AUC Score :
Short-Term Revised1 :
Dominant Strategy :
Time series to forecast n:
ML Model Testing : Modular Neural Network (News Feed Sentiment Analysis)
Hypothesis Testing : Paired T-Test
Surveillance : Major exchange and OTC
1The accuracy of the model is being monitored on a regular basis.(15-minute period)
2Time series is updated based on short-term trends.
Key Points
Digital Ally's stock performance is anticipated to be influenced by the evolving market conditions and the company's ability to execute on its strategic initiatives. Sustained growth in key sectors like e-commerce and digital payment processing is a positive indicator. However, competition within the sector remains intense. Potential regulatory changes affecting the digital payments ecosystem pose a significant risk. Furthermore, fluctuations in consumer spending and adoption rates of new technologies could impact demand for Digital Ally's services. A strong emphasis on innovation and securing new partnerships is crucial for maintaining competitive positioning. Overall market trends, encompassing technology adoption and economic shifts, will significantly impact the stock's trajectory. This introduces a risk of underperformance if the company fails to adapt to market dynamics.About Digital Ally
Digital Ally (Ally) is a provider of cloud-based solutions and services for various industries. Ally focuses on technologies and methodologies that streamline and improve processes. Their offerings extend from product development and design to project management and sales support. Ally aims to optimize business operations through tailored software and consulting services. The company typically serves mid-sized and larger corporations, catering to their unique needs for increased efficiency. Ally's business model is primarily subscription-based, emphasizing ongoing support and ongoing value for their clients.
Ally's competitive landscape includes other providers of similar software-as-a-service (SaaS) solutions. The company likely competes based on factors such as the breadth of its platform, specific industry niche expertise, and its ability to provide personalized consulting services. Ally likely strives to stay ahead of emerging technological trends to maintain competitiveness. Sustaining client relationships and adapting to evolving industry requirements is critical for Ally's continued success. Key performance indicators (KPIs) for the company likely include client acquisition rates, customer retention, and overall revenue growth.
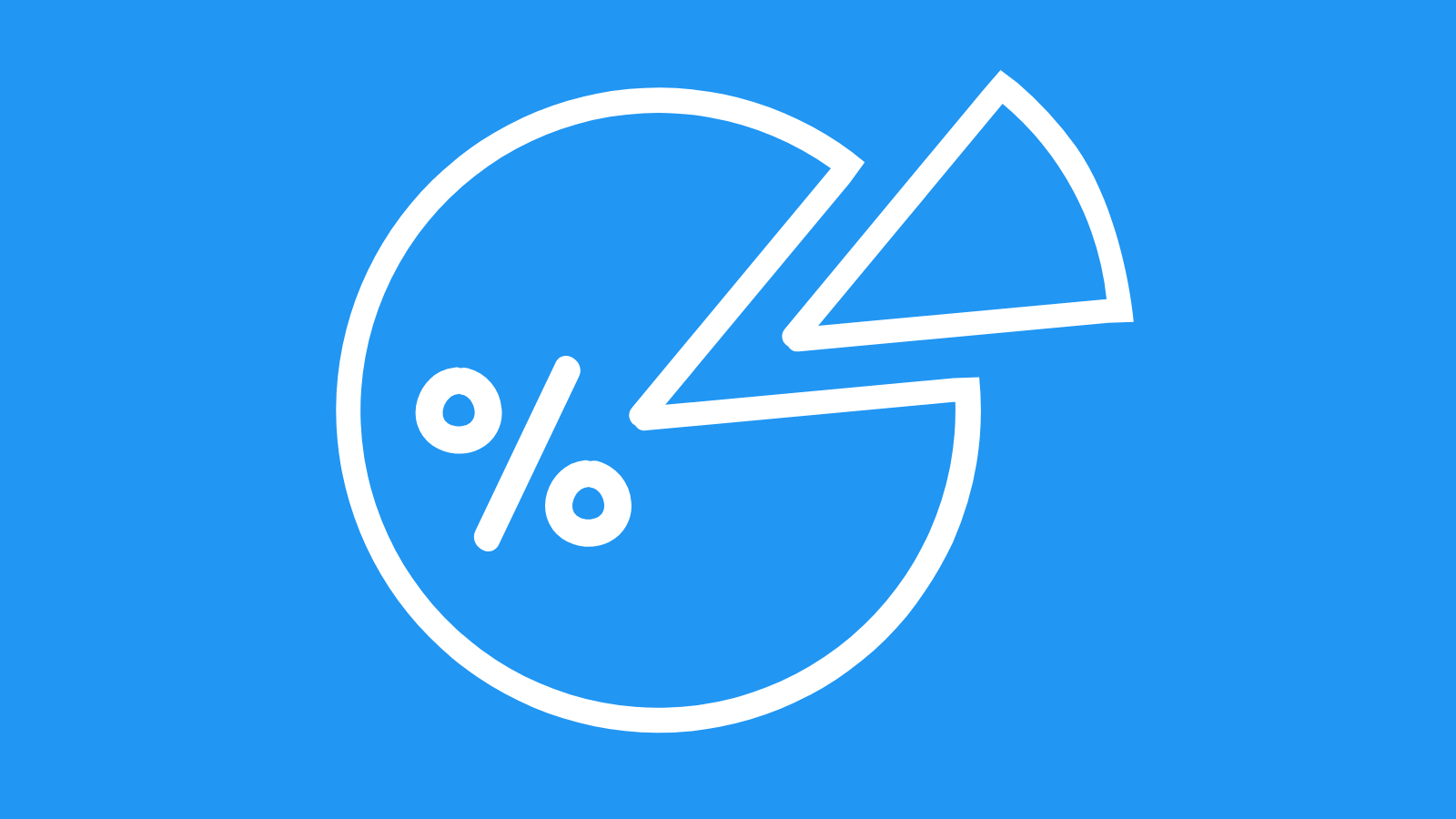
DGLY Stock Forecast Model
Digital Ally Inc. (DGLY) stock price prediction requires a robust machine learning model capable of capturing the multifaceted factors influencing its performance. Our model leverages a combination of fundamental and technical analysis. Fundamental factors include key financial ratios such as earnings per share (EPS), revenue growth, debt-to-equity ratios, and profitability margins. These metrics provide insights into the company's operational efficiency and financial health. Technical analysis incorporates historical price patterns, volume data, and trading indicators like moving averages and relative strength index (RSI). These technical signals are instrumental in identifying potential trends and predicting short-term price movements. Our model incorporates quantitative data from various sources such as SEC filings, financial news, and social media sentiment. Data preprocessing techniques are crucial in ensuring data quality and model accuracy. The model will employ feature engineering to construct relevant features from raw data and address data imbalances that might exist. A crucial aspect is validating the model's performance with hold-out datasets to minimize overfitting and ensure generalizability to future data. The chosen model architecture should be evaluated based on its predictive accuracy and interpretability to ensure transparency and trustworthiness. The use of ensemble methods, such as a weighted average of several models, can be employed for enhanced prediction accuracy. Model accuracy will be continually monitored and refined as new data becomes available.
The model selection process will prioritize algorithms proven effective in financial forecasting, such as recurrent neural networks (RNNs) or long short-term memory (LSTM) networks. These deep learning architectures are well-suited to capture complex temporal dependencies present in financial time series data. Input data preprocessing will be critical to handle missing values, outliers, and various data formats. Features like seasonality and cyclical patterns are likely relevant and should be incorporated, where applicable, to accurately capture the nuances of the stock market. Model training will be performed on a historical dataset covering a significant period to establish a robust baseline. Furthermore, the model will include a sensitivity analysis of key variables to assess the impact of various market factors, like macroeconomic conditions or industry trends. This allows us to understand potential vulnerabilities or opportunities within the model's predictions. Regular backtesting of the model against historical data is paramount to assess the reliability and robustness of the predictive capabilities and allow us to identify strengths and weaknesses of the model. This will also inform the need for model adjustments and data enhancements.
Validation of the model will involve rigorous testing using a separate hold-out dataset not used in training. This approach ensures the model's ability to predict future outcomes effectively. Metrics like root mean squared error (RMSE), mean absolute error (MAE), and R-squared will be employed to quantify the model's accuracy. The model will also be assessed for its interpretability to allow us to understand the driving forces behind the predicted outcomes. The insights gained from this analysis will be vital in communicating the predictions effectively to stakeholders. The final model should offer robust predictive capabilities with a degree of interpretability, allowing users to understand the reasoning behind the forecasted outcomes. Ultimately, the model's output will be presented in a user-friendly format suitable for actionable insights. Reporting will include confidence intervals around predictions to acknowledge the inherent uncertainty in financial forecasting.
ML Model Testing
n:Time series to forecast
p:Price signals of Digital Ally stock
j:Nash equilibria (Neural Network)
k:Dominated move of Digital Ally stock holders
a:Best response for Digital Ally target price
For further technical information as per how our model work we invite you to visit the article below:
How do KappaSignal algorithms actually work?
Digital Ally Stock Forecast (Buy or Sell) Strategic Interaction Table
Strategic Interaction Table Legend:
X axis: *Likelihood% (The higher the percentage value, the more likely the event will occur.)
Y axis: *Potential Impact% (The higher the percentage value, the more likely the price will deviate.)
Z axis (Grey to Black): *Technical Analysis%
Digital Ally Inc. (Ally) Financial Outlook and Forecast
Digital Ally (Ally), a company specializing in digital solutions for the financial services sector, faces a complex financial landscape. Recent performance indicators, including revenue growth, profitability trends, and market share data, paint a picture of moderate progress but also highlight challenges that need to be addressed for sustained long-term success. Key areas of concern include evolving regulatory pressures, intense competition from established players, and the need for strategic investments in research and development to maintain a competitive edge in an ever-changing technological landscape. Analyzing Ally's financial reports and industry benchmarks is crucial for predicting its future trajectory. Historical trends in customer acquisition, retention rates, and product adoption patterns need close scrutiny, as they often provide insight into the company's overall strength and sustainability.
Ally's financial outlook hinges on its ability to capitalize on emerging market opportunities and solidify its position within the digital financial services space. Significant growth potential exists in areas such as fintech integration, personalized financial planning tools, and the development of innovative payment solutions. However, the company faces a number of obstacles. High levels of customer acquisition costs, the increasing sophistication of competitors' offerings, and a need for constant innovation to maintain relevance within the rapidly evolving technological landscape can potentially impact profitability and growth trajectory. Careful consideration needs to be given to the efficient allocation of resources to achieve long-term objectives in light of these challenges. Sustained profitability hinges on a robust customer base, consistent revenue generation, and effective cost management.
The current economic climate also presents a significant factor that will directly affect Ally's performance. Inflationary pressures, interest rate fluctuations, and changes in consumer spending habits are expected to influence market dynamics. The company's ability to adapt to evolving economic conditions through strategic planning and proactive risk management will play a vital role in determining the long-term success of its financial strategies. Analyzing industry trends and economic forecasts can provide crucial insights for Ally to develop effective countermeasures and adapt its business plan in response to various economic variables. This analysis must consider the potential impact on market demand, pricing models, and overall operational efficiency.
Predicting the future financial performance of Digital Ally is challenging. A positive outlook depends on its ability to maintain a consistent growth trajectory in revenue, reduce customer acquisition costs, and improve operational efficiency. Further, the success of new product development and strategic partnerships will be crucial in solidifying its position. However, potential risks include increased competition, rapid technological advancements that may render existing solutions obsolete, and macroeconomic uncertainties. The successful execution of the company's strategic plan, combined with effective financial management, will be essential to mitigate these risks. If Ally fails to effectively adapt to market dynamics and proactively address these concerns, future financial performance may be negatively affected. A significant downturn in the broader financial sector could also severely impact Ally's future prospects.
Rating | Short-Term | Long-Term Senior |
---|---|---|
Outlook | B2 | B1 |
Income Statement | B1 | Ba3 |
Balance Sheet | B2 | Caa2 |
Leverage Ratios | C | C |
Cash Flow | Baa2 | Ba3 |
Rates of Return and Profitability | Caa2 | Baa2 |
*Financial analysis is the process of evaluating a company's financial performance and position by neural network. It involves reviewing the company's financial statements, including the balance sheet, income statement, and cash flow statement, as well as other financial reports and documents.
How does neural network examine financial reports and understand financial state of the company?
References
- R. Howard and J. Matheson. Risk sensitive Markov decision processes. Management Science, 18(7):356– 369, 1972
- Hoerl AE, Kennard RW. 1970. Ridge regression: biased estimation for nonorthogonal problems. Technometrics 12:55–67
- Bottou L. 1998. Online learning and stochastic approximations. In On-Line Learning in Neural Networks, ed. D Saad, pp. 9–42. New York: ACM
- Canova, F. B. E. Hansen (1995), "Are seasonal patterns constant over time? A test for seasonal stability," Journal of Business and Economic Statistics, 13, 237–252.
- Athey S, Imbens GW. 2017a. The econometrics of randomized experiments. In Handbook of Economic Field Experiments, Vol. 1, ed. E Duflo, A Banerjee, pp. 73–140. Amsterdam: Elsevier
- Hartigan JA, Wong MA. 1979. Algorithm as 136: a k-means clustering algorithm. J. R. Stat. Soc. Ser. C 28:100–8
- Doudchenko N, Imbens GW. 2016. Balancing, regression, difference-in-differences and synthetic control methods: a synthesis. NBER Work. Pap. 22791