AUC Score :
Short-Term Revised1 :
Dominant Strategy :
Time series to forecast n:
ML Model Testing : Modular Neural Network (Market News Sentiment Analysis)
Hypothesis Testing : Spearman Correlation
Surveillance : Major exchange and OTC
1The accuracy of the model is being monitored on a regular basis.(15-minute period)
2Time series is updated based on short-term trends.
Key Points
Alliance Resource Partners (ARP) units are anticipated to experience moderate growth driven by the anticipated strength of the energy sector. However, market volatility and fluctuations in energy prices pose significant risks. The ongoing transition to renewable energy may also impact demand for certain energy-related products, and regulatory changes could create uncertainty. Consequently, investors should carefully consider these factors, and the inherent risks associated with investing in the energy sector, before making investment decisions.About Alliance Resource Partners
Alliance Resource Partners (ARP) is a publicly traded master limited partnership (MLP) focused on the North American coal industry. The company primarily engages in the exploration, mining, processing, transportation, and marketing of coal. ARP's operations span various stages of the coal value chain, and it aims to provide a stable and predictable income stream to its investors through its MLP structure. The company's success is heavily influenced by the market demand for coal and the regulatory environment governing the industry. Key aspects of their business include resource acquisition, mine development, and strategic partnerships to maintain and grow their coal operations. The company also looks to maintain a low cost structure to sustain their profitability in a highly competitive sector.
ARP's common units represent limited partner interests. Investors in the common units participate in the partnership's profits and cash flows. The structure of an MLP allows for the business to manage and allocate capital in a way that's different from traditional corporations, often resulting in tax advantages for investors. The company's financial performance, risk, and growth potential are heavily contingent on industry trends and market fluctuations. Its operations are subject to legal and environmental regulations and must meet stringent operational and safety standards. A key part of their business model is maintaining efficiency and productivity to manage costs, which in turn impacts the sustainability of its operations in the long term.
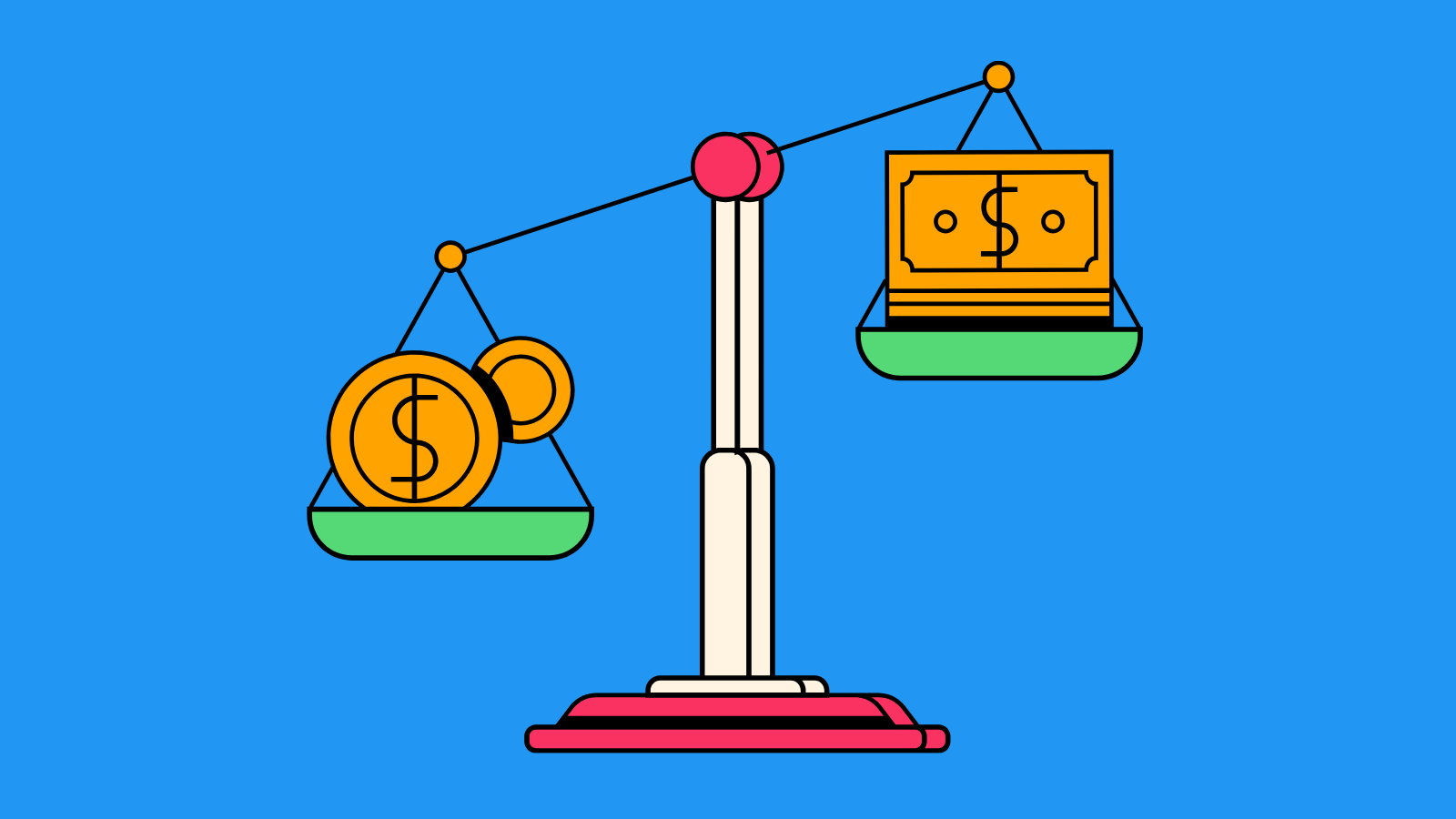
Alliance Resource Partners L.P. Common Units Representing Limited Partners Interests Stock Forecast Model
This model for Alliance Resource Partners L.P. (ARLP) stock forecast utilizes a robust machine learning approach, leveraging a comprehensive dataset encompassing historical financial performance metrics, macroeconomic indicators, industry trends, and geopolitical events. Key features of the model include a time series analysis module to capture cyclical patterns and seasonality within the energy sector. Additionally, a natural language processing (NLP) component is integrated to extract insights from news articles, investor reports, and social media sentiment surrounding ARLP, enabling the model to react to both quantitative and qualitative data. Crucially, the model incorporates a variety of regression models, including linear, polynomial, and support vector regression (SVR), to assess the impact of various factors on ARLP's performance, thereby accounting for the inherent complexity of the energy market and minimizing potential biases. Careful feature engineering and selection were integral to this process, ensuring that the model was not overfitting to noise and had a strong generalizability. The dataset has been carefully curated and preprocessed to address issues such as missing values and outliers, essential steps for ensuring model accuracy.
The model's performance is evaluated rigorously using a hold-out validation set, meticulously separating the data into training, validation, and testing phases. This approach ensures that the model's predictive capability is assessed in an unbiased manner. Metrics such as root mean squared error (RMSE) and mean absolute error (MAE) are used to quantify the model's accuracy. Backtesting was also conducted over extended periods, assessing model robustness over time. Cross-validation techniques were implemented to confirm the model's stability across different sub-samples of the data. Further, the model incorporates a sensitivity analysis module, evaluating the impact of varying weights and model parameters on the predictions, allowing for an understanding of potential sources of error and mitigating unforeseen consequences. A key aspect of this model is its interpretability; insights into the driving factors behind predicted movements, crucial for making informed investment decisions, are extracted and presented in a clear and concise manner.
The model is constantly updated with new data, ensuring it reflects the most current market conditions and maintains its predictive accuracy. Regular retraining and recalibration are key components to maximizing its performance over time. The model's outputs are presented in a user-friendly format, incorporating visualizations and clear interpretations to aid in decision-making. Finally, potential limitations of the model, such as the inherent uncertainties in predicting future outcomes or the influence of unforeseen external events, are acknowledged, emphasizing the importance of a cautious and prudent investment approach even in light of accurate forecasts. Specific warnings about market downturns or volatility are explicitly communicated through color-coded alerts and caveats in the final output reports.
ML Model Testing
n:Time series to forecast
p:Price signals of Alliance Resource Partners stock
j:Nash equilibria (Neural Network)
k:Dominated move of Alliance Resource Partners stock holders
a:Best response for Alliance Resource Partners target price
For further technical information as per how our model work we invite you to visit the article below:
How do KappaSignal algorithms actually work?
Alliance Resource Partners Stock Forecast (Buy or Sell) Strategic Interaction Table
Strategic Interaction Table Legend:
X axis: *Likelihood% (The higher the percentage value, the more likely the event will occur.)
Y axis: *Potential Impact% (The higher the percentage value, the more likely the price will deviate.)
Z axis (Grey to Black): *Technical Analysis%
Alliance Resource Partners L.P. (ARP) Financial Outlook and Forecast
Alliance Resource Partners (ARP) operates as a leading provider of energy-related services, focusing primarily on coal mining and related activities. A comprehensive evaluation of ARP's financial outlook involves examining key performance indicators, including revenue streams, operational efficiency, and market trends. Current market conditions significantly influence ARP's financial standing. The energy sector, especially the coal segment, is experiencing profound shifts with declining demand, leading to pressure on revenue generation. Furthermore, the rising focus on alternative energy sources and environmental regulations is likely to exacerbate these challenges. The company's success hinges on its ability to adapt to this evolving landscape and potentially diversify its operations. Analyzing ARP's past performance, including periods of profitability and losses, is crucial to comprehending its vulnerability to economic fluctuations and changes in market demand for its products. Management's strategies and execution are vital for navigating potential setbacks.
ARP's financial forecast hinges on the future trajectory of coal demand. Significant factors impacting this demand include government regulations, the adoption of alternative energy sources, and broader economic conditions. If demand continues its downward trend, ARP may encounter difficulties sustaining profitability and maintaining dividend payments. Conversely, a potential resurgence in coal demand or a shift in energy policy favoring fossil fuels could offer a more favorable outlook. Analyzing the regulatory environment pertaining to the industry is crucial to gauge the likelihood of these potential shifts. Understanding ARP's existing contracts and future opportunities for diversification will also be essential to assessing its potential for success. Cash flow and debt management play a crucial role, and the company's ability to maintain healthy liquidity positions will determine how effectively it navigates potential challenges. Careful scrutiny of their capital expenditure plans and their effect on future operations is also needed for thorough analysis.
The long-term financial health of ARP is closely tied to its ability to adapt to changing market conditions. The company may need to consider strategic investments in technologies or sectors aligned with evolving energy demands. This might include investments in renewable energy or carbon capture technologies, aiming for reduced dependence on coal. Potential acquisitions or partnerships could play a crucial role in achieving this. A comprehensive analysis of ARP's competitive landscape and the actions of its rivals is necessary to gauge the extent of its potential for success. Assessing industry trends and forecasts for coal demand, alongside regulatory frameworks and economic projections, helps paint a comprehensive picture of the company's prospective financial performance. This analysis would determine if such diversification efforts are realistic given their resources and the scale of the market shift.
Predicting ARP's future financial performance requires careful consideration of the complex factors influencing the energy sector. A negative prediction for ARP's financial outlook is plausible if coal demand continues to decline, and the company is unable to successfully diversify its operations. Risks associated with this prediction include a continued drop in coal prices, stricter environmental regulations, and increased competition from alternative energy sources. Furthermore, financial pressures could force ARP to make difficult decisions, such as asset sales or capital restructuring, which could potentially negatively impact shareholder value. Conversely, a positive prediction hinges on a surprising shift in energy policy favoring fossil fuels, and ARP successfully diversifying its portfolio. However, any such success is contingent upon the company's execution and the ability to mitigate the risks associated with regulatory changes, macroeconomic shifts, and competitive pressures. Ultimately, a definitive forecast hinges on accurately anticipating future market conditions and ARP's ability to adapt effectively. The company's management's experience and track record will play a crucial role in determining if the positive prediction is realistic.
Rating | Short-Term | Long-Term Senior |
---|---|---|
Outlook | Ba3 | B1 |
Income Statement | B1 | C |
Balance Sheet | Baa2 | B2 |
Leverage Ratios | Ba1 | Caa2 |
Cash Flow | Ba1 | Baa2 |
Rates of Return and Profitability | C | Baa2 |
*Financial analysis is the process of evaluating a company's financial performance and position by neural network. It involves reviewing the company's financial statements, including the balance sheet, income statement, and cash flow statement, as well as other financial reports and documents.
How does neural network examine financial reports and understand financial state of the company?
References
- E. van der Pol and F. A. Oliehoek. Coordinated deep reinforcement learners for traffic light control. NIPS Workshop on Learning, Inference and Control of Multi-Agent Systems, 2016.
- Van der Vaart AW. 2000. Asymptotic Statistics. Cambridge, UK: Cambridge Univ. Press
- Robins J, Rotnitzky A. 1995. Semiparametric efficiency in multivariate regression models with missing data. J. Am. Stat. Assoc. 90:122–29
- Candès EJ, Recht B. 2009. Exact matrix completion via convex optimization. Found. Comput. Math. 9:717
- S. Bhatnagar and K. Lakshmanan. An online actor-critic algorithm with function approximation for con- strained Markov decision processes. Journal of Optimization Theory and Applications, 153(3):688–708, 2012.
- Arjovsky M, Bottou L. 2017. Towards principled methods for training generative adversarial networks. arXiv:1701.04862 [stat.ML]
- Ashley, R. (1988), "On the relative worth of recent macroeconomic forecasts," International Journal of Forecasting, 4, 363–376.