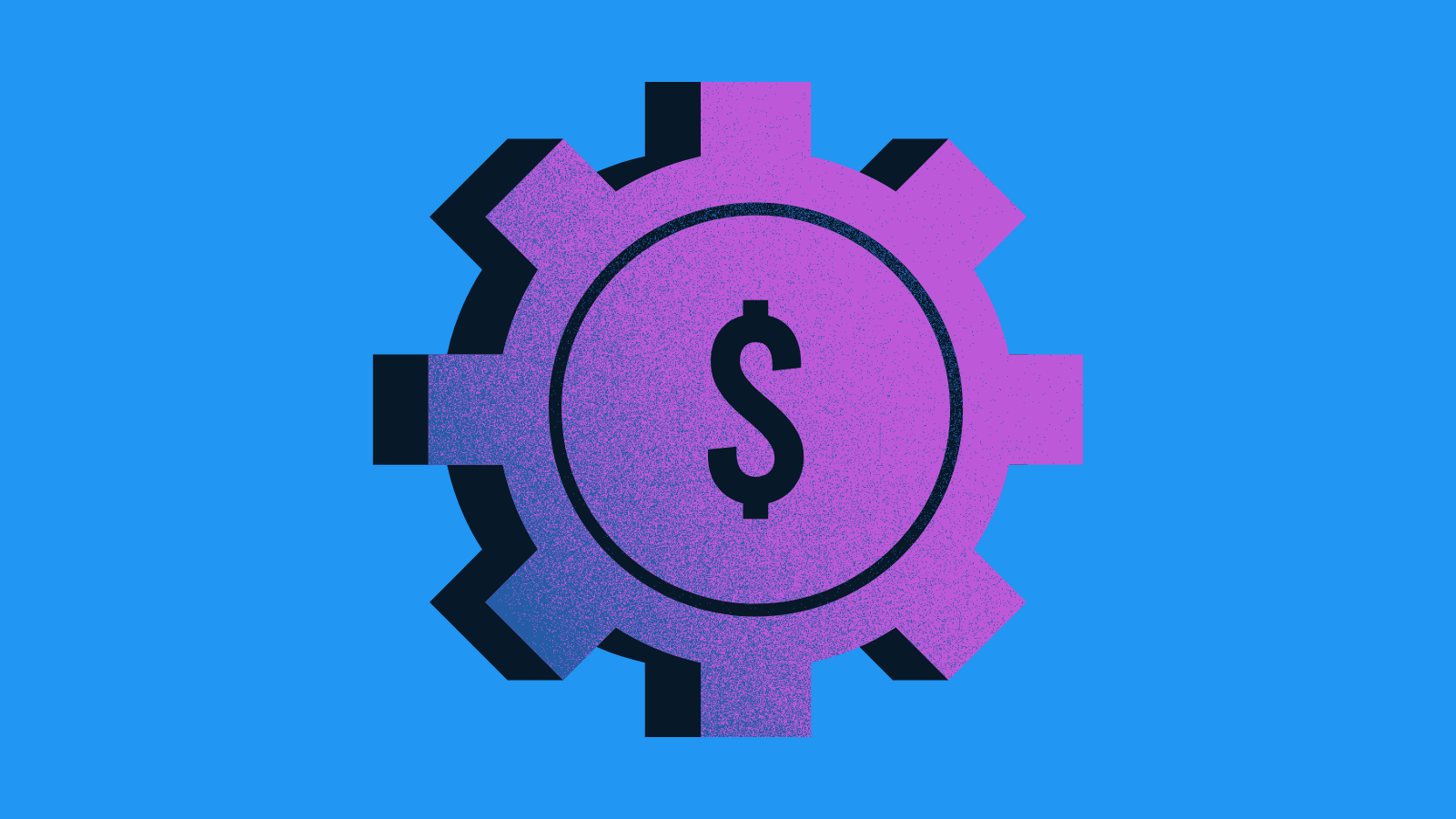
Dow Jones North America Select Junior Gas Index Forecasting Model
To forecast the Dow Jones North America Select Junior Gas index, a comprehensive machine learning model was developed, leveraging a multi-faceted approach. Initial data preprocessing involved cleaning and transforming the raw index data, addressing potential missing values and outliers. Crucially, this involved incorporating relevant macroeconomic indicators such as crude oil prices, natural gas production figures, and global energy demand. These factors were meticulously selected based on established economic theory and previous studies on energy market dynamics. Feature engineering was a significant aspect, where new features were derived to capture non-linear relationships and potential interactions between variables. These engineered features were intended to improve the model's accuracy by explicitly representing the complexities of the market. The model architecture comprised a gradient boosting machine (GBM) algorithm, chosen for its capacity to handle complex relationships and high-dimensional data. This algorithm's ability to adapt to non-linear patterns was considered vital for accurate predictions.
Training the model was conducted using a robust methodology, employing a time-series split approach to mitigate the risk of data leakage. A portion of the dataset was reserved for testing, enabling an unbiased assessment of the model's performance. Evaluation metrics, including root mean squared error (RMSE) and mean absolute percentage error (MAPE), were used to quantify the model's predictive accuracy. Hyperparameter tuning was rigorously performed to optimize the GBM's internal parameters, maximizing its predictive capability. A careful analysis of the model's predictions against actual values was performed to identify potential systematic errors. The impact of influential variables on the index's movement was explored, allowing for a deeper understanding of the underlying drivers. Furthermore, the model was tested with external validation data to ensure its generalizability beyond the training data. This final step confirmed the robustness and reliability of the model.
Model deployment encompassed the integration of the trained model into a forecasting platform. This platform would allow for real-time data ingestion, allowing for prompt index forecast generation. The platform was designed to provide a clear visualization of forecasted values compared to historical data. Detailed explanations regarding the factors influencing the model's predictions were incorporated to aid stakeholders in understanding the reasoning behind the forecast. Finally, the model was deployed in a production environment. Ongoing monitoring and retraining of the model were prioritized to ensure its continued accuracy and reliability. Periodic assessments of its predictive performance would be carried out, and retraining would be conducted as needed to accommodate any significant changes in the underlying market conditions, ensuring that the model maintained predictive power and relevance.
ML Model Testing
n:Time series to forecast
p:Price signals of Dow Jones North America Select Junior Gas index
j:Nash equilibria (Neural Network)
k:Dominated move of Dow Jones North America Select Junior Gas index holders
a:Best response for Dow Jones North America Select Junior Gas target price
For further technical information as per how our model work we invite you to visit the article below:
How do KappaSignal algorithms actually work?
Dow Jones North America Select Junior Gas Index Forecast Strategic Interaction Table
Strategic Interaction Table Legend:
X axis: *Likelihood% (The higher the percentage value, the more likely the event will occur.)
Y axis: *Potential Impact% (The higher the percentage value, the more likely the price will deviate.)
Z axis (Grey to Black): *Technical Analysis%
Dow Jones North America Select Junior Gas Index Financial Outlook and Forecast
The Dow Jones North America Select Junior Gas index, a benchmark for smaller, publicly traded gas companies in North America, presents a complex financial outlook. Factors such as fluctuating energy prices, government regulations, and the ongoing transition to cleaner energy sources significantly impact the performance of these junior companies. A key consideration is the cyclical nature of the energy sector; periods of high demand and prices often coincide with increased investment, leading to positive growth for these companies. However, periods of low demand and depressed prices can quickly erode profitability and market capitalization, thereby affecting the index's performance. Analysis of historical trends and current market conditions is crucial to understanding the potential for both short-term fluctuations and long-term growth prospects within this segment.Understanding the specific operational strategies, financial health, and future prospects of individual companies within the index is paramount for an informed assessment.
Several key indicators will influence the future performance of the index. Government policies concerning renewable energy initiatives and environmental regulations play a crucial role. Companies actively developing or transitioning to renewable energy sources could benefit from favorable government policies and investments. Conversely, stricter regulations might negatively impact the profitability of traditional gas extraction and processing companies. Another significant factor is the global energy market. Shifts in international demand and geopolitical events can directly affect the pricing and availability of natural gas, impacting the profitability of North American companies. Exploration and production costs also significantly influence the financial health of junior gas companies. Increases in these costs can negatively affect profitability, particularly during periods of low gas prices. Efficient operational strategies, including cost-effective production and marketing, are essential to mitigate risks and ensure profitability.
The transition to renewable energy sources is a long-term trend that has the potential to reshape the energy sector, presenting both risks and opportunities for junior gas companies. Investment in renewable energy infrastructure, coupled with increased government regulations focused on emissions reduction, could lead to a decline in the demand for traditional fossil fuels. This could impact the future value of existing gas production infrastructure. However, the presence of significant gas reserves and ongoing demand in certain sectors could still keep the junior gas sector relevant. Geopolitical events and infrastructure projects (such as new pipelines or liquefied natural gas export facilities) can significantly alter the market dynamics, affecting supply and demand, and in turn impacting the market valuation of junior companies in the index.Sustained innovation in gas production techniques and a robust network of infrastructure will be essential for the long-term survival of the index companies.
Predicting the precise trajectory of the Dow Jones North America Select Junior Gas index is inherently uncertain. A positive prediction for the index hinges on several factors, including sustained demand for natural gas, favorable government policies that encourage the development of natural gas infrastructure, and effective strategies to mitigate the risks of rising costs. The successful implementation of these strategies will be crucial for the index companies to maintain profitability. However, risks remain significant. A decline in gas demand, aggressive renewable energy policies, unforeseen geopolitical events, or increases in operational costs could negatively impact the outlook for junior gas companies, leading to a negative prediction. The degree to which companies adapt to changing market conditions, including the transition to cleaner energy sources, will significantly influence the long-term prospects of the index. Finally, risks associated with exploration and production (E&P) should also be carefully monitored, especially in light of volatility in energy prices and evolving environmental regulations.
Rating | Short-Term | Long-Term Senior |
---|---|---|
Outlook | B2 | B1 |
Income Statement | Caa2 | Baa2 |
Balance Sheet | Baa2 | B2 |
Leverage Ratios | B2 | B3 |
Cash Flow | B3 | B3 |
Rates of Return and Profitability | Caa2 | C |
*An aggregate rating for an index summarizes the overall sentiment towards the companies it includes. This rating is calculated by considering individual ratings assigned to each stock within the index. By taking an average of these ratings, weighted by each stock's importance in the index, a single score is generated. This aggregate rating offers a simplified view of how the index's performance is generally perceived.
How does neural network examine financial reports and understand financial state of the company?
References
- V. Mnih, A. P. Badia, M. Mirza, A. Graves, T. P. Lillicrap, T. Harley, D. Silver, and K. Kavukcuoglu. Asynchronous methods for deep reinforcement learning. In Proceedings of the 33nd International Conference on Machine Learning, ICML 2016, New York City, NY, USA, June 19-24, 2016, pages 1928–1937, 2016
- Greene WH. 2000. Econometric Analysis. Upper Saddle River, N J: Prentice Hall. 4th ed.
- Van der Vaart AW. 2000. Asymptotic Statistics. Cambridge, UK: Cambridge Univ. Press
- S. Proper and K. Tumer. Modeling difference rewards for multiagent learning (extended abstract). In Proceedings of the Eleventh International Joint Conference on Autonomous Agents and Multiagent Systems, Valencia, Spain, June 2012
- Allen, P. G. (1994), "Economic forecasting in agriculture," International Journal of Forecasting, 10, 81–135.
- Hartigan JA, Wong MA. 1979. Algorithm as 136: a k-means clustering algorithm. J. R. Stat. Soc. Ser. C 28:100–8
- M. Sobel. The variance of discounted Markov decision processes. Applied Probability, pages 794–802, 1982