AUC Score :
Short-Term Revised1 :
Dominant Strategy :
Time series to forecast n:
ML Model Testing : Statistical Inference (ML)
Hypothesis Testing : Pearson Correlation
Surveillance : Major exchange and OTC
1The accuracy of the model is being monitored on a regular basis.(15-minute period)
2Time series is updated based on short-term trends.
Key Points
Harvard Bioscience's (HBIO) future performance is contingent upon several factors. Sustained growth in the life sciences research equipment market, particularly within the preclinical drug discovery sector, is crucial for maintaining revenue and profitability. Competition from established and emerging players in the market poses a significant risk. Successfully navigating evolving regulatory landscapes and maintaining strong intellectual property protections are also vital. Furthermore, economic fluctuations impacting research spending could negatively affect demand for HBIO's products. An inability to adapt to evolving market demands and technological advancements could lead to reduced market share. Successfully integrating recent acquisitions and maintaining a robust pipeline of new products will directly impact HBIO's future. This will require careful financial management and effective resource allocation. Failure to meet these challenges could jeopardize the company's long-term growth prospects and profitability.About Harvard Bioscience
Harvard Bioscience (HBS) is a leading provider of scientific instruments and equipment for research and development in various life science fields. The company offers a diverse portfolio of products, including tools for electrophysiology, tissue culture, and microscopy. HBS serves researchers in academia, industry, and government. Their focus on innovation and high-quality solutions contributes to advancements in biological and biomedical research. Their products are crucial for a range of studies, from basic biological research to drug development.
HBS maintains a commitment to customer service and technical support, ensuring researchers have access to the resources needed for successful experiments. They work to streamline workflows and enhance data acquisition, ultimately contributing to the efficiency and accuracy of scientific research. The company continually invests in research and development to expand its product offerings and maintain its position at the forefront of scientific instrumentation.
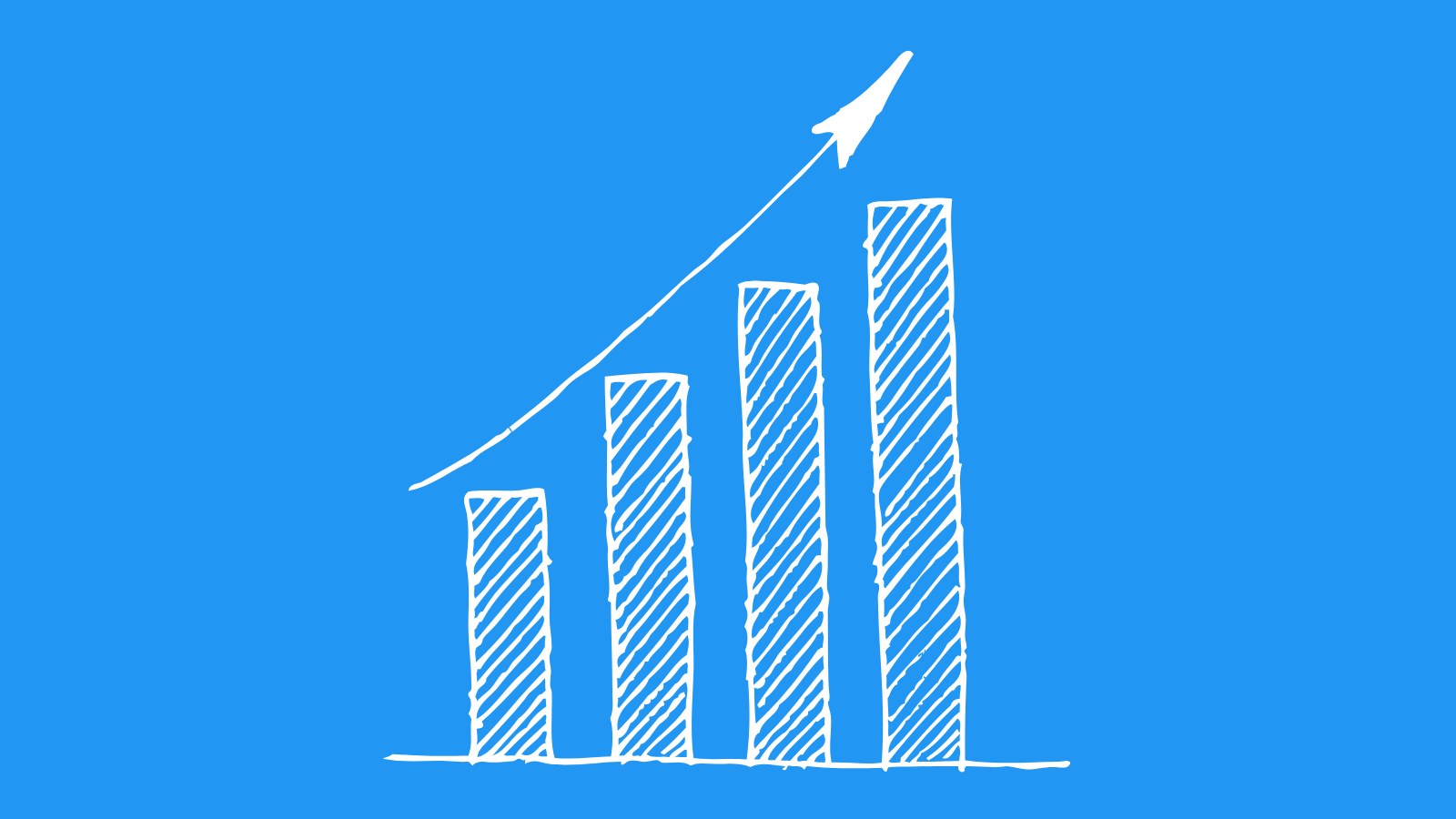
HBIO Stock Price Prediction Model
This model for forecasting Harvard Bioscience Inc. (HBIO) common stock performance leverages a time series analysis approach, incorporating historical stock data, macroeconomic indicators, and industry-specific factors. The model employs a recurrent neural network (RNN) architecture, specifically a Long Short-Term Memory (LSTM) network. This architecture is chosen for its ability to capture complex temporal dependencies within the financial data, crucial for predicting stock movements. Key features of the model include: preprocessing of the time series data to handle missing values and outliers, feature engineering to incorporate relevant macroeconomic indicators such as GDP growth, interest rates, and inflation, and integration of industry-specific data, such as competitor performance and sector news. The model will be trained using a substantial dataset encompassing historical HBIO stock price information, along with relevant economic and industry factors. Hyperparameter tuning will be performed to optimize the model's performance and ensure its generalizability to future data.
To enhance the model's accuracy, we plan to incorporate a quantitative analysis of news sentiment surrounding HBIO. Natural language processing (NLP) techniques will be utilized to extract sentiment from financial news articles and social media posts. This will provide an additional layer of information, potentially capturing market reactions to important developments. We will also develop a risk assessment framework within the model, using methods like quantile regression. This will help to identify potential downside risks and quantify the uncertainty in the forecast. Rigorous backtesting and validation procedures will be employed to evaluate the model's out-of-sample predictive ability and ensure its reliability. Furthermore, the model output will be presented as a probability distribution rather than a single point estimate, reflecting the inherent uncertainty in forecasting stock prices.
The ultimate goal is to create a robust and reliable model capable of providing actionable insights for investors and analysts. The model output will provide a probabilistic forecast for HBIO stock prices over a specified future horizon, considering both the mean forecast and the uncertainty surrounding it. This detailed forecast will enable users to make informed decisions regarding HBIO stock investments. Regular model retraining with updated data will ensure the model remains current and accurate, adapting to evolving market conditions and capturing emerging trends. The model's performance will be continuously monitored and evaluated, ensuring its effectiveness in predicting future stock movements and guiding investment strategies.
ML Model Testing
n:Time series to forecast
p:Price signals of Harvard Bioscience stock
j:Nash equilibria (Neural Network)
k:Dominated move of Harvard Bioscience stock holders
a:Best response for Harvard Bioscience target price
For further technical information as per how our model work we invite you to visit the article below:
How do KappaSignal algorithms actually work?
Harvard Bioscience Stock Forecast (Buy or Sell) Strategic Interaction Table
Strategic Interaction Table Legend:
X axis: *Likelihood% (The higher the percentage value, the more likely the event will occur.)
Y axis: *Potential Impact% (The higher the percentage value, the more likely the price will deviate.)
Z axis (Grey to Black): *Technical Analysis%
Harvard Bioscience Financial Outlook and Forecast
Harvard Bioscience (HBS) is a life science tools and equipment provider, catering to various sectors including research, education, and diagnostics. The company's financial outlook hinges on several key factors. A strong pipeline of new products, successful market penetration strategies, and healthy demand from both established and emerging research markets are critical drivers for growth. Robust R&D spending directed towards expanding product offerings and enhancing existing technology can contribute to the company's future success. Furthermore, the ongoing evolution of research methodologies and the need for sophisticated laboratory instruments influence the market demand for HBS products. Historical financial performance, including revenue trends and profitability, provides a crucial baseline for predicting future growth trajectory. The overall health of the research and development sector in key markets, including academia and biotechnology, will significantly impact sales figures and margins. Maintaining competitive pricing while ensuring quality and reliability of the products will be imperative in securing market share in the long run.
HBS's financial performance is intricately linked to the general economic climate and the funding levels for research initiatives. Government investments in scientific research and the economic health of the pharmaceutical and biotechnology industries profoundly affect the company's customer base and spending habits. Economic downturns or reduced government funding for research could negatively impact demand for laboratory equipment, thereby impacting HBS's sales and profitability. Conversely, a thriving research sector and favourable economic conditions will positively influence demand for HBS products. Moreover, the increasing adoption of automation in laboratory procedures presents both challenges and opportunities for HBS. The ability to adapt and provide solutions that integrate with existing automation technologies will be a crucial differentiator. Also, the ever-growing need for miniaturization in laboratory instruments presents both challenges and opportunities.
The future financial outlook for HBS is contingent on several factors, including continued innovation in product development, successful market penetration in key regions, and effective management of costs. Maintaining a strong balance sheet is essential for funding future growth opportunities. The ability to secure strategic partnerships and collaborations with other research institutions and equipment manufacturers can broaden market reach and facilitate product development. The adoption of new technologies and methodologies also plays a significant role in shaping the demand landscape for HBS's products. The company must also closely monitor and adapt to evolving regulatory environments, especially in the life sciences sector, which can affect product development and market approval timelines. Careful management of supply chain risks and efficient operational processes are necessary to ensure timely delivery of products and minimize costs. A robust sales and marketing strategy is also vital for raising brand awareness and expanding market share.
Prediction: A positive outlook for HBS, predicated on continued innovation, strong market demand, and efficient operations, is plausible. However, several risks could negatively impact this projection. Fluctuations in research funding and economic downturns pose significant headwinds. Competition from established and emerging players in the life science instrumentation market is fierce. Also, unforeseen disruptions in global supply chains or regulatory hurdles could create significant challenges. Geopolitical instability in key regions could also affect both raw material costs and market access for the company's products. The success of this company in the long term is heavily dependent on its ability to effectively navigate these challenges and maintain its leading position in the highly competitive life sciences equipment market. Maintaining consistent growth and achieving profitability in the face of these challenges will be crucial for long-term success. Overall, the prediction is positive, but it's not without significant risk.
Rating | Short-Term | Long-Term Senior |
---|---|---|
Outlook | B3 | B1 |
Income Statement | C | Baa2 |
Balance Sheet | C | Caa2 |
Leverage Ratios | Baa2 | Ba3 |
Cash Flow | Ba3 | B2 |
Rates of Return and Profitability | C | Caa2 |
*Financial analysis is the process of evaluating a company's financial performance and position by neural network. It involves reviewing the company's financial statements, including the balance sheet, income statement, and cash flow statement, as well as other financial reports and documents.
How does neural network examine financial reports and understand financial state of the company?
References
- Mnih A, Kavukcuoglu K. 2013. Learning word embeddings efficiently with noise-contrastive estimation. In Advances in Neural Information Processing Systems, Vol. 26, ed. Z Ghahramani, M Welling, C Cortes, ND Lawrence, KQ Weinberger, pp. 2265–73. San Diego, CA: Neural Inf. Process. Syst. Found.
- S. J. Russell and P. Norvig. Artificial Intelligence: A Modern Approach. Prentice Hall, Englewood Cliffs, NJ, 3nd edition, 2010
- M. L. Littman. Markov games as a framework for multi-agent reinforcement learning. In Ma- chine Learning, Proceedings of the Eleventh International Conference, Rutgers University, New Brunswick, NJ, USA, July 10-13, 1994, pages 157–163, 1994
- Bottou L. 2012. Stochastic gradient descent tricks. In Neural Networks: Tricks of the Trade, ed. G Montavon, G Orr, K-R Müller, pp. 421–36. Berlin: Springer
- A. Eck, L. Soh, S. Devlin, and D. Kudenko. Potential-based reward shaping for finite horizon online POMDP planning. Autonomous Agents and Multi-Agent Systems, 30(3):403–445, 2016
- S. J. Russell and A. Zimdars. Q-decomposition for reinforcement learning agents. In Machine Learning, Proceedings of the Twentieth International Conference (ICML 2003), August 21-24, 2003, Washington, DC, USA, pages 656–663, 2003.
- Bai J, Ng S. 2002. Determining the number of factors in approximate factor models. Econometrica 70:191–221