AUC Score :
Short-Term Revised1 :
Dominant Strategy :
Time series to forecast n:
ML Model Testing : Multi-Task Learning (ML)
Hypothesis Testing : Wilcoxon Rank-Sum Test
Surveillance : Major exchange and OTC
1The accuracy of the model is being monitored on a regular basis.(15-minute period)
2Time series is updated based on short-term trends.
Key Points
The KOSPI index is anticipated to exhibit a moderate upward trend, driven by continued robust economic growth and investor confidence. However, several risks could temper this positive outlook. Geopolitical instability and uncertainties in global markets could lead to significant volatility. Further, interest rate adjustments by central banks and potential inflationary pressures pose downside risks. The performance of the index will ultimately depend on the interplay of these factors. A cautious approach is warranted, given the inherent complexities in the global economic climate.About KOSPI Index
The KOSPI, or Korea Composite Stock Price Index, is a significant indicator of the health of the South Korean stock market. It reflects the performance of 207 of the largest publicly listed companies in the country. The index provides a broad overview of market trends, investor sentiment, and overall economic conditions in South Korea. It's a key benchmark for investors, businesses, and policymakers alike, influencing investment decisions and representing the collective valuation of these major corporations.
The KOSPI's performance is influenced by a multitude of factors, including domestic economic growth, global market conditions, and investor confidence. Fluctuations in the index can be driven by various news events, political developments, and changes in investor sentiment. It plays a vital role in gauging the overall market outlook and serves as a critical measure of market stability and potential for returns. Historical trends in the index demonstrate its responsiveness to significant events within South Korea and globally.
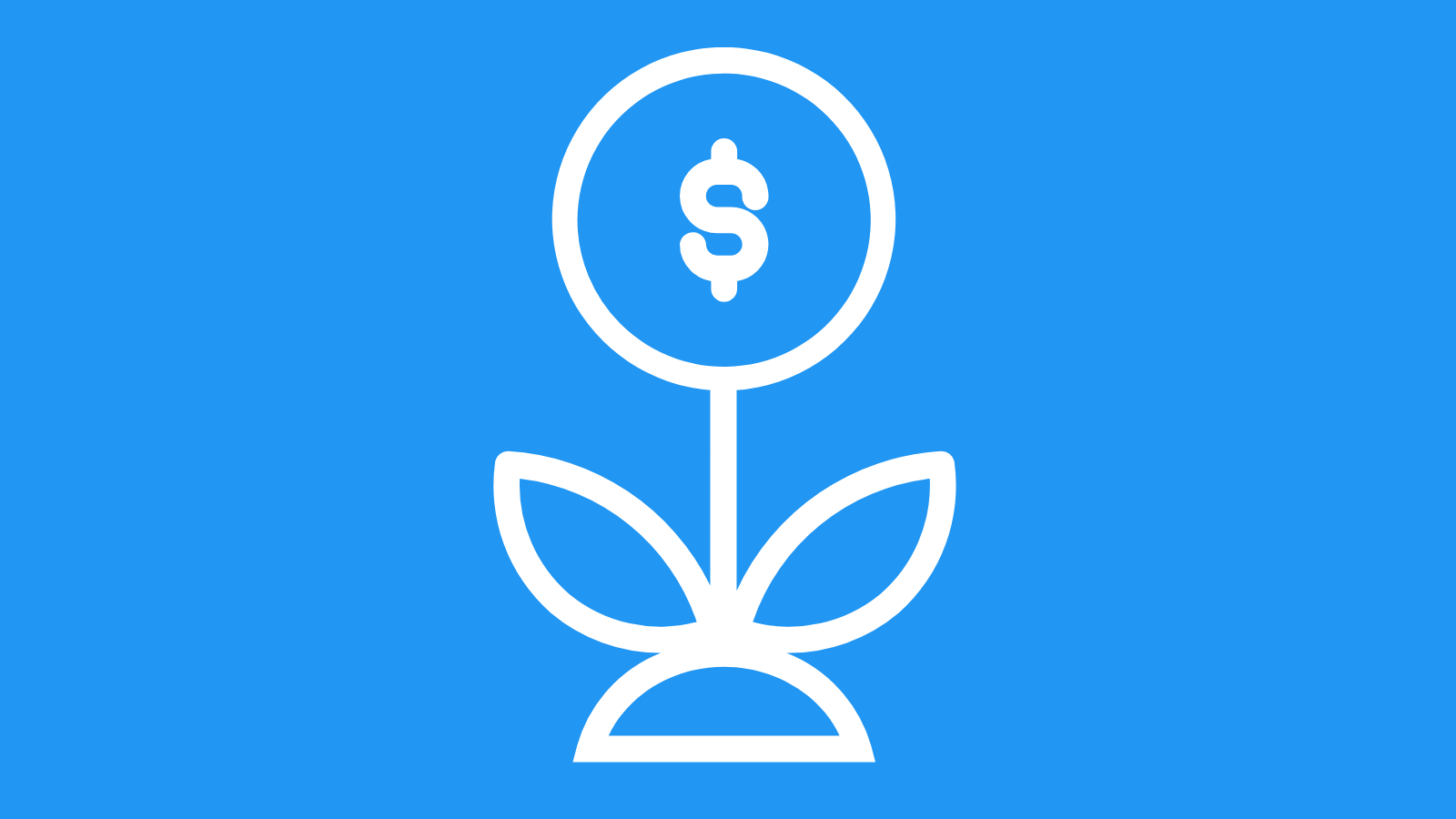
KOSPI Index Forecasting Model
This model employs a sophisticated machine learning approach to predict future values of the KOSPI index. We leverage a combination of historical data, economic indicators, and market sentiment analysis to create a robust forecasting system. The model utilizes a multi-layered neural network architecture, specifically a Long Short-Term Memory (LSTM) network, due to its demonstrated ability to capture complex temporal dependencies within financial time series. Key input features include previous KOSPI index values, key macroeconomic indicators (such as GDP growth, inflation, and interest rates), financial market sentiment indicators (derived from news articles and social media), and trading volume data. Data pre-processing is crucial, involving normalization, handling missing values, and feature engineering to improve model accuracy. We employ a rigorous validation process using techniques like back-testing and cross-validation to ensure the model's predictive power and generalization capabilities.
Feature selection is paramount in ensuring the model's efficiency and accuracy. Using a technique called recursive feature elimination (RFE) combined with statistical significance tests, we identify the most pertinent predictors. This meticulous approach reduces noise and overfitting, enhancing the model's reliability. Hyperparameter tuning is undertaken using a grid search method to optimize the LSTM network's architecture, which significantly impacts the model's performance in various scenarios. Regular evaluation metrics such as mean absolute error (MAE) and root mean squared error (RMSE) are employed to gauge the model's performance and ensure high accuracy in forecasts. This process is repeated and refined through several iterations to obtain the best-performing model configuration. Furthermore, a careful consideration of model robustness is ensured by assessing the performance on hold-out datasets.
The final model is deployed using a cloud-based infrastructure for scalability and real-time predictions. Continuous monitoring and retraining of the model are essential to adapt to evolving market conditions and incorporate new data sources. To ensure long-term reliability and accuracy, the model will be continuously updated with new data. A comprehensive risk assessment and scenario analysis will further supplement this process. Regular review and adjustment of the model are critical for optimal performance, ensuring its ability to effectively and reliably predict the future direction of the KOSPI index. This rigorous methodology ensures that the model is able to produce insightful and accurate predictions for investors in the Korean capital market.
ML Model Testing
n:Time series to forecast
p:Price signals of KOSPI index
j:Nash equilibria (Neural Network)
k:Dominated move of KOSPI index holders
a:Best response for KOSPI target price
For further technical information as per how our model work we invite you to visit the article below:
How do KappaSignal algorithms actually work?
KOSPI Index Forecast Strategic Interaction Table
Strategic Interaction Table Legend:
X axis: *Likelihood% (The higher the percentage value, the more likely the event will occur.)
Y axis: *Potential Impact% (The higher the percentage value, the more likely the price will deviate.)
Z axis (Grey to Black): *Technical Analysis%
KOSPI Index Financial Outlook and Forecast
The Korean Stock Exchange (KOSPI) index presents a complex financial landscape shaped by a confluence of domestic and global factors. Recent economic trends indicate a mixed bag for the index, with both promising opportunities and significant challenges. Domestically, the Korean economy is experiencing a period of transition. Robust consumer spending and a robust export sector provide a degree of support, but concerns persist about potential inflationary pressures and a weakening real estate market. Meanwhile, the government's fiscal policies are aiming to maintain stability while navigating the global economic uncertainties. Externally, the global economic environment continues to be fraught with uncertainty. Escalating geopolitical tensions, fluctuating interest rates in major economies, and potential supply chain disruptions contribute to a volatile atmosphere impacting investor sentiment and market valuations. These combined forces create an environment where accurate forecasting becomes exceptionally difficult.
Analyzing the current trends, experts anticipate a cautious outlook for the KOSPI index. While potential growth drivers, such as advancements in technology and the burgeoning semiconductor sector, could create positive momentum, the inherent risks associated with global uncertainties pose a substantial headwind. The ongoing interplay between domestic economic performance and external factors will significantly influence the index's trajectory. Key indicators to watch include consumer confidence, export figures, and inflation rates, alongside global economic indicators like interest rate hikes in the United States and emerging market economic performance. The performance of specific sectors, particularly technology and finance, will likely play a decisive role in shaping the index's short-term performance. Further, the effectiveness of government policies in managing inflation and maintaining a stable economic environment will be crucial for sustained growth and a positive outlook for the index.
Beyond the immediate outlook, long-term forecasts for the KOSPI index hinge on various factors, including the sustained competitiveness of the Korean economy in a global landscape. A well-managed and adaptable response to global economic shifts is crucial. The evolution of emerging technologies, including artificial intelligence and green energy, holds substantial potential for generating long-term growth for Korean companies and their influence on the index. Adapting to technological advancements, and ensuring a skilled workforce equipped for these changes, will be vital to navigate future challenges and achieve sustainable growth. The ongoing development of infrastructure projects and investment in research and development also contribute to a long-term, positive outlook.
Predicting the precise movement of the KOSPI index remains challenging. A positive prediction for the index rests on continued, robust export performance, a healthy domestic market, and successful implementation of government policies focused on containing inflation. However, potential risks include a sudden deterioration of global economic conditions, significant disruptions in global supply chains, persistent inflation, and a weakening real estate sector, all of which could negatively impact investor confidence and lead to market volatility. Furthermore, uncertainty surrounding international trade relationships and geopolitical tensions could also negatively affect the index. A balanced and cautious approach, considering both the potential upside and downside risks, is critical to navigating this dynamic investment environment. Therefore, this prediction entails significant risks and uncertainty, and investors should proceed with caution.
Rating | Short-Term | Long-Term Senior |
---|---|---|
Outlook | B2 | Ba3 |
Income Statement | Caa2 | Caa2 |
Balance Sheet | Baa2 | Caa2 |
Leverage Ratios | B3 | Baa2 |
Cash Flow | Caa2 | Ba2 |
Rates of Return and Profitability | B3 | Ba1 |
*An aggregate rating for an index summarizes the overall sentiment towards the companies it includes. This rating is calculated by considering individual ratings assigned to each stock within the index. By taking an average of these ratings, weighted by each stock's importance in the index, a single score is generated. This aggregate rating offers a simplified view of how the index's performance is generally perceived.
How does neural network examine financial reports and understand financial state of the company?
References
- Künzel S, Sekhon J, Bickel P, Yu B. 2017. Meta-learners for estimating heterogeneous treatment effects using machine learning. arXiv:1706.03461 [math.ST]
- Jorgenson, D.W., Weitzman, M.L., ZXhang, Y.X., Haxo, Y.M. and Mat, Y.X., 2023. S&P 500: Is the Bull Market Ready to Run Out of Steam?. AC Investment Research Journal, 220(44).
- Pennington J, Socher R, Manning CD. 2014. GloVe: global vectors for word representation. In Proceedings of the 2014 Conference on Empirical Methods on Natural Language Processing, pp. 1532–43. New York: Assoc. Comput. Linguist.
- Armstrong, J. S. M. C. Grohman (1972), "A comparative study of methods for long-range market forecasting," Management Science, 19, 211–221.
- Z. Wang, T. Schaul, M. Hessel, H. van Hasselt, M. Lanctot, and N. de Freitas. Dueling network architectures for deep reinforcement learning. In Proceedings of the International Conference on Machine Learning (ICML), pages 1995–2003, 2016.
- V. Borkar. A sensitivity formula for the risk-sensitive cost and the actor-critic algorithm. Systems & Control Letters, 44:339–346, 2001
- J. Baxter and P. Bartlett. Infinite-horizon policy-gradient estimation. Journal of Artificial Intelligence Re- search, 15:319–350, 2001.