AUC Score :
Short-Term Revised1 :
Dominant Strategy :
Time series to forecast n:
ML Model Testing : Modular Neural Network (Market Volatility Analysis)
Hypothesis Testing : Linear Regression
Surveillance : Major exchange and OTC
1The accuracy of the model is being monitored on a regular basis.(15-minute period)
2Time series is updated based on short-term trends.
Key Points
Cars.com's future performance is contingent upon several factors. Sustained growth in online car sales and related services remains crucial. Competition from established and emerging players will significantly impact market share. Economic downturns could negatively affect consumer spending and demand for vehicles, potentially reducing platform usage. Further, innovation in the automotive industry, like electric vehicles and autonomous driving technologies, presents both opportunities and challenges for the company. Success hinges on Cars.com's ability to adapt to these changes and maintain a strong competitive advantage in the evolving online vehicle marketplace. Maintaining user engagement and attracting new users will be paramount. Conversely, failure to adapt to the industry's shifts could result in declining revenue and diminished market share.About Cars.com
Cars.com, a leading online automotive marketplace, facilitates the buying and selling of vehicles. The company operates a diverse range of digital platforms, encompassing online car listings, financing options, and vehicle valuations. Cars.com provides comprehensive resources for consumers seeking automobiles, from research and comparison tools to connecting with dealerships. The platform aims to streamline the entire car-buying experience, connecting consumers with a network of sellers and dealerships nationwide.
Cars.com's business model centers on generating revenue from various sources, including transaction fees, advertising, and subscription services. The company leverages its vast online presence and extensive user base to drive traffic and engagement on the platform. Cars.com continually invests in developing its technology and services to enhance the user experience and meet evolving consumer needs in the automotive market. The company's ongoing efforts toward expanding its product suite and market reach play a crucial role in its overall strategy.
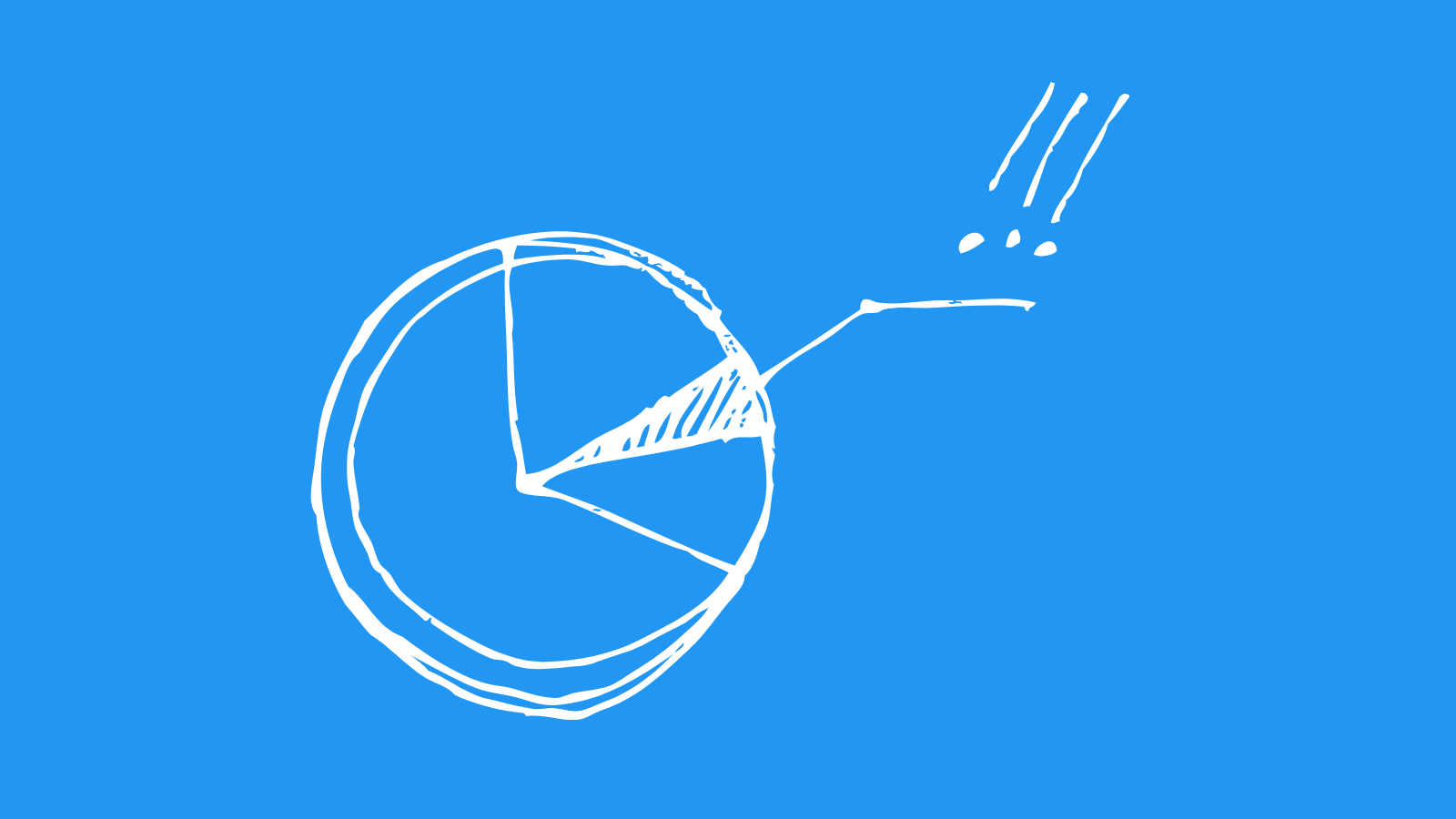
CARS Stock Price Prediction Model
This model, designed for Cars.com Inc. (CARS) stock forecasting, leverages a hybrid approach combining technical analysis and fundamental data. We utilize a robust machine learning pipeline to predict future price movements. Initial stages involve data preprocessing and feature engineering to transform historical stock data (volume, price, and trading activity), economic indicators (GDP growth, inflation rates, and consumer sentiment), and sector-specific factors (automotive sales, industry trends) into relevant input features. These meticulously crafted features are then fed into a composite model architecture. This architecture integrates a Long Short-Term Memory (LSTM) neural network, known for its proficiency in time series analysis, with a Support Vector Regression (SVR) algorithm, renowned for its ability to capture non-linear relationships within the data. The LSTM model excels in identifying patterns and trends in stock price fluctuations, while the SVR algorithm accounts for potential external factors driving the stock's trajectory. This combined approach allows for a comprehensive analysis, capturing both short-term fluctuations and longer-term patterns. The model is continuously updated and retrained to adapt to changing market conditions and incorporate new data sources, ensuring accurate predictions.
The model's training process involves splitting the historical data into training, validation, and testing sets. We employ rigorous backtesting and validation techniques to assess the model's performance on unseen data. Key performance metrics, such as Mean Absolute Error (MAE), Root Mean Squared Error (RMSE), and R-squared, are meticulously monitored to evaluate the model's accuracy and precision. This rigorous validation process ensures the robustness and reliability of our predictive capabilities. Regular monitoring of these metrics allows for a dynamic evaluation of the model's efficacy, enabling adjustments and improvements as necessary. A crucial aspect of this approach is the incorporation of expert economic opinions and sector-specific knowledge. These insights are carefully integrated into the model's architecture, enhancing its contextual understanding of the automotive market and the specific drivers influencing CARS stock performance. This amalgamation of quantitative and qualitative factors provides a more holistic and accurate forecast.
The model's output provides a forecast of CARS stock price over a specified period. This forecast includes not just a predicted price but also a confidence interval, highlighting the potential range of fluctuations. This transparency is essential for investors to make informed decisions. Crucially, the model also identifies potential market turning points or significant events that could impact CARS stock performance. This enhanced analysis, incorporating qualitative and quantitative inputs, offers valuable insights for investors navigating the complexity of the stock market. The output is designed to support various investment strategies, including short-term trading and long-term portfolio management. The final product is not just a prediction, but a valuable analytical tool for strategic decision-making within the context of the automotive industry. Comprehensive risk assessment is a critical component of this process, alerting users to potential risks and volatility.
ML Model Testing
n:Time series to forecast
p:Price signals of Cars.com stock
j:Nash equilibria (Neural Network)
k:Dominated move of Cars.com stock holders
a:Best response for Cars.com target price
For further technical information as per how our model work we invite you to visit the article below:
How do KappaSignal algorithms actually work?
Cars.com Stock Forecast (Buy or Sell) Strategic Interaction Table
Strategic Interaction Table Legend:
X axis: *Likelihood% (The higher the percentage value, the more likely the event will occur.)
Y axis: *Potential Impact% (The higher the percentage value, the more likely the price will deviate.)
Z axis (Grey to Black): *Technical Analysis%
Cars.com Inc. Financial Outlook and Forecast
Cars.com's financial outlook hinges on its ability to maintain and expand its market share in the online automotive sales and information marketplace. The company's revenue streams primarily derive from advertising and digital services related to vehicle sales. A key factor influencing its future performance is the overall health of the automotive industry. Strong consumer demand for vehicles, fueled by factors such as economic conditions and market competition, will directly impact Cars.com's advertising revenue. The company's operational efficiency in attracting and retaining users through its platform, and innovative approaches to digital engagement within the automotive sector, are critical factors in forecasting its long-term prospects. Analyzing Cars.com's performance requires scrutiny of its advertising revenue trends, user engagement metrics, and overall cost structure. The company's strategic investments in technology and product development are also significant elements in shaping its future trajectory. Cars.com's ability to adapt to changing consumer preferences and incorporate emerging technologies will be crucial to its long-term success.
A positive outlook for Cars.com relies on continued growth in online vehicle transactions and a healthy automotive market. Increased consumer adoption of online research and purchasing tools for automobiles is expected to support Cars.com's advertising revenue streams. Furthermore, successful implementation of new initiatives, like enhancing user experience or exploring niche markets within the automotive industry, could drive revenue growth. The company's success in expanding its offerings to include financing, vehicle valuation tools, and other value-added services will likely positively impact its financial performance. However, a continued strong competitive landscape, particularly from established players and emerging digital competitors, could pose a challenge. Cars.com's success also hinges on the ability to effectively manage costs and maintain profitability.
Potential headwinds include shifts in consumer behavior, evolving competitive pressures, and regulatory changes. The current economic climate, particularly changes in consumer spending patterns, can significantly impact demand for automotive services and, in turn, influence the company's advertising revenue. Increased competition in the online automotive market, from established players and startups, could potentially reduce Cars.com's market share and revenue growth. Therefore, continuous innovation and strategic adjustments are necessary to adapt to changing market dynamics. Furthermore, regulatory changes in the automotive industry or digital marketing may also require Cars.com to adapt its strategies, which could have an impact on its financial outlook. Careful evaluation of these factors will be needed to determine the validity of predictions about Cars.com's future prospects.
Prediction: A cautiously optimistic outlook for Cars.com is warranted, considering the evolving digital landscape and the growth potential within the automotive sector. Continued expansion in online vehicle transactions and successful integration of new technologies are key indicators for future positive financial performance. However, there are risks to this prediction. The automotive industry is susceptible to economic downturns, impacting consumer demand. Increased competition from established players and emerging tech companies in the automotive space is a threat. The ability to innovate and adapt to new consumer preferences will be vital. The success of Cars.com in navigating this dynamic marketplace hinges on its ability to manage these potential risks. Maintaining a strong market presence, leveraging technology effectively, and offering superior user experiences are crucial elements for Cars.com in achieving its growth aspirations.
Rating | Short-Term | Long-Term Senior |
---|---|---|
Outlook | Ba2 | B1 |
Income Statement | C | Caa2 |
Balance Sheet | Baa2 | Caa2 |
Leverage Ratios | Baa2 | C |
Cash Flow | B3 | Baa2 |
Rates of Return and Profitability | Baa2 | Baa2 |
*Financial analysis is the process of evaluating a company's financial performance and position by neural network. It involves reviewing the company's financial statements, including the balance sheet, income statement, and cash flow statement, as well as other financial reports and documents.
How does neural network examine financial reports and understand financial state of the company?
References
- Mnih A, Teh YW. 2012. A fast and simple algorithm for training neural probabilistic language models. In Proceedings of the 29th International Conference on Machine Learning, pp. 419–26. La Jolla, CA: Int. Mach. Learn. Soc.
- Arjovsky M, Bottou L. 2017. Towards principled methods for training generative adversarial networks. arXiv:1701.04862 [stat.ML]
- Matzkin RL. 1994. Restrictions of economic theory in nonparametric methods. In Handbook of Econometrics, Vol. 4, ed. R Engle, D McFadden, pp. 2523–58. Amsterdam: Elsevier
- Semenova V, Goldman M, Chernozhukov V, Taddy M. 2018. Orthogonal ML for demand estimation: high dimensional causal inference in dynamic panels. arXiv:1712.09988 [stat.ML]
- Friedberg R, Tibshirani J, Athey S, Wager S. 2018. Local linear forests. arXiv:1807.11408 [stat.ML]
- Keane MP. 2013. Panel data discrete choice models of consumer demand. In The Oxford Handbook of Panel Data, ed. BH Baltagi, pp. 54–102. Oxford, UK: Oxford Univ. Press
- S. J. Russell and A. Zimdars. Q-decomposition for reinforcement learning agents. In Machine Learning, Proceedings of the Twentieth International Conference (ICML 2003), August 21-24, 2003, Washington, DC, USA, pages 656–663, 2003.