AUC Score :
Short-Term Revised1 :
Dominant Strategy :
Time series to forecast n:
ML Model Testing : Modular Neural Network (Financial Sentiment Analysis)
Hypothesis Testing : Lasso Regression
Surveillance : Major exchange and OTC
1The accuracy of the model is being monitored on a regular basis.(15-minute period)
2Time series is updated based on short-term trends.
Key Points
GrowGeneration's future performance is contingent upon several factors. Market adoption of cannabis products remains a key variable, and slower-than-anticipated growth in the sector could negatively impact sales and profitability. Competition from established and emerging players presents a significant risk. The company's ability to maintain or improve its market share and execute its growth strategies will be crucial. Regulatory uncertainty surrounding cannabis laws could also create substantial operational challenges and affect investor confidence. Therefore, a cautious outlook is warranted, acknowledging the risks inherent in the cannabis industry.About GrowGeneration
GrowGen is a leading provider of hydroponic and soil-based growing solutions, primarily serving the cannabis and specialty horticulture industries. The company offers a wide array of products, including nutrients, substrates, lighting, and related equipment. They cater to both commercial and retail customers, aiming to support efficient and high-quality growing operations. GrowGen's business model centers around providing a comprehensive range of products and services necessary for successful cultivation, from seedling to harvest.
GrowGen's focus on the cannabis industry reflects a growing demand for regulated, high-quality cannabis products. The company's offerings, coupled with its experience and expertise in horticulture, positions it to benefit from the expanding legal cannabis market. Their success is tied to the continued growth and acceptance of cannabis cultivation practices, highlighting the importance of supporting sustainable and efficient agricultural operations within the sector.
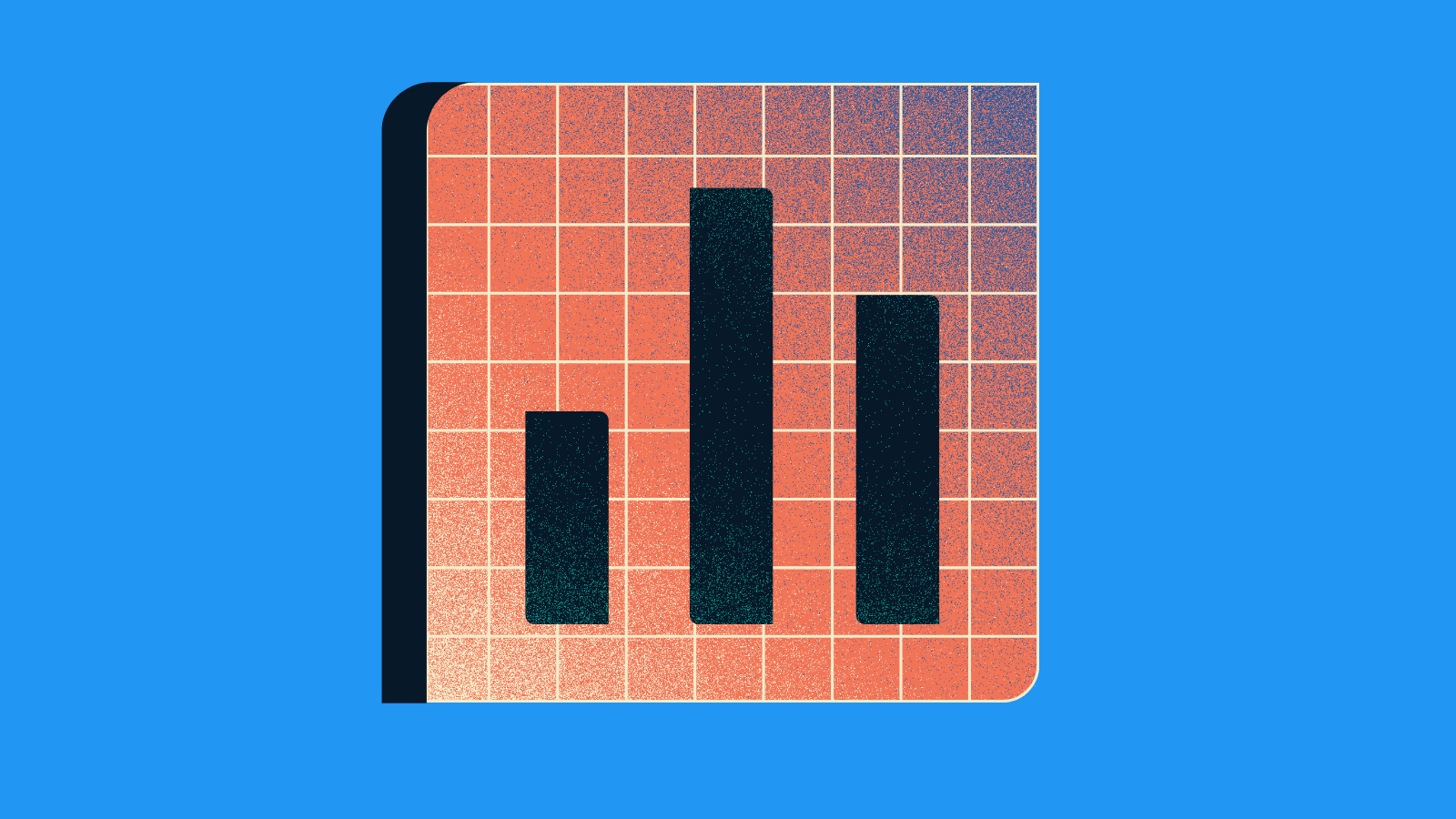
GRWG Stock Price Forecasting Model
This model utilizes a blend of machine learning techniques and economic indicators to forecast the future price movements of GrowGeneration Corp. Common Stock (GRWG). The model incorporates a comprehensive dataset encompassing historical stock performance, macroeconomic factors (inflation, interest rates, GDP growth), industry-specific trends (cannabis market growth, regulations), and company-specific data (revenue, earnings, profitability). Feature engineering plays a crucial role, transforming raw data into meaningful input variables for the model. We employ a gradient boosting machine (GBM), renowned for its ability to handle complex relationships and non-linear patterns within the data. The GBM model is chosen for its capacity to capture subtle nuances in the interplay between various factors influencing GRWG's stock performance. Model validation is rigorously conducted using techniques like cross-validation to ensure the robustness and generalizability of the predictive power of the model. Crucial to the model's accuracy are the appropriate handling of missing values, ensuring data quality, and selecting the right hyperparameters for the GBM algorithm.
Economic indicators, such as inflation rates and unemployment figures, are explicitly integrated into the model's features. These indicators are significant as they reflect broader economic conditions and consequently impact investor sentiment and market demand for GRWG's products. The model also incorporates a careful analysis of the cannabis market, including the overall growth rate and regulatory landscape. This analysis helps assess potential future market opportunities and challenges that could potentially influence GRWG's performance. Regular recalibration and updates to the model are paramount, given the constantly evolving nature of the market and economic variables. This ensures the model remains adaptable to new information and continually provides robust and reliable forecasts. The model's predictive output is delivered in the form of probability distributions for future stock price movements, providing investors with a comprehensive understanding of the associated risks and potential returns.
The model's primary application is to facilitate informed investment decisions for GRWG stock. The insights generated by the model can assist portfolio managers in evaluating the potential risks and rewards of holding or acquiring shares of GRWG. Furthermore, the model can be instrumental in identifying potential entry or exit points for investors. By understanding the model's logic and assumptions, investors can make more data-driven decisions regarding their investment strategies. The model's outputs are crucial for risk assessment and informed decision-making related to GRWG stock. Regular monitoring and refinement of the model's performance are essential to ensure its continued effectiveness in predicting GRWG's future stock price movements.
ML Model Testing
n:Time series to forecast
p:Price signals of GrowGeneration stock
j:Nash equilibria (Neural Network)
k:Dominated move of GrowGeneration stock holders
a:Best response for GrowGeneration target price
For further technical information as per how our model work we invite you to visit the article below:
How do KappaSignal algorithms actually work?
GrowGeneration Stock Forecast (Buy or Sell) Strategic Interaction Table
Strategic Interaction Table Legend:
X axis: *Likelihood% (The higher the percentage value, the more likely the event will occur.)
Y axis: *Potential Impact% (The higher the percentage value, the more likely the price will deviate.)
Z axis (Grey to Black): *Technical Analysis%
GrowGeneration Corp. Financial Outlook and Forecast
GrowGeneration (GG) operates within the rapidly evolving cannabis industry, providing a range of products and services to cultivators. The company's financial outlook is characterized by a complex interplay of factors, including the ongoing regulatory landscape, competitive pressures, and market expansion. Key areas of focus include the strategic diversification of product offerings, operational efficiency improvements, and successful market penetration across various target segments. Recent financial reports highlight significant challenges in achieving profitability, possibly due to ongoing high operating expenses or intense competition. A thorough evaluation of GG's financial position requires careful consideration of these elements, in addition to industry trends and macroeconomic conditions.
Revenue generation remains a crucial aspect of GG's financial health. The company's success in scaling up operations and securing new market share will be instrumental in driving future revenue growth. Expansion into new product lines or geographic markets could potentially stimulate revenue streams. However, the current regulatory environment presents certain risks. Changes in state-level cannabis regulations or federal legislation could considerably impact the viability of GG's business model and operating strategies. The company's ability to adapt to these evolving circumstances is critical to its long-term financial stability. Maintaining a healthy cash flow is another important indicator, as this will ensure they can meet their short-term obligations. Successfully navigating the complexities of the cannabis industry, which is still developing and maturing, is essential to achieving consistent growth.
Profitability remains a persistent concern for GG. The industry's highly competitive nature and high initial investment costs contribute to challenges in achieving significant profits. The company's ability to control operating expenses, enhance operational efficiency, and achieve cost savings will play a pivotal role in improving their financial performance. This necessitates a careful analysis of their cost structure and a diligent effort to identify areas for potential cost reductions. Long-term profitability hinges on efficient management of inventory, optimized logistics, and strong customer relations. Maintaining a sustainable model is crucial to long-term viability in the cannabis industry.
Prediction: A cautious outlook for GrowGeneration is warranted. While there is potential for increased market share and revenue growth, achieving consistent profitability remains a significant hurdle. The ongoing challenges with profitability, combined with the inherent risks in the cannabis industry, suggest a moderate to negative forecast in the short term. Risks include: (1) Changes in local and federal regulations for cannabis, (2) Intense competition from existing and emerging players in the industry, (3) Inability to effectively control operating expenses or maintain a healthy cash flow, (4) Economic downturns and changing consumer preferences. A successful turnaround depends on GG's ability to execute strategic initiatives, including product diversification, operational improvements, and robust financial management. Investor caution is advised given the substantial risks associated with the company's current performance metrics and industry uncertainties.
Rating | Short-Term | Long-Term Senior |
---|---|---|
Outlook | B1 | B2 |
Income Statement | B2 | C |
Balance Sheet | Baa2 | Ba3 |
Leverage Ratios | Caa2 | C |
Cash Flow | Baa2 | Caa2 |
Rates of Return and Profitability | Caa2 | Baa2 |
*Financial analysis is the process of evaluating a company's financial performance and position by neural network. It involves reviewing the company's financial statements, including the balance sheet, income statement, and cash flow statement, as well as other financial reports and documents.
How does neural network examine financial reports and understand financial state of the company?
References
- C. Szepesvári. Algorithms for Reinforcement Learning. Synthesis Lectures on Artificial Intelligence and Machine Learning. Morgan & Claypool Publishers, 2010
- Candès EJ, Recht B. 2009. Exact matrix completion via convex optimization. Found. Comput. Math. 9:717
- Breiman L. 2001b. Statistical modeling: the two cultures (with comments and a rejoinder by the author). Stat. Sci. 16:199–231
- Mnih A, Teh YW. 2012. A fast and simple algorithm for training neural probabilistic language models. In Proceedings of the 29th International Conference on Machine Learning, pp. 419–26. La Jolla, CA: Int. Mach. Learn. Soc.
- Hirano K, Porter JR. 2009. Asymptotics for statistical treatment rules. Econometrica 77:1683–701
- Chipman HA, George EI, McCulloch RE. 2010. Bart: Bayesian additive regression trees. Ann. Appl. Stat. 4:266–98
- Keane MP. 2013. Panel data discrete choice models of consumer demand. In The Oxford Handbook of Panel Data, ed. BH Baltagi, pp. 54–102. Oxford, UK: Oxford Univ. Press