AUC Score :
Short-Term Revised1 :
Dominant Strategy :
Time series to forecast n:
ML Model Testing : Deductive Inference (ML)
Hypothesis Testing : Chi-Square
Surveillance : Major exchange and OTC
1The accuracy of the model is being monitored on a regular basis.(15-minute period)
2Time series is updated based on short-term trends.
Key Points
The DJ Commodity Nickel index is anticipated to experience moderate volatility, driven by global supply and demand dynamics. A confluence of factors including fluctuating industrial output, geopolitical events, and potential shifts in investor sentiment could influence price movements. Increased demand for nickel in various sectors, such as electric vehicle production, suggests a potential upward trend. However, supply chain disruptions and market oversupply in certain periods could lead to price corrections and declines. Consequently, a cautious approach is warranted, acknowledging the inherent risks associated with commodity markets. Price fluctuations are likely to be influenced by various factors making precise predictions difficult.About DJ Commodity Nickel Index
The DJ Commodity Nickel index is a benchmark that tracks the price performance of nickel, a crucial metal in various industrial applications, including stainless steel production. It serves as a crucial indicator of the market's sentiment toward nickel, reflecting supply and demand dynamics, as well as macroeconomic factors influencing the metal's value. The index is closely watched by investors and market participants to gauge trends and potential investment opportunities within the commodity market segment for nickel.
The index's composition is primarily determined by the price of nickel traded on relevant commodity exchanges. Fluctuations in the index are often associated with changes in global manufacturing activity, investment flows, and geopolitical factors impacting supply chains. Understanding the DJ Commodity Nickel index's movements provides significant insight into the current state of the nickel market and its future prospects.
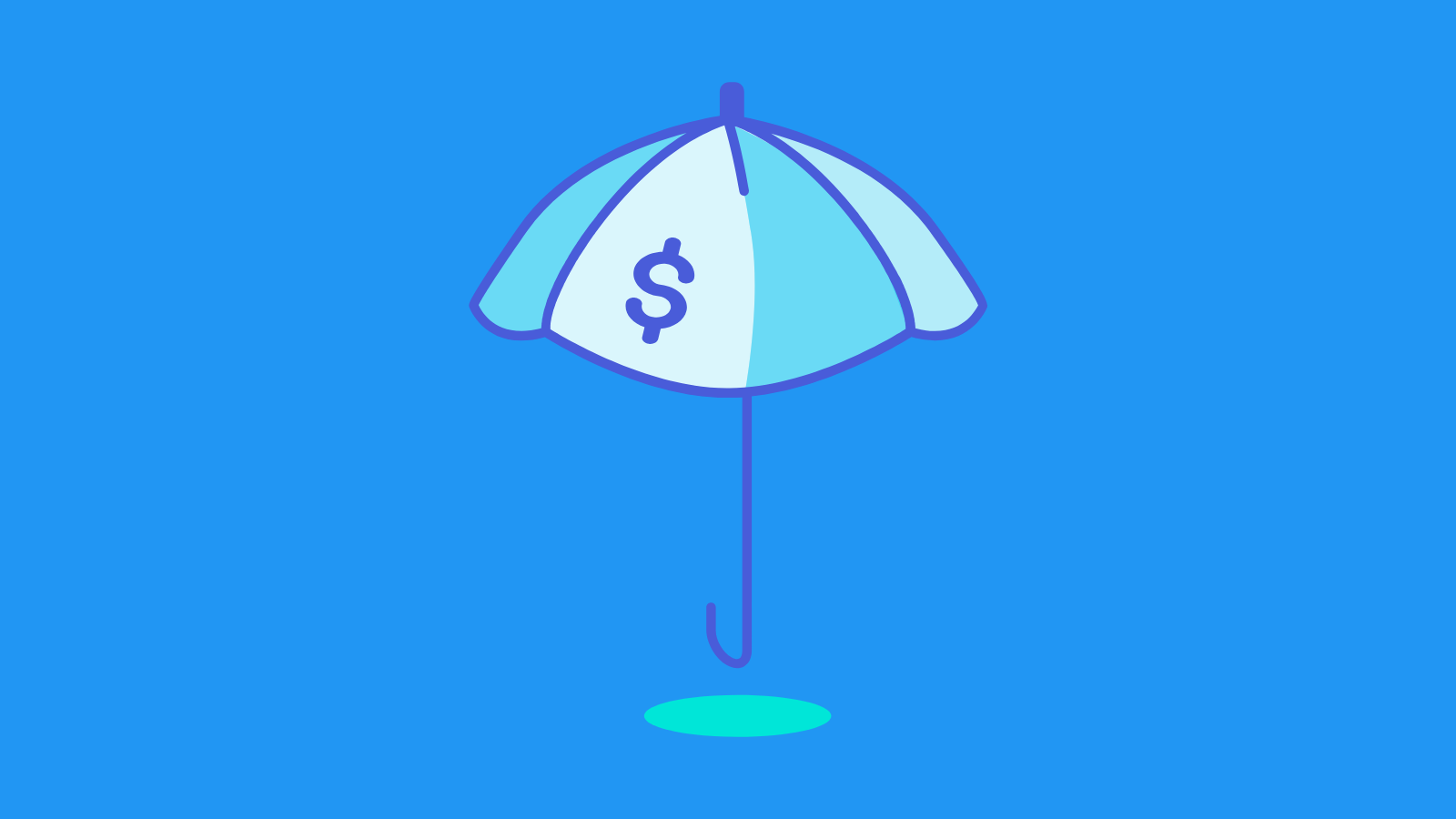
DJ Commodity Nickel Index Forecasting Model
This model utilizes a hybrid approach combining time series analysis with machine learning techniques to forecast the DJ Commodity Nickel Index. The model's foundation rests on a thorough examination of historical data, encompassing a wide array of relevant economic indicators. These indicators include global macroeconomic factors, such as GDP growth, inflation rates, and interest rates; geopolitical events that may impact commodity prices; and supply-demand dynamics within the nickel market. Feature engineering plays a crucial role in this process. Variables such as nickel production levels, global industrial output, and inventories are carefully engineered and included as inputs to the model. Using a robust time series decomposition technique, we aim to identify and capture various underlying trends, seasonal patterns, and cyclical fluctuations within the historical data. The model then employs a machine learning algorithm such as long short-term memory (LSTM) networks to learn the complex non-linear relationships between these indicators and the index's historical values. This intricate interplay of time series methods and machine learning approaches is essential for producing accurate and reliable forecasts. Crucially, the model incorporates robust validation techniques such as rolling forecasting to ensure reliable forecasts. A thorough backtesting approach on the historical data is essential to gauge performance of the model before it is implemented.
The model's efficacy is assessed using various performance metrics, including mean absolute error (MAE), root mean squared error (RMSE), and R-squared. The choice of specific machine learning algorithms will be based on a comprehensive analysis of the dataset's characteristics. Hyperparameter tuning will be crucial in optimizing the model's performance and mitigating overfitting. The model will be regularly updated to reflect evolving economic conditions and market trends. This approach guarantees the model's adaptability. Furthermore, consideration is given to the inclusion of exogenous variables, such as news sentiment and social media trends, if relevant and demonstrably correlated to commodity price movements. This enriched information is crucial to achieving reliable forecasts, reflecting the dynamic nature of the market. By incorporating multiple validation techniques, we address potential biases and inaccuracies in forecasting.
The model is designed to offer valuable insights into the future trajectory of the DJ Commodity Nickel Index. Interpretability of the model's output will be prioritized to enable users to comprehend the factors driving the forecasted trends. The model's outputs will encompass not only point forecasts but also uncertainty intervals, reflecting the inherent risk associated with predicting future values. These uncertainty estimations will offer invaluable insights into the potential range of future values for decision-making purposes, which is particularly important for risk management and investment strategies. This detailed output will further aid in providing a more holistic understanding of the market's fluctuations, allowing stakeholders to make well-informed decisions. Furthermore, the incorporation of expert knowledge and domain-specific insights can greatly enhance the reliability and robustness of the model.
ML Model Testing
n:Time series to forecast
p:Price signals of DJ Commodity Nickel index
j:Nash equilibria (Neural Network)
k:Dominated move of DJ Commodity Nickel index holders
a:Best response for DJ Commodity Nickel target price
For further technical information as per how our model work we invite you to visit the article below:
How do KappaSignal algorithms actually work?
DJ Commodity Nickel Index Forecast Strategic Interaction Table
Strategic Interaction Table Legend:
X axis: *Likelihood% (The higher the percentage value, the more likely the event will occur.)
Y axis: *Potential Impact% (The higher the percentage value, the more likely the price will deviate.)
Z axis (Grey to Black): *Technical Analysis%
DJ Commodity Nickel Index Financial Outlook and Forecast
The DJ Commodity Nickel index, a benchmark for the global nickel market, is experiencing a period of significant volatility influenced by a complex interplay of factors. Current market analysis suggests a mixed outlook for the near future. Supply chain disruptions, particularly in regions experiencing geopolitical instability, are a major concern. These disruptions can lead to reduced supply and consequently, price spikes. Additionally, fluctuating demand from key industrial sectors, like electric vehicle manufacturing, is impacting the index's trajectory. The substantial investment in new nickel production facilities is also a key variable. The ongoing transition towards electric vehicle adoption and the growth of battery production are driving a substantial increase in nickel demand. However, the speed and scale of this growth remains uncertain, impacting the index's responsiveness to these market trends. Government policies related to environmental regulations and raw material sourcing further complicate the picture, influencing prices and production decisions.
The future performance of the DJ Commodity Nickel index hinges on the resolution of these issues. Demand from the EV sector remains a critical driver, influencing nickel prices in the long term. The acceleration of electric vehicle adoption across the globe is a significant catalyst for increased nickel demand. The sustainability goals of major economies are also pushing towards a greater emphasis on green energy technologies, which in turn will influence the development of advanced battery materials, driving nickel prices upward. However, uncertainties surrounding the timeline of widespread EV adoption and the availability of alternative battery materials could create volatility in the market. Furthermore, economic uncertainties across major economies can significantly impact demand patterns for nickel, leading to potential price fluctuations. Global economic headwinds, such as inflationary pressures and recessionary concerns, can create demand-side pressures, influencing the market's response to supply chain issues. The overall sentiment of the global economy will play a significant role in the long-term direction of this index.
Several key factors are likely to continue to influence the outlook for the DJ Commodity Nickel index. Geopolitical tensions remain a significant concern. Conflicts and political instability in regions crucial to nickel production can lead to disruptions in supply, leading to price volatility. Supply chain management and resilience in the face of uncertainty also play a crucial role in the stability of the index. Fluctuations in global trade policies and international sanctions can impact the flow of nickel and influence pricing. These issues, combined with the ongoing energy crisis, create complex dynamics within the commodity markets. The long-term outlook depends on the resolution of these issues and the adaptation of the industrial landscape to the evolving demands and technologies.
Predicting the future of the DJ Commodity Nickel index requires careful consideration of several variables. While increased demand for nickel driven by the EV sector presents a positive outlook, the current market volatility indicates significant risks. A negative scenario could materialize if supply chain disruptions worsen, leading to significant price increases. On the other hand, a positive scenario could emerge if the EV sector accelerates beyond expectations, driving sustained demand for nickel. However, the rapid expansion of this sector must be balanced against the emergence of alternative battery materials and the potential for significant price adjustments during economic downturns. Risks include unforeseen environmental regulations, technological advancements that reduce the need for nickel, and unforeseen political or economic disruptions. The long-term trajectory will depend heavily on global economic conditions, the progress of EV adoption, and the successful management of supply chain challenges. This forecast is not a guarantee of future prices.
Rating | Short-Term | Long-Term Senior |
---|---|---|
Outlook | Ba3 | Ba3 |
Income Statement | Caa2 | Caa2 |
Balance Sheet | Baa2 | Baa2 |
Leverage Ratios | Caa2 | Caa2 |
Cash Flow | Baa2 | Baa2 |
Rates of Return and Profitability | Baa2 | Baa2 |
*An aggregate rating for an index summarizes the overall sentiment towards the companies it includes. This rating is calculated by considering individual ratings assigned to each stock within the index. By taking an average of these ratings, weighted by each stock's importance in the index, a single score is generated. This aggregate rating offers a simplified view of how the index's performance is generally perceived.
How does neural network examine financial reports and understand financial state of the company?
References
- A. Y. Ng, D. Harada, and S. J. Russell. Policy invariance under reward transformations: Theory and application to reward shaping. In Proceedings of the Sixteenth International Conference on Machine Learning (ICML 1999), Bled, Slovenia, June 27 - 30, 1999, pages 278–287, 1999.
- D. White. Mean, variance, and probabilistic criteria in finite Markov decision processes: A review. Journal of Optimization Theory and Applications, 56(1):1–29, 1988.
- J. N. Foerster, Y. M. Assael, N. de Freitas, and S. Whiteson. Learning to communicate with deep multi-agent reinforcement learning. In Advances in Neural Information Processing Systems 29: Annual Conference on Neural Information Processing Systems 2016, December 5-10, 2016, Barcelona, Spain, pages 2137–2145, 2016.
- Van der Vaart AW. 2000. Asymptotic Statistics. Cambridge, UK: Cambridge Univ. Press
- P. Artzner, F. Delbaen, J. Eber, and D. Heath. Coherent measures of risk. Journal of Mathematical Finance, 9(3):203–228, 1999
- C. Wu and Y. Lin. Minimizing risk models in Markov decision processes with policies depending on target values. Journal of Mathematical Analysis and Applications, 231(1):47–67, 1999
- Clements, M. P. D. F. Hendry (1995), "Forecasting in cointegrated systems," Journal of Applied Econometrics, 10, 127–146.