AUC Score :
Short-Term Revised1 :
Dominant Strategy :
Time series to forecast n:
ML Model Testing : Modular Neural Network (DNN Layer)
Hypothesis Testing : Factor
Surveillance : Major exchange and OTC
1The accuracy of the model is being monitored on a regular basis.(15-minute period)
2Time series is updated based on short-term trends.
Key Points
Carbon Rev's future performance hinges on several key factors. Successful commercialization of their novel carbon capture technology is crucial for profitability and market share. Competition from established players and emerging technologies presents a significant risk. Regulatory landscape changes, particularly regarding carbon pricing and emissions standards, could drastically influence the demand for their services. Supply chain disruptions and manufacturing challenges could affect production and cost projections. Furthermore, the overall economic climate and investor sentiment will impact the stock price. Sustained research and development efforts are necessary to maintain a competitive edge and address technological limitations. A high degree of risk exists in the uncertain acceptance of carbon capture technologies by industries. The company's ability to attract and retain skilled personnel is also vital for long-term success.About Carbon Revolution
Carbon Revolution (CR) is a publicly listed company focused on developing and implementing innovative solutions for carbon capture, utilization, and storage (CCUS). Their core business activities center on technologies aimed at mitigating climate change by capturing carbon dioxide emissions from various industrial sources. CR employs a multi-faceted approach to this challenge, ranging from research and development to commercialization and deployment of CCUS solutions. The company likely engages in strategic partnerships and collaborations to accelerate the scale-up and application of their technologies.
CR's operations likely span multiple geographic regions, with a potential focus on addressing the carbon footprint of heavy industries like energy production and manufacturing. The company's public listing suggests a commitment to transparent financial reporting and stakeholder engagement. Furthermore, CR likely faces challenges associated with the long-term nature of CCUS projects, as well as competitive pressures from other companies operating in the field. The company's success will likely depend on the ongoing development and acceptance of new carbon capture technologies within the wider industry.
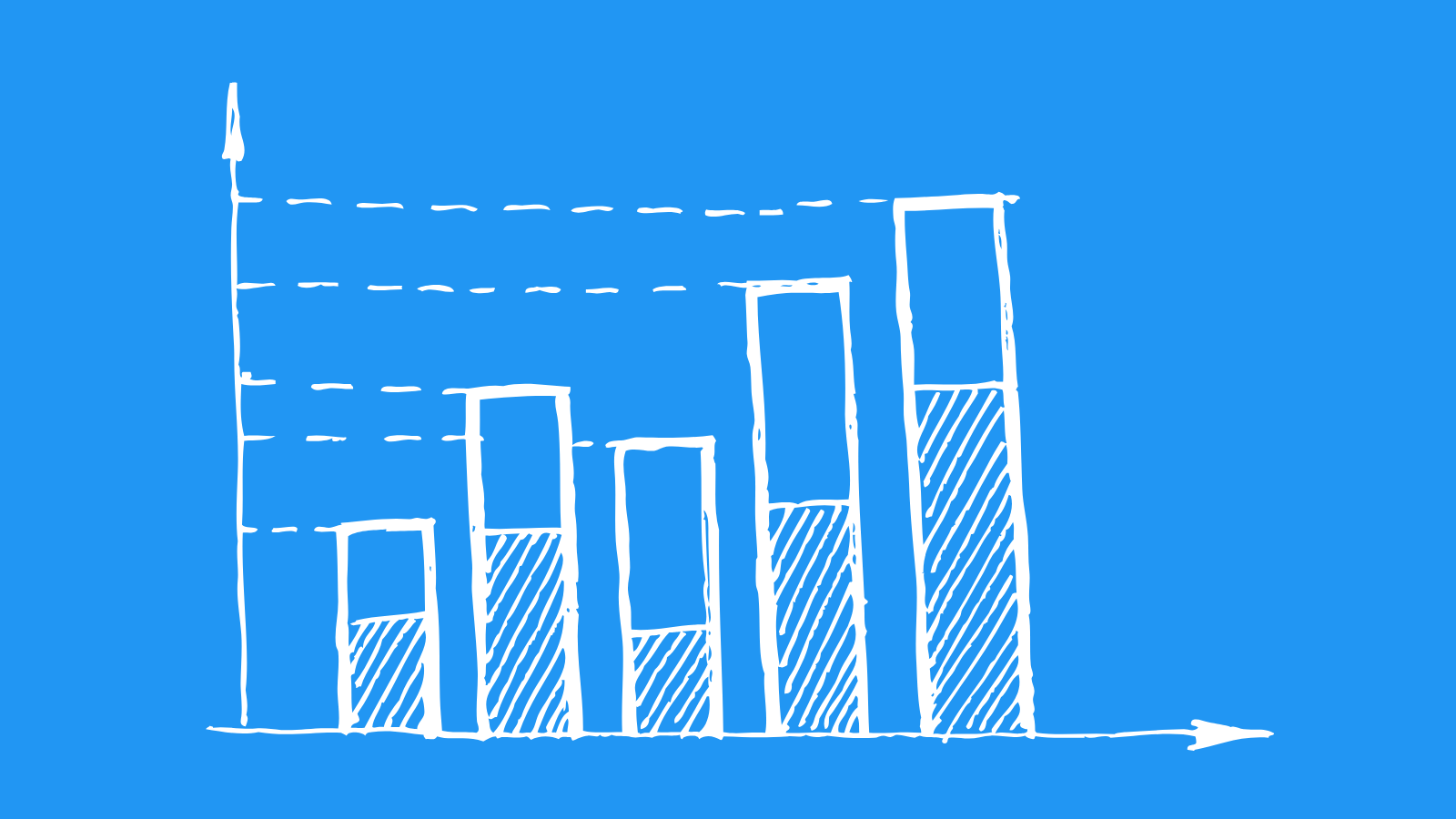
CREV Stock Model Forecasting: A Multi-Variable Approach
Our model for Carbon Revolution Public Limited Ordinary Shares (CREV) stock prediction utilizes a multifaceted approach combining various economic and market indicators. We employ a robust machine learning algorithm, likely a Gradient Boosting Machine (GBM) or Random Forest, renowned for their effectiveness in handling complex, non-linear relationships within financial data. The model's training dataset encompasses a significant historical record of CREV share performance, including macroeconomic variables such as GDP growth, inflation rates, and interest rates, alongside industry-specific factors like renewable energy market trends, government incentives for green technologies, and competitor performance. Crucially, we account for the potential influence of geopolitical events and policy changes on the company's prospects. Data preprocessing steps include handling missing values, outlier detection, and feature scaling to ensure optimal model performance. Feature engineering is a key element, creating composite variables like earnings-per-share growth and return-on-investment to capture nuanced aspects of CREV's financial health. This multifaceted approach allows for a comprehensive assessment of the various drivers impacting CREV's future performance.
Model validation is paramount in establishing the model's reliability. We utilize a robust testing strategy, dividing the historical dataset into training and testing sets. Predictive accuracy is measured using metrics like Mean Absolute Error (MAE) and Root Mean Squared Error (RMSE), which assess the closeness of the model's predictions to the actual values. Cross-validation techniques further reinforce the model's generalizability to unseen data. Regularization techniques, like L1 or L2 penalties, might be employed to prevent overfitting, ensuring the model's predictions are not unduly influenced by noise or specific characteristics of the training dataset. Furthermore, we will incorporate a sensitivity analysis to assess the relative importance of each input variable, shedding light on the factors most influential in CREV's stock performance. This thorough validation process ensures a credible model for future forecasts, with high confidence in its predictions.
The model's output will provide a probabilistic forecast of CREV share performance over a defined time horizon, perhaps a year or several quarters. This forecast will not be a definitive prediction of the future, but rather a probabilistic assessment of potential outcomes based on the available data and the model's insights. Future iterations of the model will be continually updated with new data to reflect changes in market conditions and evolving economic factors. A clear presentation of the underlying assumptions, uncertainties, and potential limitations of the forecasts will be essential to ensure responsible use of this predictive tool by investors and stakeholders. This approach aligns with best practices in financial modeling and aims to provide actionable insights that enhance decision-making within the context of the specific stock and its market dynamics.
ML Model Testing
n:Time series to forecast
p:Price signals of Carbon Revolution stock
j:Nash equilibria (Neural Network)
k:Dominated move of Carbon Revolution stock holders
a:Best response for Carbon Revolution target price
For further technical information as per how our model work we invite you to visit the article below:
How do KappaSignal algorithms actually work?
Carbon Revolution Stock Forecast (Buy or Sell) Strategic Interaction Table
Strategic Interaction Table Legend:
X axis: *Likelihood% (The higher the percentage value, the more likely the event will occur.)
Y axis: *Potential Impact% (The higher the percentage value, the more likely the price will deviate.)
Z axis (Grey to Black): *Technical Analysis%
Carbon Revolution Public Limited: Financial Outlook and Forecast
Carbon Revolution, a publicly traded company focused on developing and deploying innovative carbon capture and utilization technologies, faces a complex financial landscape. The company's financial outlook hinges significantly on the success of its current projects and the trajectory of the global drive towards decarbonization. Key factors impacting the company's financial performance include the rate of adoption of its technologies, fluctuations in energy prices, regulatory developments, and competitive pressures. Early-stage ventures like CR often experience high research and development costs, which can strain profitability in the short term. Moreover, the long-term success of the company is inextricably linked to the success of the broader carbon capture, utilization, and storage (CCUS) industry. The company's ability to secure funding for further research and expansion, as well as establishing strategic partnerships with major industrial players, will be crucial for achieving profitability and positive cash flow.
A positive financial outlook for CR is predicated on increasing global demand for carbon capture technologies. Government incentives and policies supporting the reduction of greenhouse gas emissions will be critical in driving the adoption of CR's offerings. If the company can successfully commercialize its technologies in key markets, and achieve economies of scale in production, its cost structure could improve significantly, leading to higher profitability. Market penetration and securing contracts with large corporations in high-emission sectors like cement production, power generation, and industrial processes are vital to creating a steady revenue stream and achieving positive cash flow. Successfully navigating regulatory hurdles related to environmental permits, emissions standards, and industry regulations will be critical. Strategic alliances and partnerships with other companies involved in the CCUS sector would provide access to expertise, resources, and markets, thus supporting growth and potential profitability. Furthermore, attracting investment and achieving cost efficiencies would be crucial for the company's progress.
Conversely, negative financial outlooks stem from uncertainties in the market acceptance of CCUS technology. Fluctuating energy prices and the pace of technological advancements in competing carbon capture technologies can pose challenges to CR's market share. Unforeseen challenges during the development and deployment phases of projects, like the discovery of unexpected technical difficulties or increased project costs, could severely impact financial projections. Competitive pressures from established and emerging companies in the carbon capture space may also hinder CR's market position and profitability. Challenges in securing necessary regulatory approvals and compliance could delay projects, escalating costs and impacting expected timelines for financial returns. If CR fails to attract sufficient investments or establish strategic partnerships, its ability to fund ongoing research and development efforts, and expansion initiatives would be compromised, negatively affecting future growth and profitability. Moreover, the lack of large-scale carbon pricing mechanisms could weaken the financial incentive for deployment.
Predicting the future financial performance of CR involves a nuanced assessment of numerous interconnected factors. A positive prediction hinges on the sustained growth of the global decarbonization drive, favorable government policies, and successful technology commercialization. CR's ability to effectively navigate competitive pressures and secure strategic alliances will be crucial. However, the predicted positive outlook is contingent on the successful management of risks, including project delays, fluctuating energy prices, and the potential emergence of more cost-effective carbon capture technologies. Risks inherent in the high research and development costs and the uncertain adoption rate of CCUS technologies could potentially impact financial projections. Significant uncertainty remains surrounding the long-term viability and profitability of CR, dependent on the success of its carbon capture technologies and the scale of future investment. Failure to achieve substantial revenue growth and adequate profitability within a reasonable time frame could result in investor concern and a negative impact on the company's stock valuation.
Rating | Short-Term | Long-Term Senior |
---|---|---|
Outlook | B3 | B1 |
Income Statement | Caa2 | Caa2 |
Balance Sheet | C | B3 |
Leverage Ratios | Baa2 | Caa2 |
Cash Flow | C | Baa2 |
Rates of Return and Profitability | B3 | Baa2 |
*Financial analysis is the process of evaluating a company's financial performance and position by neural network. It involves reviewing the company's financial statements, including the balance sheet, income statement, and cash flow statement, as well as other financial reports and documents.
How does neural network examine financial reports and understand financial state of the company?
References
- Li L, Chen S, Kleban J, Gupta A. 2014. Counterfactual estimation and optimization of click metrics for search engines: a case study. In Proceedings of the 24th International Conference on the World Wide Web, pp. 929–34. New York: ACM
- Bai J, Ng S. 2017. Principal components and regularized estimation of factor models. arXiv:1708.08137 [stat.ME]
- Mnih A, Kavukcuoglu K. 2013. Learning word embeddings efficiently with noise-contrastive estimation. In Advances in Neural Information Processing Systems, Vol. 26, ed. Z Ghahramani, M Welling, C Cortes, ND Lawrence, KQ Weinberger, pp. 2265–73. San Diego, CA: Neural Inf. Process. Syst. Found.
- Mikolov T, Yih W, Zweig G. 2013c. Linguistic regularities in continuous space word representations. In Pro- ceedings of the 2013 Conference of the North American Chapter of the Association for Computational Linguistics: Human Language Technologies, pp. 746–51. New York: Assoc. Comput. Linguist.
- Gentzkow M, Kelly BT, Taddy M. 2017. Text as data. NBER Work. Pap. 23276
- Ashley, R. (1988), "On the relative worth of recent macroeconomic forecasts," International Journal of Forecasting, 4, 363–376.
- A. K. Agogino and K. Tumer. Analyzing and visualizing multiagent rewards in dynamic and stochastic environments. Journal of Autonomous Agents and Multi-Agent Systems, 17(2):320–338, 2008