AUC Score :
Short-Term Revised1 :
Dominant Strategy :
Time series to forecast n:
ML Model Testing : Reinforcement Machine Learning (ML)
Hypothesis Testing : Wilcoxon Rank-Sum Test
Surveillance : Major exchange and OTC
1The accuracy of the model is being monitored on a regular basis.(15-minute period)
2Time series is updated based on short-term trends.
Key Points
This exclusive content is only available to premium users.About Dow Jones U.S. Select Home Construction Index
The Dow Jones U.S. Select Home Construction Index is a market-capitalization-weighted index designed to track the performance of companies primarily engaged in residential home construction in the United States. It provides a snapshot of the overall health and direction of the sector, reflecting factors such as building material costs, housing demand, and regulatory environments. The index aims to capture the performance of the largest and most significant publicly traded companies within this sector, although the exact companies included can and do change over time.
The index's constituents are closely monitored for their financial performance and market presence. This allows investors to gain insights into trends influencing home construction and associated industries, like lumber and other building materials. Fluctuations in the index's value are considered an indicator of the broader economic climate, as residential construction is often highly sensitive to economic cycles and market sentiment. Changes in this index, therefore, can offer valuable insights into the current and projected future health of the U.S. residential construction market.
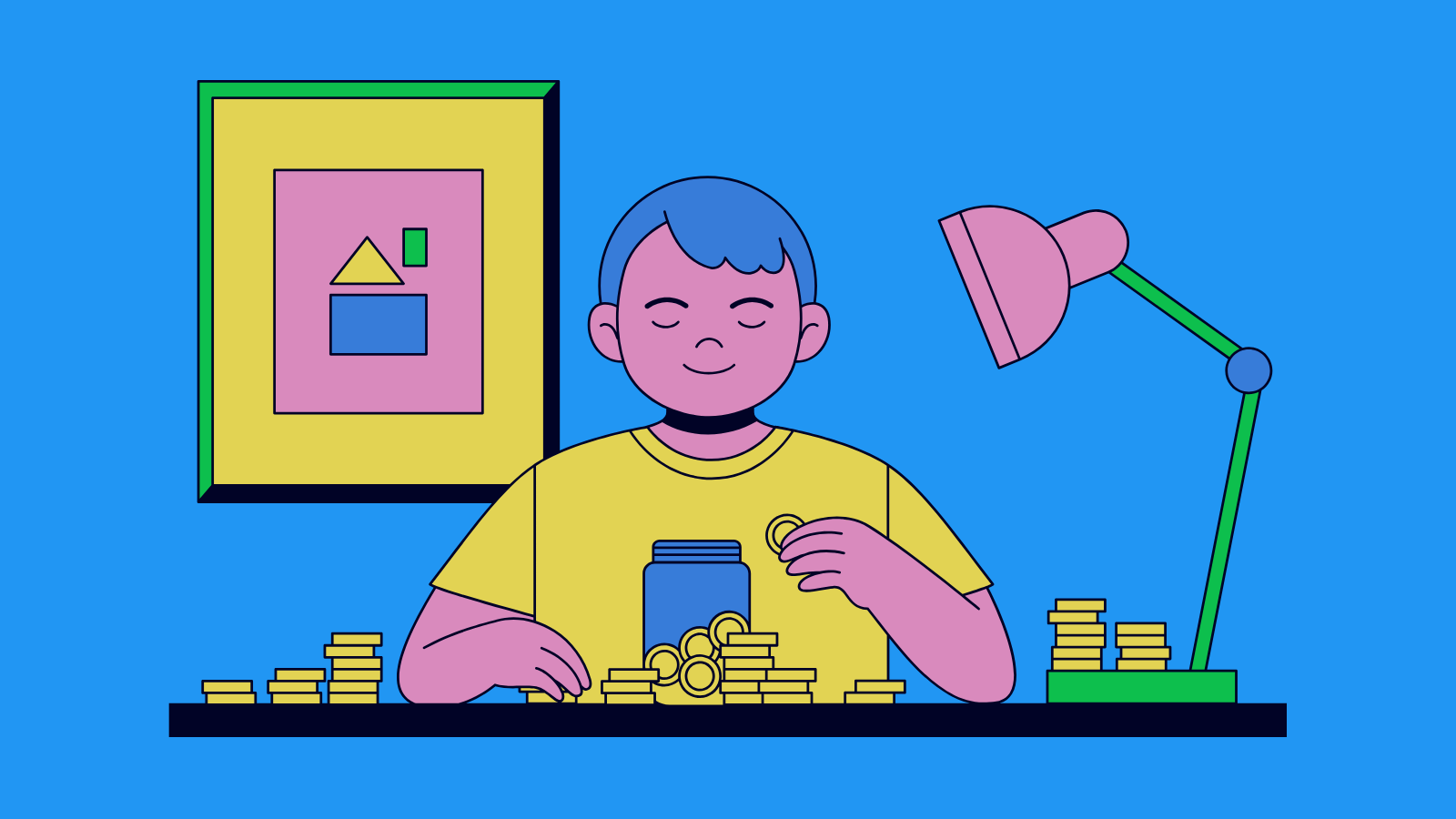
Dow Jones U.S. Select Home Construction Index Forecasting Model
This model utilizes a combination of machine learning algorithms and economic indicators to predict future movements in the Dow Jones U.S. Select Home Construction index. The model's core components include a comprehensive dataset encompassing historical index data, alongside relevant economic factors such as interest rates, housing starts, building permits, and consumer confidence. Feature engineering plays a crucial role, transforming these raw data points into meaningful predictors. This involves creating derived variables like moving averages, ratios, and lagged values to capture underlying trends and seasonality. The dataset is rigorously pre-processed to handle missing values, outliers, and potential data leakage. A crucial aspect of this model is the careful selection of appropriate machine learning algorithms, including regression models (e.g., linear, support vector regression) and time series models (e.g., ARIMA, LSTM). These models are trained on the processed data to establish a predictive relationship between the features and the target variable – future index values. Performance is rigorously assessed using appropriate metrics like Mean Squared Error (MSE), Root Mean Squared Error (RMSE), and R-squared to ensure model reliability. Cross-validation techniques are employed to validate the model's ability to generalize to unseen data.
The model's architecture allows for dynamic updates as new data becomes available. A sliding window approach is used for incremental learning. Regular model retraining is scheduled to incorporate recent economic data, ensuring the model continuously adapts to changing market conditions. This proactive approach helps maintain the accuracy and predictive power of the model over time. Furthermore, sensitivity analysis is employed to understand the impact of different economic variables on the index's projected movement. This aids in identifying key drivers of the construction market and enhances the comprehensiveness of the model's insights. The model is designed to provide not only a point forecast but also a confidence interval, reflecting the model's uncertainty and allowing for more informed decision-making.
The model's success hinges on the continuous monitoring and evaluation of its performance. Regular performance reviews will be conducted to identify potential biases, model degradation, or areas requiring improvement. Furthermore, the inclusion of external economic data sources, like government reports and market analysis, can provide additional perspectives and improve the model's predictive capabilities. Finally, the model's outputs are presented in a user-friendly format, making the forecasts accessible and understandable to analysts, investors, and stakeholders. Visualizations and clear explanations accompany the predictions to aid in interpretation and decision-making. This ongoing iterative process will enable the model's continual refinement and improvement.
ML Model Testing
n:Time series to forecast
p:Price signals of Dow Jones U.S. Select Home Construction index
j:Nash equilibria (Neural Network)
k:Dominated move of Dow Jones U.S. Select Home Construction index holders
a:Best response for Dow Jones U.S. Select Home Construction target price
For further technical information as per how our model work we invite you to visit the article below:
How do KappaSignal algorithms actually work?
Dow Jones U.S. Select Home Construction Index Forecast Strategic Interaction Table
Strategic Interaction Table Legend:
X axis: *Likelihood% (The higher the percentage value, the more likely the event will occur.)
Y axis: *Potential Impact% (The higher the percentage value, the more likely the price will deviate.)
Z axis (Grey to Black): *Technical Analysis%
Dow Jones U.S. Select Home Construction Index Financial Outlook and Forecast
The Dow Jones U.S. Select Home Construction Index reflects the performance of publicly traded companies primarily engaged in residential construction activities. Analyzing its financial outlook requires a comprehensive understanding of the underlying economic forces impacting the housing sector. Current economic conditions, including interest rates, inflation, and overall consumer confidence, play a crucial role. A robust housing market typically corresponds with positive performance in the index, fueled by demand for new homes and related construction services. Conversely, a downturn in the sector often translates to a decline in the index. Factors like government policies regarding housing incentives and regulations also significantly influence construction activity. Recent trends in housing starts, permits issued, and home sales figures provide crucial insights for forecasting future performance of the index.
Several key macroeconomic variables are integral to assessing the index's prospective trajectory. Interest rate fluctuations, especially mortgage rates, exert a considerable influence on both the demand for new homes and the profitability of construction companies. Higher rates typically curb borrowing and, consequently, reduce demand, impacting the index negatively. Inflationary pressures, impacting material costs, labor costs, and consumer spending, also need careful consideration. Sustained inflation can erode purchasing power, potentially discouraging new home purchases and dampening the construction sector's growth. Consumer confidence in the broader economy significantly influences housing demand. Increased uncertainty and pessimism can lead to a decline in the housing market and a corresponding downward trend in the index. Analyzing historical correlation between these macroeconomic factors and the index's performance can help develop informed predictions for future trends.
Several factors may contribute to a positive or negative outlook for the Dow Jones U.S. Select Home Construction Index. A sustained period of low interest rates, favorable government policies, and improving consumer confidence could drive increased demand for new homes. This positive economic climate can boost the profitability of construction companies, contributing to a rise in the index. Conversely, a prolonged economic downturn, rising interest rates, increasing material costs, or regulatory hurdles could lead to decreased demand and decreased profitability for construction companies, potentially leading to negative index performance. The availability of skilled labor and the overall supply chain efficiency will be critical factors in determining if construction companies can meet demand and profitability expectations.
While predicting the future is inherently uncertain, a positive outlook for the index might be supported by a combination of factors such as a stable or moderately improving economic climate, sustained low interest rates (although caution is needed given the recent trend toward increasing rates), and favorable government policies that support the housing sector. However, this positive forecast is conditional on several key risks. Potential risks include unexpected increases in interest rates, sharp rises in inflation, significant consumer spending declines, or increased regulatory hurdles. Unexpected events like natural disasters or supply chain disruptions could further complicate the situation and significantly impair the outlook for the construction sector. Thus, while a favorable outlook is possible, the current environment suggests that considerable caution is warranted when considering investments in the Dow Jones U.S. Select Home Construction Index. The analysis suggests that any prediction must be considered in light of these dynamic risks and uncertainties. The index's performance will likely fluctuate depending on the evolution of these macroeconomic conditions.
Rating | Short-Term | Long-Term Senior |
---|---|---|
Outlook | Ba2 | Ba2 |
Income Statement | Baa2 | Baa2 |
Balance Sheet | B3 | Ba2 |
Leverage Ratios | Ba2 | B3 |
Cash Flow | Ba2 | B3 |
Rates of Return and Profitability | B1 | Baa2 |
*An aggregate rating for an index summarizes the overall sentiment towards the companies it includes. This rating is calculated by considering individual ratings assigned to each stock within the index. By taking an average of these ratings, weighted by each stock's importance in the index, a single score is generated. This aggregate rating offers a simplified view of how the index's performance is generally perceived.
How does neural network examine financial reports and understand financial state of the company?
References
- Alexander, J. C. Jr. (1995), "Refining the degree of earnings surprise: A comparison of statistical and analysts' forecasts," Financial Review, 30, 469–506.
- Thomas P, Brunskill E. 2016. Data-efficient off-policy policy evaluation for reinforcement learning. In Pro- ceedings of the International Conference on Machine Learning, pp. 2139–48. La Jolla, CA: Int. Mach. Learn. Soc.
- Li L, Chen S, Kleban J, Gupta A. 2014. Counterfactual estimation and optimization of click metrics for search engines: a case study. In Proceedings of the 24th International Conference on the World Wide Web, pp. 929–34. New York: ACM
- E. Collins. Using Markov decision processes to optimize a nonlinear functional of the final distribution, with manufacturing applications. In Stochastic Modelling in Innovative Manufacturing, pages 30–45. Springer, 1997
- M. Puterman. Markov Decision Processes: Discrete Stochastic Dynamic Programming. Wiley, New York, 1994.
- Mikolov T, Yih W, Zweig G. 2013c. Linguistic regularities in continuous space word representations. In Pro- ceedings of the 2013 Conference of the North American Chapter of the Association for Computational Linguistics: Human Language Technologies, pp. 746–51. New York: Assoc. Comput. Linguist.
- D. White. Mean, variance, and probabilistic criteria in finite Markov decision processes: A review. Journal of Optimization Theory and Applications, 56(1):1–29, 1988.