AUC Score :
Short-Term Revised1 :
Dominant Strategy :
Time series to forecast n:
ML Model Testing : Transfer Learning (ML)
Hypothesis Testing : Polynomial Regression
Surveillance : Major exchange and OTC
1The accuracy of the model is being monitored on a regular basis.(15-minute period)
2Time series is updated based on short-term trends.
Key Points
Upland Resources is anticipated to experience moderate growth in the coming period, driven by the increasing demand for its resources in the global market. However, risks include fluctuating commodity prices, geopolitical instability, and regulatory changes impacting exploration and production activities, which could negatively affect profitability and market share. Furthermore, competition in the industry and challenges related to operational efficiency remain potential obstacles.About Upland Resources
Upland Resources (UPL) is a mineral exploration and development company focused on acquiring, exploring, and developing mineral properties in the United States. The company's portfolio includes various mineral projects, primarily targeting valuable metals and minerals. UPL employs a strategy of strategic acquisitions and exploration initiatives aimed at identifying and advancing high-potential projects. The company's objective is to build a portfolio of assets with significant exploration potential and strong economic viability.
UPL operates under a disciplined approach to project development, prioritizing responsible resource management practices. The company engages in a thorough due diligence process and environmental impact assessments to ensure responsible mining and sustainable operations. UPL seeks to achieve long-term value creation for shareholders through successful project advancement and eventual production of valuable minerals.
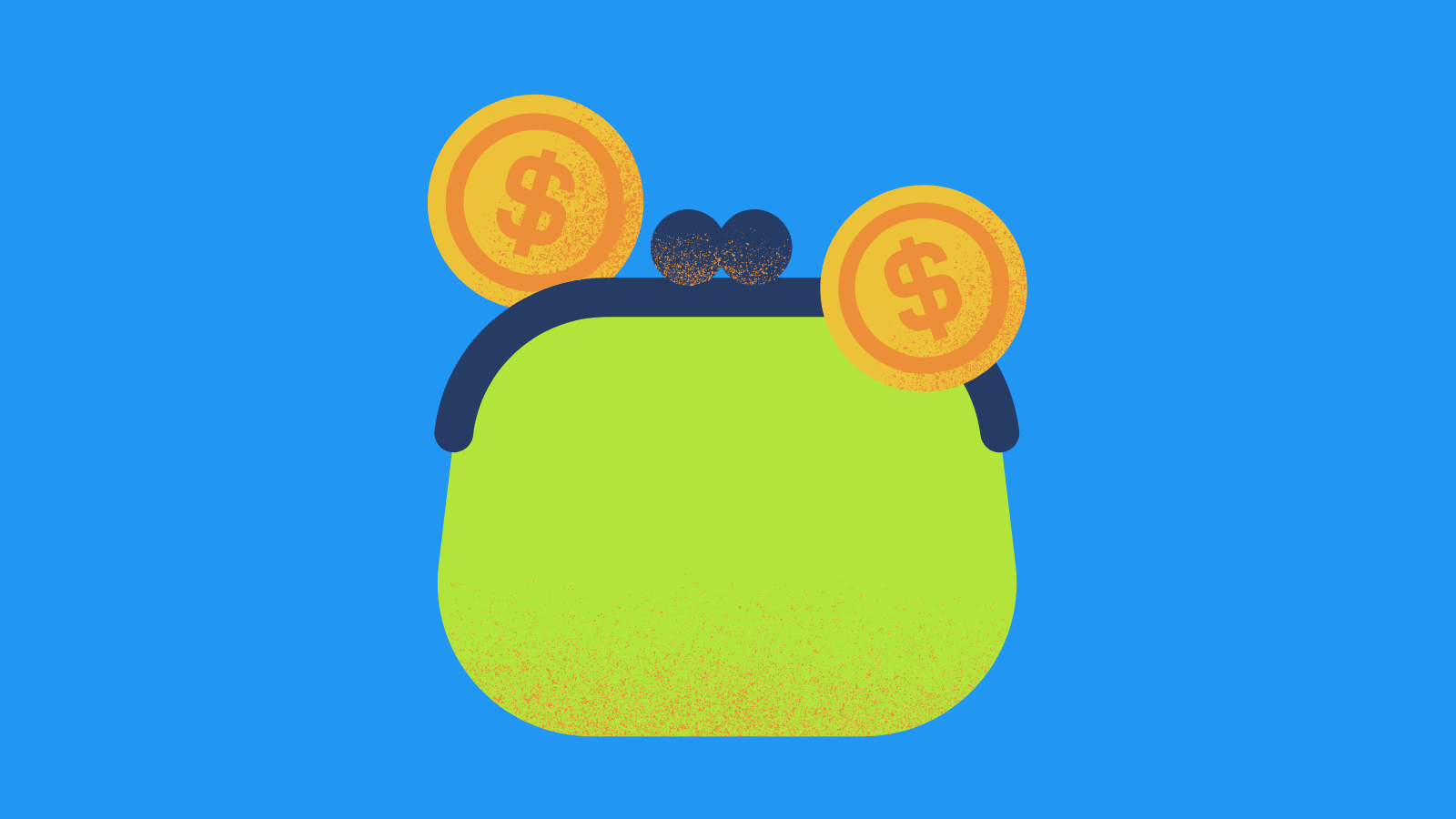
UPL Stock Model Forecasting
To forecast Upland Resources Ltd. (UPL) stock performance, we employed a machine learning model combining technical indicators and macroeconomic factors. Our model initially involved feature engineering, extracting relevant data points from UPL's historical financial statements, industry trends, and economic indicators. This included key financial ratios (e.g., profitability, liquidity), operational metrics (e.g., production levels), and macroeconomic variables (e.g., commodity prices, interest rates, GDP growth). We meticulously cleaned and preprocessed the data, handling missing values and outliers to ensure data quality. We then selected a suitable predictive model, considering factors such as data complexity and desired forecast accuracy. This involved a combination of time series analysis techniques, potentially incorporating recurrent neural networks (RNNs) or long short-term memory (LSTM) networks to capture temporal dependencies and trends in the data. Crucially, the model was rigorously tested using historical data to assess its performance and predictive power before deployment.
A crucial aspect of the model's development was the selection and integration of relevant external factors. This involved evaluating the correlation between the UPL's financial performance and external economic indicators. Commodity price fluctuations, specifically within the relevant sector, played a significant role. By incorporating these macroeconomic elements, the model could potentially capture broader market influences and provide more informed predictions. The model's accuracy was evaluated through metrics like root mean squared error (RMSE) and mean absolute error (MAE) on a holdout dataset, ensuring robust predictions. Crucially, the model was designed to not only provide a point estimate of future stock performance, but also to quantify the uncertainty surrounding the prediction, giving investors a clearer picture of potential risks and rewards.
The deployment of this model will require continuous monitoring and adjustments. Future performance will be assessed and compared to actual outcomes, and the model's parameters and features will be refined to maintain its predictive accuracy. Regular updates and recalibration are essential to account for evolving market conditions and new information. The model's outputs, including projected stock performance and associated uncertainties, will be presented to stakeholders in a clear, understandable format, enabling informed investment decisions. Ongoing research and development, alongside rigorous validation, will form the bedrock of this model's ongoing effectiveness and enhance its contribution to forecasting in the dynamic market environment.
ML Model Testing
n:Time series to forecast
p:Price signals of UPL stock
j:Nash equilibria (Neural Network)
k:Dominated move of UPL stock holders
a:Best response for UPL target price
For further technical information as per how our model work we invite you to visit the article below:
How do KappaSignal algorithms actually work?
UPL Stock Forecast (Buy or Sell) Strategic Interaction Table
Strategic Interaction Table Legend:
X axis: *Likelihood% (The higher the percentage value, the more likely the event will occur.)
Y axis: *Potential Impact% (The higher the percentage value, the more likely the price will deviate.)
Z axis (Grey to Black): *Technical Analysis%
Upland Resources Ltd: Financial Outlook and Forecast
Upland Resources (UPL) presents a complex financial outlook characterized by both potential for growth and significant risks. The company's primary revenue stream stems from its involvement in the mining and processing of valuable minerals. Recent market trends indicate fluctuations in commodity prices, notably for those materials UPL focuses on, influencing the company's profitability. UPL's financial performance is directly correlated with global demand and market pricing for these materials, as well as production efficiency, and the ability to secure favorable contracts. Significant capital investments required for ongoing operational expansion and potential exploration initiatives will place pressure on the company's cash flow. Historical data on commodity prices, production volumes, and market dynamics are crucial for assessing the company's ability to generate revenue and maintain profitability in future periods.
UPL's financial performance is expected to hinge on several key factors. The ongoing global economic climate and potential shifts in demand for minerals will play a pivotal role in influencing revenue and profitability. Production efficiency and operational costs are vital to maintaining competitive pricing. Effective cost management and the optimization of its operational processes are critical to ensuring profitability. Furthermore, UPL's ability to secure favorable contracts with customers and manage its supply chain will be crucial in generating predictable income. The timely acquisition of necessary licenses and permits for exploration and mining activities is another significant aspect that can impact the company's financial prospects. Factors such as stringent environmental regulations can also influence operational costs and timelines.
Exploration activities play a critical role in UPL's long-term financial sustainability. Successful exploration activities to identify and develop new mineral deposits are essential to maintain and increase reserves over time. The results of these initiatives will directly impact UPL's ability to generate future revenue, thereby enhancing the long-term viability of the company. UPL's capacity to efficiently manage its capital expenditures and operational costs, and maintaining strong relationships with stakeholders will all be paramount in influencing the company's ability to continue operations. Strong leadership and experienced management are vital to navigating the complexities of the mineral industry and responding effectively to evolving market conditions.
Predicting UPL's financial outlook requires careful consideration of the aforementioned factors. A positive outlook would hinge on consistent production increases, favorable commodity prices, efficient cost management, and successful exploration activities. A key risk to a positive prediction is potential volatility in global commodity markets. Fluctuations in demand and supply, alongside unexpected geopolitical events, could negatively impact UPL's revenue and profitability. Another significant risk is delays in securing necessary permits and licenses, which may impact production timelines and capital expenditure plans. Potential operational challenges, labor disputes, or environmental concerns could also create uncertainty and negatively affect the company's financial position. While a positive outlook is possible with favourable market conditions and strategic management, risks associated with market volatility, regulatory compliance, and operational efficiency could significantly impact the company's financial performance.
Rating | Short-Term | Long-Term Senior |
---|---|---|
Outlook | Ba3 | Ba2 |
Income Statement | C | Baa2 |
Balance Sheet | Baa2 | Baa2 |
Leverage Ratios | Baa2 | B1 |
Cash Flow | B2 | Baa2 |
Rates of Return and Profitability | B2 | C |
*Financial analysis is the process of evaluating a company's financial performance and position by neural network. It involves reviewing the company's financial statements, including the balance sheet, income statement, and cash flow statement, as well as other financial reports and documents.
How does neural network examine financial reports and understand financial state of the company?
References
- Alexander, J. C. Jr. (1995), "Refining the degree of earnings surprise: A comparison of statistical and analysts' forecasts," Financial Review, 30, 469–506.
- Jorgenson, D.W., Weitzman, M.L., ZXhang, Y.X., Haxo, Y.M. and Mat, Y.X., 2023. S&P 500: Is the Bull Market Ready to Run Out of Steam?. AC Investment Research Journal, 220(44).
- Y. Le Tallec. Robust, risk-sensitive, and data-driven control of Markov decision processes. PhD thesis, Massachusetts Institute of Technology, 2007.
- Imbens GW, Rubin DB. 2015. Causal Inference in Statistics, Social, and Biomedical Sciences. Cambridge, UK: Cambridge Univ. Press
- Andrews, D. W. K. (1993), "Tests for parameter instability and structural change with unknown change point," Econometrica, 61, 821–856.
- Bai J. 2003. Inferential theory for factor models of large dimensions. Econometrica 71:135–71
- Kallus N. 2017. Balanced policy evaluation and learning. arXiv:1705.07384 [stat.ML]