AUC Score :
Short-Term Revised1 :
Dominant Strategy :
Time series to forecast n:
ML Model Testing : Active Learning (ML)
Hypothesis Testing : Wilcoxon Sign-Rank Test
Surveillance : Major exchange and OTC
1The accuracy of the model is being monitored on a regular basis.(15-minute period)
2Time series is updated based on short-term trends.
Key Points
Lisata Therapeutics' future performance hinges on the successful advancement of its pipeline of therapies, particularly the progress of its lead candidates through clinical trials. Positive trial results and regulatory approvals would significantly boost investor confidence and drive stock price appreciation. Conversely, setbacks in clinical trials, unfavorable regulatory decisions, or the emergence of significant safety concerns could severely impact the stock's value. Competition in the therapeutic area will also be a considerable factor. Investors should also monitor the company's financial health, including cash flow generation, and its ability to secure further funding, as this can affect its long-term viability. Financial performance and fundraising efforts are critical to sustained development and market penetration efforts.About Lisata Therapeutics
Lisata Therapeutics, a biotechnology company, focuses on developing novel therapeutics for the treatment of rare diseases. Their research and development efforts are primarily centered on identifying and addressing unmet medical needs in areas with limited treatment options. The company utilizes cutting-edge scientific approaches, aiming to translate promising preclinical findings into effective therapies for patients. Their pipeline of drug candidates is under various stages of clinical development, with a notable focus on their lead programs. The company's commitment to its mission is underpinned by its scientific team's expertise and dedicated efforts to advance innovative therapies.
Lisata's strategy emphasizes strategic collaborations and partnerships to leverage external resources and expertise. The company actively seeks collaborations to enhance its capabilities in drug discovery, development, and clinical trials. Through these partnerships, Lisata can efficiently leverage outside resources to accelerate its progress. Investor relations play a key role in building trust and support within the medical research community. Transparency and commitment to rigorous scientific processes are important cornerstones of Lisata's approach to drug development and therapeutic advancement.
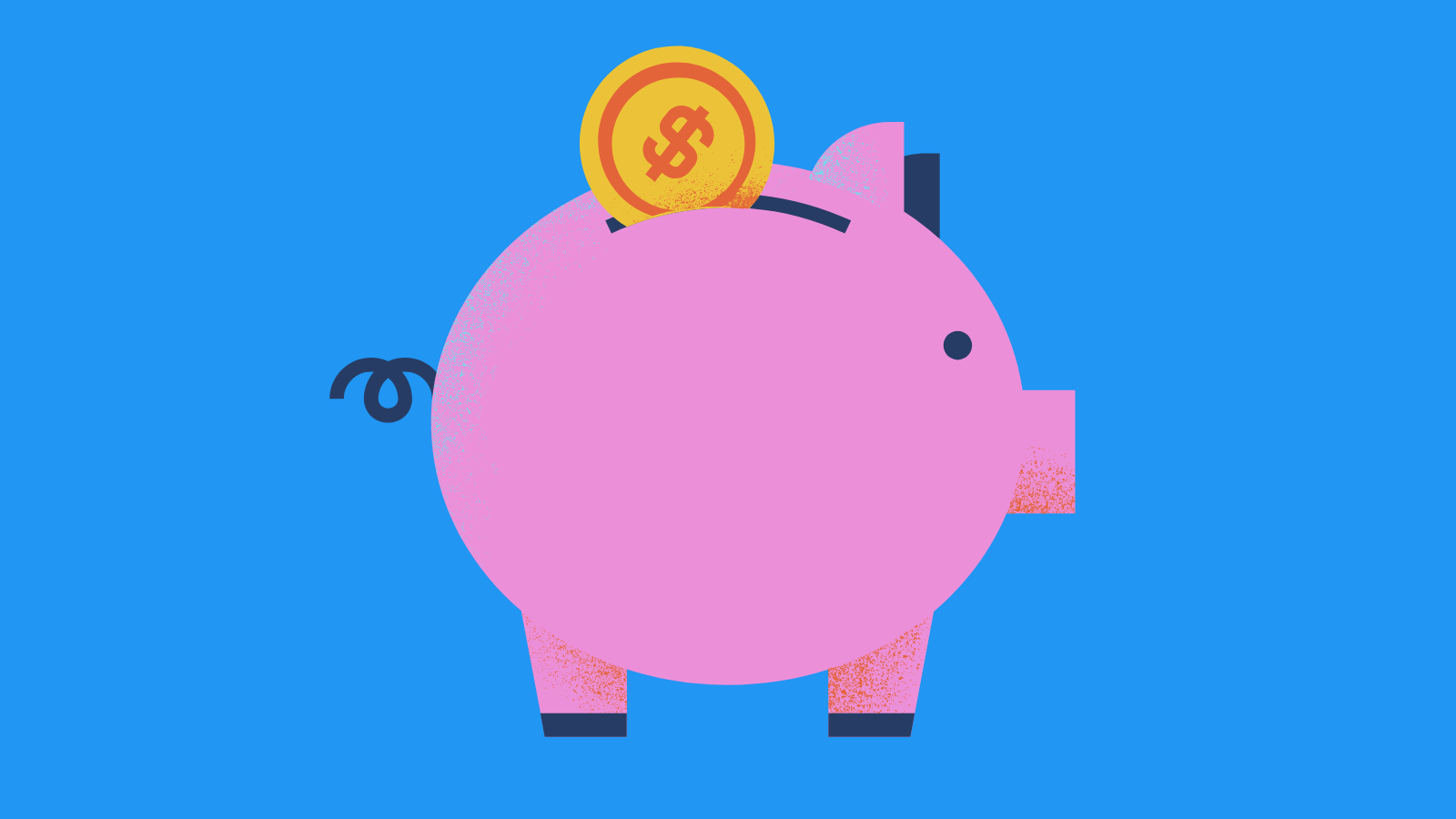
LSTA Stock Model Forecasting
This document outlines a proposed machine learning model for forecasting the future performance of Lisata Therapeutics Inc. (LSTA) common stock. The model leverages a multi-faceted approach, integrating fundamental financial data, market sentiment indicators, and macroeconomic factors. A comprehensive dataset will be compiled, encompassing historical stock prices, key financial ratios (e.g., earnings per share, revenue growth, debt-to-equity ratio), industry-specific news and publications, and macroeconomic indicators (e.g., GDP growth, interest rates, unemployment). Data preprocessing will include cleaning, feature engineering (creating new variables from existing ones, such as forward-looking revenue estimates, and incorporating external data on the industry's technological advancements), and normalization to ensure that all variables contribute equally to the model's training. A crucial component of this strategy is the incorporation of sentiment analysis from news articles and social media to capture the collective investor and market perception toward LSTA. This data will be meticulously processed to ensure its relevance and reliability in the forecasting process.
The model architecture will employ a recurrent neural network (RNN) specifically a Long Short-Term Memory (LSTM) network. LSTM networks excel in handling sequential data, making them suitable for capturing trends and patterns in financial time series. Training will involve splitting the dataset into training, validation, and testing sets. Performance will be evaluated through various metrics, including mean absolute error (MAE), root mean squared error (RMSE), and R-squared. The model will be tuned using hyperparameter optimization techniques to maximize its predictive accuracy on unseen data. Further model evaluation will involve backtesting, using historical data to assess the model's performance over different time horizons. The model will be regularly updated with new data to ensure its effectiveness and accuracy. An essential aspect of the process will be to document all steps of the data collection, processing, and modeling to ensure transparency and reproducibility. This will include thorough documentation of the selection of variables, the methodology employed in feature engineering and the model architecture.
The model's output will be a probability distribution or a point estimate for future LSTA stock price movements. This prediction will be accompanied by a confidence interval, allowing stakeholders to assess the uncertainty associated with the forecast. Regular reporting and validation are crucial to ensure the model's ongoing accuracy and relevance. The model's performance will be continuously monitored and re-evaluated, incorporating feedback and adjustments as necessary, ensuring the model remains a valuable tool for anticipating future price patterns and understanding market sentiment about LSTA. A detailed report outlining model performance and recommendations will be presented, providing an evidence-based forecasting strategy.
ML Model Testing
n:Time series to forecast
p:Price signals of Lisata Therapeutics stock
j:Nash equilibria (Neural Network)
k:Dominated move of Lisata Therapeutics stock holders
a:Best response for Lisata Therapeutics target price
For further technical information as per how our model work we invite you to visit the article below:
How do KappaSignal algorithms actually work?
Lisata Therapeutics Stock Forecast (Buy or Sell) Strategic Interaction Table
Strategic Interaction Table Legend:
X axis: *Likelihood% (The higher the percentage value, the more likely the event will occur.)
Y axis: *Potential Impact% (The higher the percentage value, the more likely the price will deviate.)
Z axis (Grey to Black): *Technical Analysis%
Lisata Therapeutics Inc. Financial Outlook and Forecast
Lisata Therapeutics' (LST) financial outlook presents a complex picture, characterized by significant uncertainty stemming from the early-stage nature of its drug development pipeline. The company's current focus is on advancing several drug candidates into clinical trials, a costly and lengthy process with no guarantee of success. Key financial metrics will likely be heavily influenced by the outcomes of these trials, including the successful completion of phase 1 and phase 2 trials. Revenue generation at this stage is almost entirely absent, relying solely on funding secured from investors to support research and development efforts. Significant capital expenditure is projected for ongoing research and development. Operational efficiency and diligent cost management will be crucial for sustained investor confidence and to mitigate potential financial strain. The company's financial strength is, therefore, fundamentally dependent on the successful development and commercialization of its drug candidates and the securing of additional funding. A lack of substantial revenue streams and a significant need for research funding pose a substantial financial challenge.
Forecasting LST's financial performance requires careful consideration of several variables. The progression of clinical trials, including positive and negative data, will directly influence investor sentiment and valuations. If the company's drug candidates demonstrate promising efficacy and safety profiles in clinical trials, the potential for future revenue streams could be substantial. Conversely, setbacks in trials could lead to decreased investor confidence and a potential downturn in the company's financial performance. Market acceptance of the drug candidates will also be a critical factor in determining the company's future financial success. The anticipated timeline for product development is crucial to understanding the cash flow requirements and the potential profitability in the long term. Potential partnerships or acquisitions with pharmaceutical companies could also offer significant financial benefits by accelerating development and potentially facilitating faster market entry.
An important aspect to consider is the overall competitive landscape. LST is operating in a highly competitive pharmaceutical market. Success will hinge upon demonstrating the superior efficacy and safety profiles of its drug candidates in comparison to existing treatments and those in development. The competitive landscape includes large pharmaceutical companies and other biotech firms actively pursuing similar therapeutic areas. This competition demands a robust strategy for intellectual property protection, strong marketing, and exceptional scientific evidence to gain market share. Strategic alliances and collaborations could be critical to enhance technological and financial resources in this competitive setting. In addition, regulatory hurdles, which are common in the pharmaceutical sector, pose additional uncertainties in the timelines and financial requirements associated with product approvals and commercialization. Regulatory approval processes are complex and lengthy, often leading to significant delays and uncertainty in anticipated timelines and financial outlays.
Predicting LST's financial outlook involves a degree of optimism balanced with substantial risk. A positive prediction hinges on successfully completing clinical trials, demonstrating robust efficacy and safety, and securing regulatory approvals. These achievements could translate into significant market share and sustained financial growth. However, this prediction carries considerable risk. The risk of failure in clinical trials is substantial, resulting in the potential loss of substantial financial investment. Regulatory hurdles and competition from other pharmaceutical companies could also severely limit market access. Additional funding requirements may prove difficult to secure if trial results are not positive. In this context, a negative outlook would stem from setbacks in clinical trials, unfavorable regulatory decisions, or inability to secure further financing. The uncertainty inherent in early-stage drug development demands cautious optimism and continuous evaluation of risk factors for a nuanced understanding of the potential financial trajectory of Lisata Therapeutics.
Rating | Short-Term | Long-Term Senior |
---|---|---|
Outlook | B2 | B2 |
Income Statement | B3 | B2 |
Balance Sheet | Ba3 | Caa2 |
Leverage Ratios | B3 | Caa2 |
Cash Flow | Baa2 | C |
Rates of Return and Profitability | Caa2 | Ba1 |
*Financial analysis is the process of evaluating a company's financial performance and position by neural network. It involves reviewing the company's financial statements, including the balance sheet, income statement, and cash flow statement, as well as other financial reports and documents.
How does neural network examine financial reports and understand financial state of the company?
References
- Hartford J, Lewis G, Taddy M. 2016. Counterfactual prediction with deep instrumental variables networks. arXiv:1612.09596 [stat.AP]
- N. B ̈auerle and J. Ott. Markov decision processes with average-value-at-risk criteria. Mathematical Methods of Operations Research, 74(3):361–379, 2011
- Burkov A. 2019. The Hundred-Page Machine Learning Book. Quebec City, Can.: Andriy Burkov
- Y. Le Tallec. Robust, risk-sensitive, and data-driven control of Markov decision processes. PhD thesis, Massachusetts Institute of Technology, 2007.
- Burgess, D. F. (1975), "Duality theory and pitfalls in the specification of technologies," Journal of Econometrics, 3, 105–121.
- D. Bertsekas. Nonlinear programming. Athena Scientific, 1999.
- Dietterich TG. 2000. Ensemble methods in machine learning. In Multiple Classifier Systems: First International Workshop, Cagliari, Italy, June 21–23, pp. 1–15. Berlin: Springer