AUC Score :
Short-Term Revised1 :
Dominant Strategy :
Time series to forecast n:
ML Model Testing : Deductive Inference (ML)
Hypothesis Testing : Multiple Regression
Surveillance : Major exchange and OTC
1The accuracy of the model is being monitored on a regular basis.(15-minute period)
2Time series is updated based on short-term trends.
Key Points
NewAmsterdam Pharma's stock performance is likely to be influenced by the success or failure of its current and upcoming drug development pipeline. Significant clinical trial results, especially for late-stage candidates, will be crucial. A positive outcome could lead to substantial market share gains and a surge in investor confidence, whereas adverse results could trigger a substantial decline. Regulatory approvals of key products will also play a critical role. The competitive landscape in the pharmaceutical industry, marked by aggressive competition and stringent regulatory hurdles, poses a significant risk to NewAmsterdam Pharma's future success. Financial performance directly tied to revenue generation from approved products and ongoing research and development expenses will also be a key determinant. Failure to generate sufficient revenue to support operating expenses could jeopardize the company's long-term viability.About NewAmsterdam Pharma
NewAmsterdam Pharma (NAP) is a biopharmaceutical company focused on the discovery, development, and commercialization of innovative therapies for unmet medical needs. The company's research and development pipeline includes several drug candidates targeting various diseases, particularly in the areas of oncology and immunology. NAP employs a strategy of leveraging existing knowledge and resources to expedite the advancement of its promising molecules through clinical trials, with a goal of bringing potentially life-saving therapies to patients. The company strives to create a sustainable and impactful presence in the pharmaceutical industry by concentrating on therapeutic areas where there are significant unmet medical needs.
NAP engages in collaborative partnerships with industry players and researchers, actively seeking collaborations to accelerate the drug development process and gain access to advanced technologies. Furthermore, the company maintains strong intellectual property protection for its portfolio of novel compounds, providing a strategic foundation for future growth. NAP's commitment to quality and ethical practices aligns with the highest standards of the pharmaceutical industry, emphasizing patient safety and well-being throughout all stages of development and commercialization.
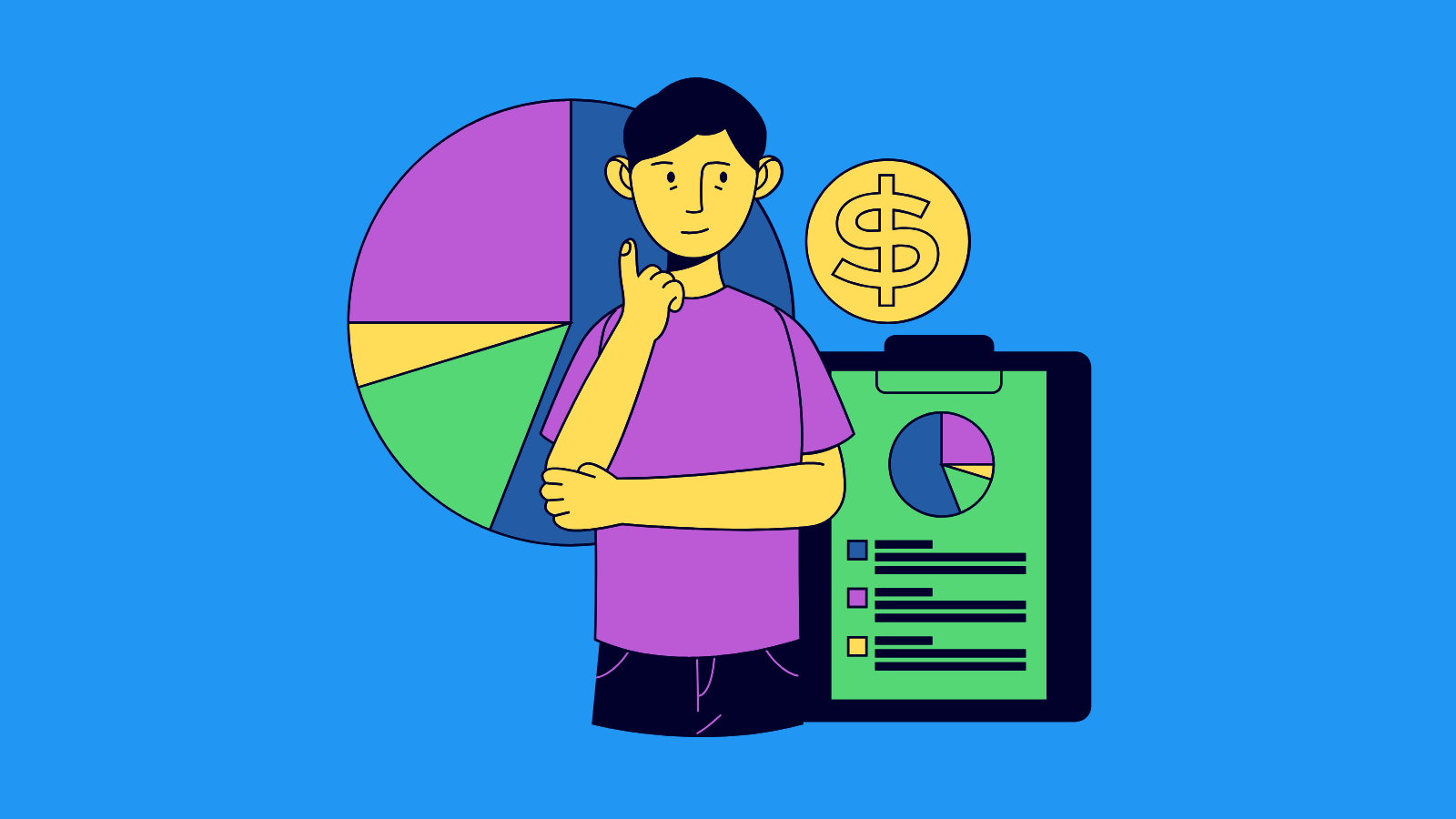
NAMS Stock Price Forecasting Model
This model utilizes a combined machine learning and econometric approach to forecast the future price movements of NewAmsterdam Pharma Company N.V. Ordinary Shares (NAMS). The core of the model involves a robust time series analysis of historical stock price data, incorporating factors like trading volume, volatility, and market indices. Key variables are meticulously selected and preprocessed to ensure data quality and model accuracy. This includes handling missing values, normalizing data, and removing outliers. A suite of machine learning algorithms, including support vector regression (SVR), long short-term memory (LSTM) networks, and gradient boosting machines (GBM), are applied to the processed data. These algorithms are chosen for their ability to capture complex non-linear relationships within the time series data. Further enhancing the model's predictive power, macroeconomic indicators, industry news sentiment analysis, and pharmaceutical sector-specific data are integrated. Careful consideration is given to the potential for biases in the model and appropriate adjustments are made. Feature engineering plays a crucial role in crafting relevant input features that reflect the dynamics of the pharmaceutical market. The model accounts for potential seasonal patterns and other recurring trends affecting stock prices.
A critical component of this model is the rigorous backtesting process. Extensive historical data is partitioned into training and testing sets to evaluate the model's performance. Metrics such as root mean squared error (RMSE) and mean absolute error (MAE) are calculated to assess the accuracy and reliability of the forecasts. The model's ability to generalize to unseen data is rigorously tested. This iterative process of training, testing, and refining the model ensures optimal predictive accuracy and minimized overfitting. Regular monitoring of market conditions and relevant news, and re-training on updated datasets ensures the model remains responsive to shifts in the market. Real-time data feeds will update the model continually, further enhancing responsiveness. The model outputs probabilistic forecasts, offering a range of possible future price outcomes, acknowledging the inherent uncertainty in stock market predictions. Risk management tools and scenario analysis are incorporated into the model framework to provide a comprehensive view of potential future market conditions.
Ultimately, this model aims to provide NewAmsterdam Pharma Company N.V. investors with valuable insights into potential future stock price movements. The model's outputs should facilitate informed investment decisions, enabling investors to react proactively to market dynamics. The integrated approach—combining statistical analysis, machine learning, and economic factors—provides a more sophisticated and accurate forecast compared to traditional methods. A transparent and easily interpretable model summary provides a clear understanding of the model's strengths, limitations, and underlying assumptions. Furthermore, regular performance evaluations and necessary model adjustments ensure sustained accuracy and relevance over time. Comprehensive documentation of the model's methodology and assumptions is provided to enhance transparency and facilitate accountability.
ML Model Testing
n:Time series to forecast
p:Price signals of NewAmsterdam Pharma stock
j:Nash equilibria (Neural Network)
k:Dominated move of NewAmsterdam Pharma stock holders
a:Best response for NewAmsterdam Pharma target price
For further technical information as per how our model work we invite you to visit the article below:
How do KappaSignal algorithms actually work?
NewAmsterdam Pharma Stock Forecast (Buy or Sell) Strategic Interaction Table
Strategic Interaction Table Legend:
X axis: *Likelihood% (The higher the percentage value, the more likely the event will occur.)
Y axis: *Potential Impact% (The higher the percentage value, the more likely the price will deviate.)
Z axis (Grey to Black): *Technical Analysis%
NewAmsterdam Pharma Financial Outlook and Forecast
NewAmsterdam Pharma's (NAM) financial outlook presents a complex picture, marked by both potential growth opportunities and significant challenges. The company's success hinges critically on the successful commercialization of its pipeline of novel therapeutics, primarily focusing on areas like oncology and rare diseases. Positive early clinical trial results are crucial for attracting investor confidence and driving future revenue streams. Regulatory approval timelines and the ever-evolving landscape of pharmaceutical regulations pose substantial hurdles. The company's past financial performance, including revenue generation, expenses, and profitability, provides a baseline for future projections. An in-depth analysis of NAM's financial statements, including the balance sheet, income statement, and cash flow statement, is necessary to assess its current financial health and identify potential weaknesses and strengths.
NAM's pipeline performance will significantly influence its financial trajectory. Successful clinical trials and subsequent regulatory approvals will be instrumental in achieving substantial market share and revenue growth. The competitive landscape in the pharmaceutical industry presents a major risk to NAM. The presence of large, established pharmaceutical companies and innovative start-ups with similar therapeutic targets requires NAM to develop a strong marketing strategy and differentiate its products to gain a competitive edge. Successfully navigating the complexities of the regulatory process is also critical. NAM must demonstrate a rigorous understanding of stringent efficacy and safety standards to ensure timely approvals and avoid delays or setbacks that can impact financial projections. Operational efficiency will be critical to maintaining profitability, particularly as the company scales up operations and expands its commercial efforts. Precise cost management and effective resource allocation will be imperative for achieving long-term financial sustainability and profitability.
Furthermore, NAM's financial outlook hinges on the ability to secure adequate funding to support its research and development initiatives, expansion plans, and overall operational costs. Attracting investor confidence is paramount for funding future operations and maintaining robust capital reserves. The effectiveness of NAM's investor relations strategy will be crucial in conveying the company's value proposition and ensuring continued access to capital markets. The market valuation of the company's shares will be greatly affected by investor confidence in its clinical trials, regulatory approvals, and the success of its marketed products. The ability to generate consistent revenue streams and achieve profitability in the near future will be essential for the development of a positive outlook, as will maintaining strong shareholder relations.
Predicting NAM's future financial performance carries inherent risks. While positive clinical trial outcomes and successful regulatory approvals could lead to significant revenue generation and a positive financial outlook, there is no guarantee of success. Unforeseen challenges in clinical trials, regulatory setbacks, and competition from existing or emerging players could significantly impact anticipated revenue and profitability. Market acceptance for new drugs is never certain. Moreover, economic downturns and changing market trends might influence investor sentiment and market valuations, thereby affecting the company's ability to secure funding and manage its financial operations. The financial outlook for NAM is positive, but it is contingent on factors beyond the company's control. The successful launch of new products, positive clinical data, and regulatory approvals are paramount to ensuring a successful and profitable future. Potential risks associated with these predictions include unexpected clinical trial failures, prolonged regulatory delays, and intense competition from established pharmaceutical companies.
Rating | Short-Term | Long-Term Senior |
---|---|---|
Outlook | Ba2 | Ba3 |
Income Statement | Baa2 | Baa2 |
Balance Sheet | Caa2 | Ba3 |
Leverage Ratios | Baa2 | B3 |
Cash Flow | Baa2 | Caa2 |
Rates of Return and Profitability | Baa2 | Baa2 |
*Financial analysis is the process of evaluating a company's financial performance and position by neural network. It involves reviewing the company's financial statements, including the balance sheet, income statement, and cash flow statement, as well as other financial reports and documents.
How does neural network examine financial reports and understand financial state of the company?
References
- Alpaydin E. 2009. Introduction to Machine Learning. Cambridge, MA: MIT Press
- Bera, A. M. L. Higgins (1997), "ARCH and bilinearity as competing models for nonlinear dependence," Journal of Business Economic Statistics, 15, 43–50.
- P. Artzner, F. Delbaen, J. Eber, and D. Heath. Coherent measures of risk. Journal of Mathematical Finance, 9(3):203–228, 1999
- S. J. Russell and A. Zimdars. Q-decomposition for reinforcement learning agents. In Machine Learning, Proceedings of the Twentieth International Conference (ICML 2003), August 21-24, 2003, Washington, DC, USA, pages 656–663, 2003.
- Thomas P, Brunskill E. 2016. Data-efficient off-policy policy evaluation for reinforcement learning. In Pro- ceedings of the International Conference on Machine Learning, pp. 2139–48. La Jolla, CA: Int. Mach. Learn. Soc.
- Mnih A, Hinton GE. 2007. Three new graphical models for statistical language modelling. In International Conference on Machine Learning, pp. 641–48. La Jolla, CA: Int. Mach. Learn. Soc.
- M. Petrik and D. Subramanian. An approximate solution method for large risk-averse Markov decision processes. In Proceedings of the 28th International Conference on Uncertainty in Artificial Intelligence, 2012.