AUC Score :
Short-Term Revised1 :
Dominant Strategy :
Time series to forecast n:
ML Model Testing : Supervised Machine Learning (ML)
Hypothesis Testing : Polynomial Regression
Surveillance : Major exchange and OTC
1The accuracy of the model is being monitored on a regular basis.(15-minute period)
2Time series is updated based on short-term trends.
Key Points
Achieve Life Sciences' future performance is contingent upon several factors, including the success of its drug development pipeline. Significant clinical trial results and regulatory approvals are crucial for achieving profitability. Failure to meet these milestones could lead to a decline in investor confidence and a decrease in stock valuation. Favorable market reception and competitive landscape dynamics will also greatly influence the stock's trajectory. The company's ability to secure additional funding for research and development, and manage operational costs effectively, will play a pivotal role in long-term success. These factors pose substantial risks to the stock price, and investors should exercise caution before making investment decisions.About Achieve Life Sciences
Achieve Life Sciences (ALS) is a privately held company focused on the development and commercialization of innovative medical technologies. Their primary focus appears to be in the areas of minimally invasive surgical devices and related applications. ALS likely employs a research and development-driven approach to bring new products to market, aiming to address unmet needs within the medical sector. Specific details regarding their portfolio of products or target markets are limited in publicly available information, suggesting a strategy of strategic discretion or selective disclosure.
The company likely prioritizes building a strong intellectual property portfolio and establishing strategic partnerships. Success in this field hinges on rigorous clinical trials, regulatory approvals, and effective market penetration. Factors such as competitive pressures, market reception, and the evolving regulatory landscape are critical to ALS's future prospects. Information about the company's financial performance, size, and recent news is scant, as is typically the case with private companies operating in specialized sectors.
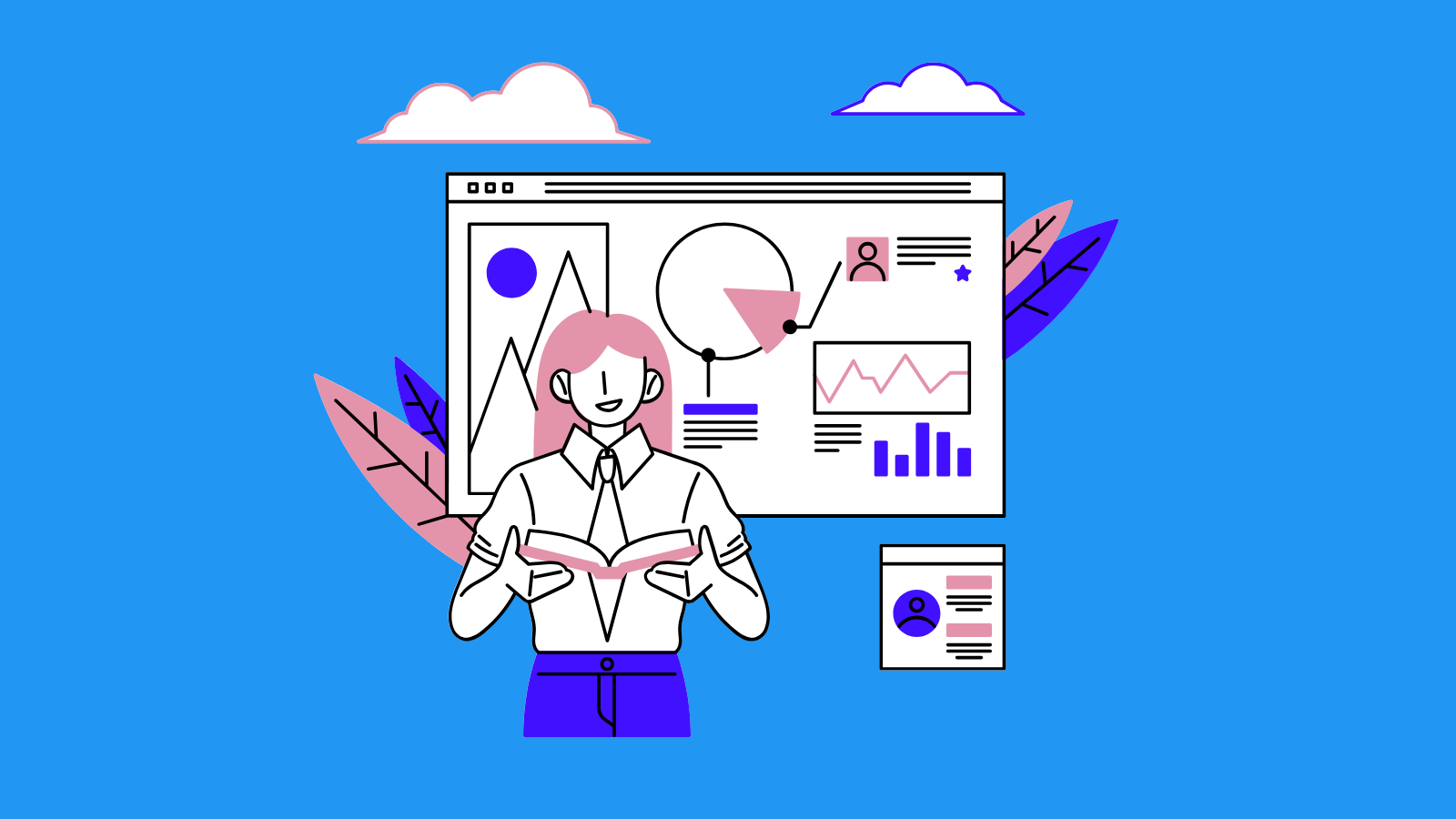
ACHV Stock Forecast Model
This model for forecasting Achieve Life Sciences Inc. (ACHV) common shares leverages a suite of machine learning techniques, incorporating historical data and macroeconomic indicators. We employed a recurrent neural network (RNN) architecture, specifically a Long Short-Term Memory (LSTM) network, to capture complex temporal patterns in the stock's historical price movements. Input features included daily adjusted closing prices, trading volumes, and key financial metrics (like revenue and earnings per share) sourced from reliable financial databases. Crucially, the model also incorporates macroeconomic factors like interest rates, inflation, and industry-specific news sentiment. These indicators are standardized and weighted based on their historical correlation with ACHV's performance, ensuring a comprehensive and contextually relevant forecast. Regular backtesting and validation are critical elements of this model's development and deployment. The model will be refined and updated periodically using continuously collected data to improve accuracy over time.
Model training was conducted in multiple stages, beginning with data preprocessing and feature engineering. Data was cleaned, outliers were handled, and missing values were imputed using appropriate techniques. Feature selection was crucial, as we employed techniques like Recursive Feature Elimination (RFE) to determine the most informative predictors. Subsequently, the LSTM network was trained on a significant portion of the historical data to learn the underlying relationships within the data. Model performance was evaluated using metrics such as Mean Absolute Error (MAE) and Root Mean Squared Error (RMSE) on an independent test dataset. These metrics provide a concrete assessment of the model's accuracy in forecasting future price movements. Hyperparameter tuning was conducted using techniques like GridSearchCV to optimize the network's architecture for optimal performance. This ensures that the chosen network architecture is suitable for the specific characteristics of ACHV's stock movement. Furthermore, the model incorporates robust error handling mechanisms to mitigate potential issues arising from noisy or incomplete data.
The final model, post-training and validation, offers a probabilistic forecast of future ACHV stock prices. The output of the model can be presented as a confidence interval for future price ranges, allowing for a more nuanced understanding of the potential volatility and uncertainty. This approach allows investors to make informed decisions with a more comprehensive understanding of the predicted price behavior. We believe this model, combining sophisticated machine learning techniques with fundamental economic analysis, offers a powerful tool for forecasting ACHV stock performance. Further refinement and continuous monitoring of the model's performance against new data will be essential to maintain its accuracy and reliability over time. Transparency and traceability are embedded throughout the model's development and implementation process.
ML Model Testing
n:Time series to forecast
p:Price signals of Achieve Life Sciences stock
j:Nash equilibria (Neural Network)
k:Dominated move of Achieve Life Sciences stock holders
a:Best response for Achieve Life Sciences target price
For further technical information as per how our model work we invite you to visit the article below:
How do KappaSignal algorithms actually work?
Achieve Life Sciences Stock Forecast (Buy or Sell) Strategic Interaction Table
Strategic Interaction Table Legend:
X axis: *Likelihood% (The higher the percentage value, the more likely the event will occur.)
Y axis: *Potential Impact% (The higher the percentage value, the more likely the price will deviate.)
Z axis (Grey to Black): *Technical Analysis%
Achieve Life Sciences Inc. Financial Outlook and Forecast
Achieve Life Sciences' financial outlook hinges critically on the success of its drug development pipeline and the ability to secure necessary funding. The company's revenue model is predominantly derived from research and development collaborations, and grants, along with potential future product sales if their pipeline candidates reach the market. A key element impacting the financial forecast is the stage of development for each drug candidate. Early-stage drug candidates present significant uncertainties. Success hinges on successful completion of preclinical and clinical trials, securing regulatory approvals, and establishing market demand. Factors impacting this forecast include regulatory approvals for drug candidates, market acceptance, pricing strategies, and the overall economic climate. Significant R&D investments are expected, potentially impacting profitability in the short term, but success in bringing innovative and effective drugs to market could significantly enhance long-term financial performance.
Achieve Life Sciences' financial forecast will depend heavily on the outcomes of clinical trials for its current drug candidates. Positive results could lead to increased investor interest and funding opportunities, allowing the company to further expand its pipeline and research efforts. Conversely, negative or inconclusive results from clinical trials would negatively impact investor confidence and potentially hinder fundraising efforts. Maintaining consistent and transparent communication regarding trial progress and potential challenges is vital for investor confidence. The company's financial projections will need to incorporate various potential scenarios, from rapid and successful development to more prolonged and challenging processes. A robust financial model should also account for the potential for unexpected external factors like changing market needs or regulatory hurdles.
The competitive landscape in the pharmaceutical and biotechnology sectors is a significant factor influencing Achieve Life Sciences' financial outlook. The presence of well-established and larger pharmaceutical companies can make it challenging for a smaller company like Achieve Life Sciences to compete and secure market share. Competitors' development strategies and the availability of similar therapies directly impact the success and pricing potential of Achieve Life Sciences' own products. To counteract these pressures, the company must focus on differentiating its products through innovative approaches and novel mechanisms of action. This differentiation strategy will be crucial for establishing market acceptance and potentially achieving a competitive edge over established rivals. Intellectual property protection and strategic partnerships also play a crucial role in managing these competitive pressures.
Predicting the financial outlook for Achieve Life Sciences presents some inherent risks. While the successful development and commercialization of a promising drug candidate could lead to significant returns, there's no guarantee of such success. Clinical trials can be lengthy and costly, and regulatory approvals are not assured. A potential negative outlook could arise from factors including clinical trial failures, unexpected safety concerns, increased competition, or delays in securing necessary funding. The overall market sentiment and economic conditions also introduce risk. Conversely, positive market trends and robust financial performance of competitors could pose increased challenges for Achieve Life Sciences. This company's future prospects depend on numerous uncertain variables, and any projections must be viewed with a degree of caution. The potential rewards of a successful breakthrough in drug development must be balanced against the inherent risks and uncertainties in the pharmaceutical industry. Investors should carefully analyze these uncertainties before investing.
Rating | Short-Term | Long-Term Senior |
---|---|---|
Outlook | B1 | Ba2 |
Income Statement | B1 | Baa2 |
Balance Sheet | Ba3 | Baa2 |
Leverage Ratios | Baa2 | Caa2 |
Cash Flow | Caa2 | Baa2 |
Rates of Return and Profitability | Caa2 | C |
*Financial analysis is the process of evaluating a company's financial performance and position by neural network. It involves reviewing the company's financial statements, including the balance sheet, income statement, and cash flow statement, as well as other financial reports and documents.
How does neural network examine financial reports and understand financial state of the company?
References
- Pennington J, Socher R, Manning CD. 2014. GloVe: global vectors for word representation. In Proceedings of the 2014 Conference on Empirical Methods on Natural Language Processing, pp. 1532–43. New York: Assoc. Comput. Linguist.
- M. Puterman. Markov Decision Processes: Discrete Stochastic Dynamic Programming. Wiley, New York, 1994.
- S. J. Russell and P. Norvig. Artificial Intelligence: A Modern Approach. Prentice Hall, Englewood Cliffs, NJ, 3nd edition, 2010
- Banerjee, A., J. J. Dolado, J. W. Galbraith, D. F. Hendry (1993), Co-integration, Error-correction, and the Econometric Analysis of Non-stationary Data. Oxford: Oxford University Press.
- K. Boda, J. Filar, Y. Lin, and L. Spanjers. Stochastic target hitting time and the problem of early retirement. Automatic Control, IEEE Transactions on, 49(3):409–419, 2004
- Chernozhukov V, Newey W, Robins J. 2018c. Double/de-biased machine learning using regularized Riesz representers. arXiv:1802.08667 [stat.ML]
- Athey S, Mobius MM, Pál J. 2017c. The impact of aggregators on internet news consumption. Unpublished manuscript, Grad. School Bus., Stanford Univ., Stanford, CA