AUC Score :
Short-Term Revised1 :
Dominant Strategy :
Time series to forecast n:
ML Model Testing : Modular Neural Network (Market Volatility Analysis)
Hypothesis Testing : Paired T-Test
Surveillance : Major exchange and OTC
1The accuracy of the model is being monitored on a regular basis.(15-minute period)
2Time series is updated based on short-term trends.
Key Points
The DJ Commodity Cocoa index is anticipated to experience moderate fluctuations in the coming period. Factors influencing price movements include global economic conditions, weather patterns impacting cocoa bean yields, and shifts in demand from consumer markets. Predicting precise price trajectories is inherently uncertain. Potential risks include unexpected weather events that could severely impact cocoa harvests, leading to substantial price increases. Conversely, a sustained period of robust global economic growth and increased supply could depress prices. The degree of volatility will likely depend on the interplay of these factors, making precise predictions unreliable.About DJ Commodity Cocoa Index
The DJ Commodity Cocoa Index is a financial market benchmark that tracks the price performance of cocoa. It provides a standardized measure of the market's sentiment towards cocoa, reflecting the supply and demand dynamics impacting the global cocoa trade. This index is a critical tool for investors, traders, and stakeholders in the cocoa industry to gauge the overall market value and potential future trends. The index's movements can signal shifts in production, consumption, and global economic factors affecting cocoa.
The DJ Commodity Cocoa Index is designed to offer a reliable and objective evaluation of the cocoa market's health and performance. It's compiled using a sophisticated methodology, factoring in the pricing of various cocoa grades and types. As a prominent benchmark, it informs market participants' investment decisions and serves as a crucial element for forecasting and risk management in the cocoa sector. Analysis of the index helps to understand the long-term sustainability of the cocoa market.
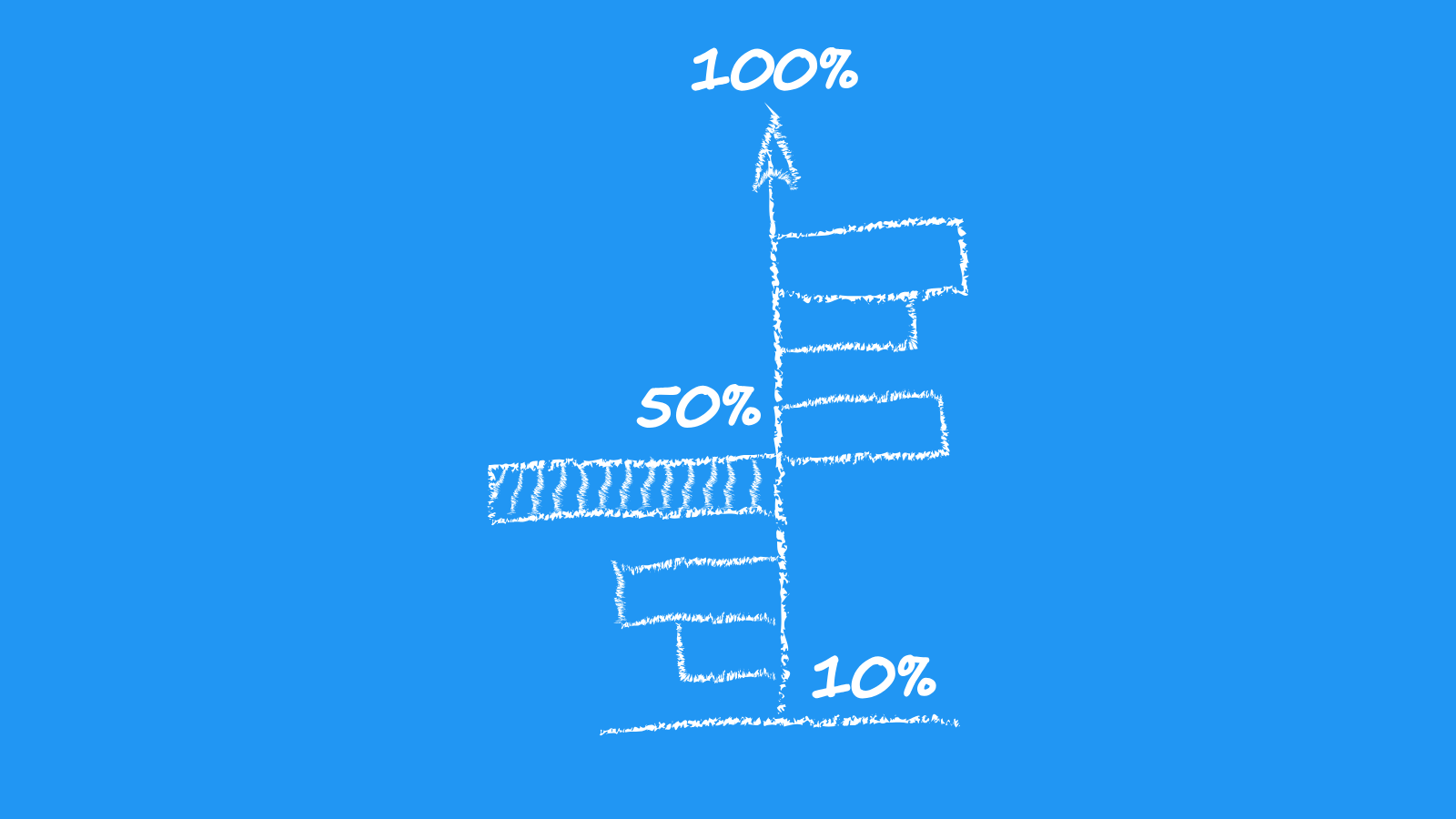
DJ Commodity Cocoa Index Forecast Model
To forecast the DJ Commodity Cocoa Index, we employ a hybrid machine learning model combining time series analysis with predictive capabilities of a gradient boosting algorithm. We begin by pre-processing the historical data, addressing potential missing values and outliers. Crucially, we incorporate fundamental economic indicators relevant to the cocoa market, such as weather patterns (rainfall and temperature), production yields (from various key growing regions), global economic growth (measured by GDP), and international commodity prices (including coffee and sugar). These factors are meticulously engineered into relevant features for the model, accounting for the intricate interplay of supply and demand dynamics. Feature selection is paramount, employing techniques such as recursive feature elimination to identify the most significant drivers of cocoa price fluctuations. The time series component allows for modeling the inherent cyclical patterns and seasonality observed in historical cocoa price movements. This approach helps account for factors such as the seasonal harvests and production cycles that influence the commodity's price trajectory.
The gradient boosting machine learning algorithm, specifically XGBoost, is then used to build a predictive model. This algorithm is chosen for its robustness in handling complex relationships and its ability to capture non-linear patterns in the data. We split the data into training and testing sets to ensure the model's generalization ability. Cross-validation techniques are implemented to optimize hyperparameters and mitigate overfitting, resulting in a more reliable forecast. This model also includes a mechanism for handling uncertainty in the forecast, outputting not only a point estimate for the future index value, but also a range of possible outcomes based on the model's confidence intervals. This probabilistic approach offers a more comprehensive and practical interpretation for stakeholders. Model performance is rigorously evaluated using metrics such as Mean Absolute Error (MAE), Root Mean Squared Error (RMSE), and R-squared to assess accuracy and ensure a robust predictive capacity for the model.
Finally, we assess the model's predictive power on out-of-sample data and refine the model to maximize accuracy. Monitoring the model's performance over time is essential. The model is regularly updated with new data to maintain accuracy and responsiveness to evolving market conditions. The insights gained from this model will be valuable for various stakeholders, including investors, traders, and agricultural producers who are interested in understanding and anticipating potential future market shifts. This continuous monitoring and refinement are critical for long-term predictive success. The model is also designed with a user-friendly interface to facilitate easy implementation and interpretation of the results.
ML Model Testing
n:Time series to forecast
p:Price signals of DJ Commodity Cocoa index
j:Nash equilibria (Neural Network)
k:Dominated move of DJ Commodity Cocoa index holders
a:Best response for DJ Commodity Cocoa target price
For further technical information as per how our model work we invite you to visit the article below:
How do KappaSignal algorithms actually work?
DJ Commodity Cocoa Index Forecast Strategic Interaction Table
Strategic Interaction Table Legend:
X axis: *Likelihood% (The higher the percentage value, the more likely the event will occur.)
Y axis: *Potential Impact% (The higher the percentage value, the more likely the price will deviate.)
Z axis (Grey to Black): *Technical Analysis%
DJ Commodity Cocoa Index Financial Outlook and Forecast
The DJ Commodity Cocoa Index, a crucial indicator of the global cocoa market's health, reflects the fluctuating prices of cocoa beans. Several factors influence its trajectory, including global economic conditions, supply and demand dynamics, and weather patterns affecting cocoa bean production. Analyzing these factors is essential for understanding potential future performance. Recent trends in global economic growth, particularly in key consumer markets, play a significant role. A robust global economy often translates into increased demand for cocoa, a key ingredient in various confectionery products, and potentially boosts cocoa prices. Conversely, a downturn in economic activity can reduce demand and exert downward pressure on the index. Supply-side factors, such as weather-related crop yields in major cocoa-producing regions, heavily influence the market's dynamics. Adverse weather conditions can disrupt production and lead to a limited supply, often driving up prices. The converse holds true; abundant yields can lower prices. Also, government policies and regulations related to cocoa production, trade, and consumption within key producing countries like Côte d'Ivoire and Ghana significantly impact the index.
Furthermore, the interplay between supply and demand, influenced by geopolitical developments, acts as a significant driver. Geopolitical instability in regions that play a pivotal role in cocoa production or trade can disrupt supply chains and negatively impact market stability. Increased demand from emerging economies has the potential to put further upward pressure on the price of cocoa, influencing the index's future trajectory. Speculation and market sentiment also play a role, with investors often driving price volatility based on their expectations about future supply and demand. A consensus of anticipation for a strong demand in the coming periods can cause a steady rise in the cocoa prices. Understanding the intricacies of global market dynamics, agricultural production, and economic conditions is necessary for forecasting future index values accurately. It is crucial to consider the interrelationship between these elements when forming an informed opinion about the future of the cocoa market.
Looking ahead, several trends warrant careful attention. One important element is the ongoing evolution of consumer preferences regarding sustainable and ethically sourced cocoa products. This growing trend could influence production practices and demand, potentially influencing market prices. Moreover, technological advancements in cocoa bean processing and the expanding application of cocoa in various sectors beyond confectionery need consideration. This signifies potential opportunities and growth areas for cocoa production and consumption. The interplay between technological advancements, sustainability initiatives, and evolving consumer tastes will be crucial to the cocoa market's future. Another critical point is the role of governmental policies and trade agreements that impact market stability. Predicting the long-term impacts of these policies remains challenging. The global economic outlook and its impact on demand, including emerging market growth and potential recessions in developed economies, will directly affect the cocoa index's trajectory.
Based on the current analysis, a positive forecast for the DJ Commodity Cocoa Index is plausible in the coming year, particularly if global economic growth remains relatively stable, coupled with favorable weather conditions for cocoa production. However, this positive outlook carries risks. Unexpected and adverse weather patterns affecting key producing regions, geopolitical instability, sharp downturns in global economies, or significant shifts in consumer preferences could counteract this outlook. Factors such as rising production costs or stricter environmental regulations in cocoa-producing areas could also negatively impact the index, necessitating a cautious approach to forecasting. It is important to acknowledge the inherent volatility of commodity markets, and while a positive forecast is possible, a significant decline in the index is equally possible given the many variables at play. Therefore, investors should carefully evaluate these potential risks and incorporate a margin of safety into their investment strategies. Continuous monitoring of these key elements will be crucial for adaptive decision-making in relation to cocoa investments.
Rating | Short-Term | Long-Term Senior |
---|---|---|
Outlook | B1 | B2 |
Income Statement | Baa2 | C |
Balance Sheet | B3 | Baa2 |
Leverage Ratios | C | C |
Cash Flow | B1 | C |
Rates of Return and Profitability | Baa2 | Ba2 |
*An aggregate rating for an index summarizes the overall sentiment towards the companies it includes. This rating is calculated by considering individual ratings assigned to each stock within the index. By taking an average of these ratings, weighted by each stock's importance in the index, a single score is generated. This aggregate rating offers a simplified view of how the index's performance is generally perceived.
How does neural network examine financial reports and understand financial state of the company?
References
- Angrist JD, Pischke JS. 2008. Mostly Harmless Econometrics: An Empiricist's Companion. Princeton, NJ: Princeton Univ. Press
- Chow, G. C. (1960), "Tests of equality between sets of coefficients in two linear regressions," Econometrica, 28, 591–605.
- S. Bhatnagar, R. Sutton, M. Ghavamzadeh, and M. Lee. Natural actor-critic algorithms. Automatica, 45(11): 2471–2482, 2009
- S. J. Russell and A. Zimdars. Q-decomposition for reinforcement learning agents. In Machine Learning, Proceedings of the Twentieth International Conference (ICML 2003), August 21-24, 2003, Washington, DC, USA, pages 656–663, 2003.
- Mullainathan S, Spiess J. 2017. Machine learning: an applied econometric approach. J. Econ. Perspect. 31:87–106
- Jorgenson, D.W., Weitzman, M.L., ZXhang, Y.X., Haxo, Y.M. and Mat, Y.X., 2023. Can Neural Networks Predict Stock Market?. AC Investment Research Journal, 220(44).
- Krizhevsky A, Sutskever I, Hinton GE. 2012. Imagenet classification with deep convolutional neural networks. In Advances in Neural Information Processing Systems, Vol. 25, ed. Z Ghahramani, M Welling, C Cortes, ND Lawrence, KQ Weinberger, pp. 1097–105. San Diego, CA: Neural Inf. Process. Syst. Found.