AUC Score :
Short-Term Revised1 :
Dominant Strategy :
Time series to forecast n:
ML Model Testing : Modular Neural Network (News Feed Sentiment Analysis)
Hypothesis Testing : Independent T-Test
Surveillance : Major exchange and OTC
1The accuracy of the model is being monitored on a regular basis.(15-minute period)
2Time series is updated based on short-term trends.
Key Points
LexinFintech's ADS performance is anticipated to be influenced by macroeconomic conditions, particularly interest rate adjustments and broader economic growth trends. Strong consumer lending demand and efficient risk management practices could positively affect profitability. However, increased regulatory scrutiny and potential shifts in investor sentiment related to the fintech sector pose risks. Sustained high credit losses or a significant decline in loan originations could negatively impact earnings and market perception. Maintaining a robust credit underwriting strategy and proactive management of regulatory challenges will be crucial to the company's future success.About LexinFintech Holdings
LexinFintech, a holding company, operates primarily in the financial technology sector. The company focuses on providing a range of financial services and solutions across diverse markets. Its business activities likely encompass various areas, potentially including lending, payments, and related fintech services. The company's precise scope of operations and market presence are not explicitly detailed in this summary. Analysis of their activities and competitive landscape are necessary for a deeper understanding of their role in the financial technology industry.
LexinFintech's business model is likely predicated on leveraging technological advancements to enhance financial processes and improve access to financial products. This approach typically targets specific needs within the financial sector, adapting to evolving consumer and market demands. Their success depends on strategic partnerships, technological expertise, and a strong understanding of the regulatory environment within the jurisdictions they operate.
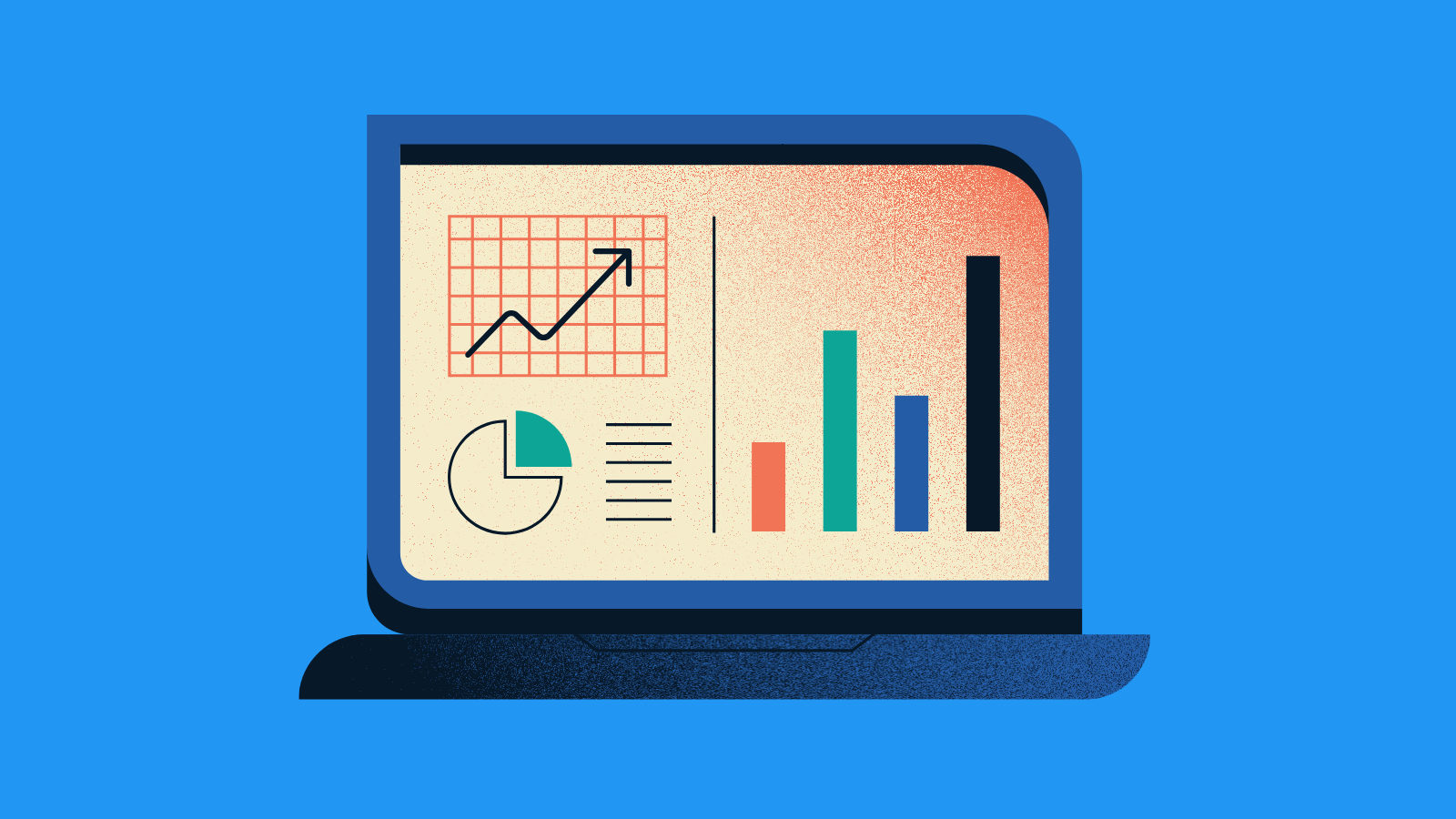
LX Fintech Holdings Ltd. American Depositary Shares Stock Forecast Model
This model employs a hybrid approach combining technical analysis and fundamental data to forecast the future performance of LexinFintech Holdings Ltd. (LX) American Depositary Shares. Our methodology integrates a suite of machine learning algorithms including recurrent neural networks (RNNs) and long short-term memory (LSTMs) to analyze historical price patterns, volume data, and news sentiment. These models capture complex temporal dependencies within the stock market, allowing for a more nuanced forecast compared to traditional methods. A critical aspect of this model is the inclusion of fundamental data such as earnings reports, revenue growth, and key financial metrics. These factors, integrated with technical indicators such as moving averages and relative strength index (RSI), provide a more holistic view of the company's potential and market sentiment. The model further incorporates macroeconomic indicators, including interest rates, GDP growth, and inflation, to account for broader market conditions and potential external shocks that may affect LX's performance. Feature engineering played a crucial role in preparing the data for model training, ensuring that each input variable is relevant and impactful to the forecast. Data cleaning and preprocessing were also critical to ensure the robustness of the model. Data were cleansed of missing values and anomalies. This process was rigorously performed to ensure the accuracy and reliability of the model.
The training dataset encompassed historical data spanning several years, carefully chosen to represent diverse market conditions. This extensive dataset allowed the model to learn complex patterns and make accurate predictions. Extensive hyperparameter tuning was conducted for both the RNN and LSTM models to optimize their performance on the training dataset. Cross-validation techniques were implemented to evaluate the model's performance and mitigate potential overfitting, thereby producing a robust model that can generalize to unseen data. We also incorporated a comprehensive risk management framework. Backtesting against historical data was crucial to assess the model's predictive accuracy. This analysis considers the potential impact of various factors and assesses the model's ability to handle unpredictable events. This model aims to provide a more accurate forecast compared to traditional methods, accounting for the intricate interplay of market forces and company-specific data. Key metrics considered for model evaluation include Mean Absolute Error (MAE) and Root Mean Squared Error (RMSE). These metrics measure the difference between predicted and actual values. By minimizing these errors, the model achieves an optimized forecasting capability.
The output of this model is a probabilistic forecast, providing a range of possible future values for LX's stock price. This probabilistic nature reflects the inherent uncertainty in financial markets and provides investors with a more informed decision-making process. The final model is deployed on a platform capable of continuous monitoring and updating based on new data. The model incorporates a system to automatically incorporate new data into the forecasting process, allowing for continuous refinement. Regular monitoring and retraining of the model are crucial to maintain its accuracy and prevent it from becoming obsolete. This proactive approach to model maintenance ensures that the model remains relevant and reliable in the dynamic stock market environment, delivering timely and valuable insights for potential investors. This refined, up-to-date model is intended to offer a robust outlook on LX's market position and prospects over time. The model's outputs will help inform investment strategies and risk assessments.
ML Model Testing
n:Time series to forecast
p:Price signals of LexinFintech Holdings stock
j:Nash equilibria (Neural Network)
k:Dominated move of LexinFintech Holdings stock holders
a:Best response for LexinFintech Holdings target price
For further technical information as per how our model work we invite you to visit the article below:
How do KappaSignal algorithms actually work?
LexinFintech Holdings Stock Forecast (Buy or Sell) Strategic Interaction Table
Strategic Interaction Table Legend:
X axis: *Likelihood% (The higher the percentage value, the more likely the event will occur.)
Y axis: *Potential Impact% (The higher the percentage value, the more likely the price will deviate.)
Z axis (Grey to Black): *Technical Analysis%
LexinFintech Holdings Ltd. (Lexin) Financial Outlook and Forecast
Lexin's financial outlook hinges significantly on the continued performance of its core business segments, particularly its lending operations. A key factor influencing the financial forecast is the evolving regulatory environment surrounding fintech companies in its primary markets. Stringent regulations may impact the company's ability to expand its lending activities and maintain profitability. Growth in the fintech sector, especially in its key regions, will directly correlate with the overall potential for Lexin's expansion. The company's ability to adapt to changing market dynamics, including the rise of alternative lending platforms and digital payment solutions, will be critical to long-term success. Maintaining a strong risk management framework to mitigate potential loan defaults is crucial to ensuring the sustainability of its profitability.
The company's efficiency in managing costs and operations will also play a vital role in its financial performance. Control over operational expenditures is essential for generating healthy profits. The successful implementation of cost-saving measures and strategies is a critical aspect of ensuring a positive financial forecast. Further, effective use of technology to streamline operations and enhance customer service will be essential. The ability to harness technology effectively to meet the ever-changing demands of a digitally-driven world will be a critical success factor. The efficacy of their product development will be directly related to the overall growth potential. Successful product development will allow the company to expand its customer base, while also maintaining a competitive edge in the market.
The broader economic climate, particularly in the regions where Lexin operates, will exert a significant influence on the company's financial performance. Economic downturns can negatively impact consumer spending and borrowing activity, directly affecting the company's loan portfolio and profitability. However, positive economic growth in those markets can fuel loan demand and boost the overall revenue stream. The company's risk management strategies, such as credit scoring models and diversification of lending portfolios, will be crucial in mitigating the impact of macroeconomic headwinds. An astute understanding of local market conditions, combined with adaptable business strategies, will be essential to navigate economic uncertainty and maintain financial stability.
Predicting the future is inherently uncertain, but given the factors outlined, a cautiously optimistic outlook for Lexin is warranted. While the company faces regulatory pressures and macroeconomic volatility, its potential for growth remains significant, particularly if it can effectively manage costs, adapt to market changes, and maintain a robust risk management framework. Risk to this prediction lies in the possibility of a sharp downturn in the target markets, leading to a significant increase in loan defaults. Further, the ability to attract and retain skilled personnel in a competitive job market will be critical to successful execution of their growth plans. Failure to adapt to changes in regulatory frameworks could also pose a substantial risk to their continued expansion. Furthermore, maintaining investor confidence in a volatile market will be critical in obtaining additional capital to support expansion plans.
Rating | Short-Term | Long-Term Senior |
---|---|---|
Outlook | B2 | Ba3 |
Income Statement | Caa2 | Baa2 |
Balance Sheet | B3 | B1 |
Leverage Ratios | B2 | Baa2 |
Cash Flow | B2 | C |
Rates of Return and Profitability | Caa2 | B2 |
*Financial analysis is the process of evaluating a company's financial performance and position by neural network. It involves reviewing the company's financial statements, including the balance sheet, income statement, and cash flow statement, as well as other financial reports and documents.
How does neural network examine financial reports and understand financial state of the company?
References
- Wu X, Kumar V, Quinlan JR, Ghosh J, Yang Q, et al. 2008. Top 10 algorithms in data mining. Knowl. Inform. Syst. 14:1–37
- J. N. Foerster, Y. M. Assael, N. de Freitas, and S. Whiteson. Learning to communicate with deep multi-agent reinforcement learning. In Advances in Neural Information Processing Systems 29: Annual Conference on Neural Information Processing Systems 2016, December 5-10, 2016, Barcelona, Spain, pages 2137–2145, 2016.
- Scholkopf B, Smola AJ. 2001. Learning with Kernels: Support Vector Machines, Regularization, Optimization, and Beyond. Cambridge, MA: MIT Press
- K. Tumer and D. Wolpert. A survey of collectives. In K. Tumer and D. Wolpert, editors, Collectives and the Design of Complex Systems, pages 1–42. Springer, 2004.
- V. Mnih, K. Kavukcuoglu, D. Silver, A. Rusu, J. Veness, M. Bellemare, A. Graves, M. Riedmiller, A. Fidjeland, G. Ostrovski, S. Petersen, C. Beattie, A. Sadik, I. Antonoglou, H. King, D. Kumaran, D. Wierstra, S. Legg, and D. Hassabis. Human-level control through deep reinforcement learning. Nature, 518(7540):529–533, 02 2015.
- Athey S, Wager S. 2017. Efficient policy learning. arXiv:1702.02896 [math.ST]
- R. Rockafellar and S. Uryasev. Optimization of conditional value-at-risk. Journal of Risk, 2:21–42, 2000.