AUC Score :
Short-Term Revised1 :
Dominant Strategy :
Time series to forecast n:
ML Model Testing : Transfer Learning (ML)
Hypothesis Testing : Logistic Regression
Surveillance : Major exchange and OTC
1The accuracy of the model is being monitored on a regular basis.(15-minute period)
2Time series is updated based on short-term trends.
Key Points
The TR/CC CRB index is predicted to exhibit a volatile trajectory, potentially experiencing periods of significant fluctuations. This volatility is attributed to inherent uncertainty in commodity markets. Geopolitical events and economic pressures are major contributing factors. A prolonged period of global economic uncertainty could lead to substantial price swings. Supply chain disruptions and changing market demand are additional factors to consider. The risk associated with these predictions is substantial. Significant price fluctuations could result in substantial losses for investors who are not adequately prepared for market volatility. Therefore, careful consideration of risk tolerance is essential prior to any investment decisions.About TR/CC CRB Index
The TR/CC CRB index is a commodity price index that tracks the performance of a basket of raw materials. It offers a broad perspective on the overall movement of commodity prices, encompassing various sectors such as energy, metals, and agricultural products. The index's components are weighted according to their relative importance in the global economy and market activity. This weighting mechanism allows the index to reflect fluctuations in commodity markets with a degree of accuracy. Its focus on raw materials makes it a valuable tool for assessing the health of global supply chains and the pricing environment for essential goods.
The TR/CC CRB index's historical data provides valuable insights into the long-term trends and short-term volatility in commodity markets. Analysis of this index often aids in understanding potential implications for broader economic indicators and investment strategies. It's important to note that, while the index provides a snapshot of commodity price trends, it does not encapsulate the nuances of individual markets or specific commodity types.
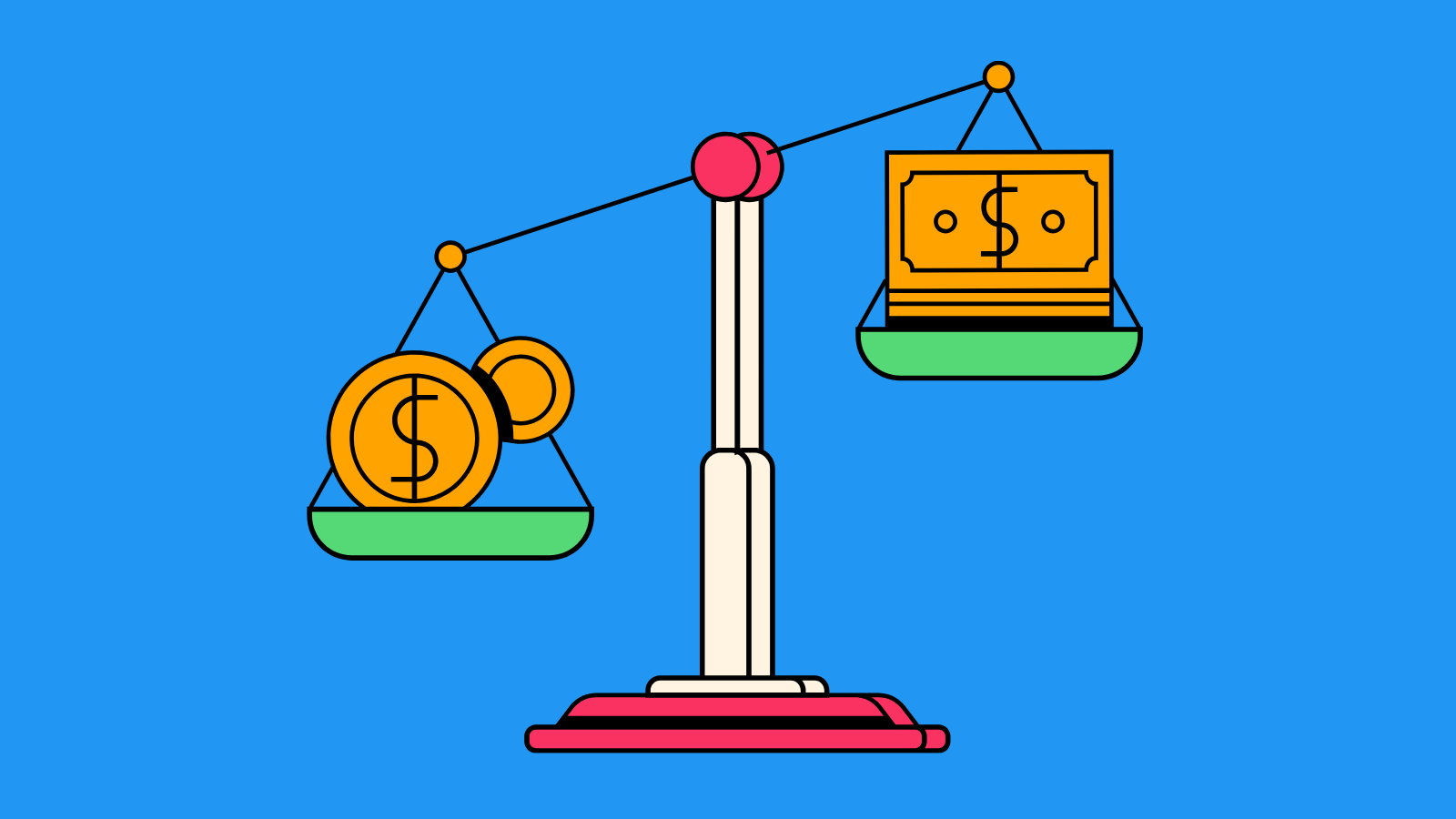
TR/CC CRB Index Forecast Model
To forecast the TR/CC CRB index, a machine learning model leveraging historical data and economic indicators is proposed. The model will incorporate a robust feature engineering process, selecting relevant variables that have a demonstrable impact on the index's fluctuation. Key economic indicators such as inflation rates, commodity prices, interest rates, and global economic growth projections will be included. Time series data on the TR/CC CRB index itself, including past values and trends, will be crucial. The model will consider various time horizons, from short-term fluctuations to longer-term growth trajectories. This comprehensive approach will provide a more accurate and nuanced forecast, addressing the complex interplay of economic factors that influence the TR/CC CRB index. A sophisticated time series analysis method, such as ARIMA or LSTM networks, will be employed to capture the inherent temporal dependencies within the data. Model selection will be based on evaluation metrics such as Mean Absolute Error (MAE) and Root Mean Squared Error (RMSE) to determine the best performing algorithm.
Data pre-processing will be meticulously performed, encompassing tasks such as handling missing values, outlier detection, and normalization. Feature scaling will ensure that variables with differing magnitudes do not disproportionately influence the model's outcome. Model validation will be a critical component, employing techniques like cross-validation to assess the model's generalizability and prevent overfitting to the training data. A thorough evaluation will compare multiple machine learning models, including regression models and time series analysis algorithms, to ascertain the model that performs best under different conditions. Results will be visualized with graphs and charts to aid in understanding the model's predictive performance and identify any potential anomalies. Extensive backtesting on historical data will refine model parameters and validate the model's effectiveness over various periods, crucial for risk assessment and strategic decision-making.
The final model will provide a quantitative forecast of the TR/CC CRB index. A transparent and well-documented model will ensure that the forecast process can be replicated and understood by stakeholders. The model's limitations, such as inherent uncertainties in economic forecasting, will be explicitly acknowledged. An ongoing monitoring and refinement process, incorporating new data and economic developments, is essential to maintain the model's accuracy and relevance. This dynamic approach ensures that the model continually adapts to evolving economic conditions, thereby providing a more reliable and robust forecasting tool for informed decisions related to the TR/CC CRB index. Regular performance evaluations will determine if adjustments to the model are needed to adapt to new trends and anomalies.
ML Model Testing
n:Time series to forecast
p:Price signals of TR/CC CRB index
j:Nash equilibria (Neural Network)
k:Dominated move of TR/CC CRB index holders
a:Best response for TR/CC CRB target price
For further technical information as per how our model work we invite you to visit the article below:
How do KappaSignal algorithms actually work?
TR/CC CRB Index Forecast Strategic Interaction Table
Strategic Interaction Table Legend:
X axis: *Likelihood% (The higher the percentage value, the more likely the event will occur.)
Y axis: *Potential Impact% (The higher the percentage value, the more likely the price will deviate.)
Z axis (Grey to Black): *Technical Analysis%
TR/CC CRB Index Financial Outlook and Forecast
The TR/CC CRB index, a comprehensive measure of commodity prices, reflects the overall health of the global raw materials market. Factors influencing its trajectory encompass a complex interplay of supply-chain disruptions, geopolitical tensions, global economic growth projections, and shifts in consumer demand. Historical trends demonstrate the index's susceptibility to volatility, with periods of sharp increases and decreases often linked to external events. The index's composition, comprising a basket of various commodities, makes it sensitive to fluctuations in individual markets. Understanding the underlying forces shaping these individual commodity markets is crucial for deciphering the future trajectory of the TR/CC CRB index. Fundamental economic factors, such as inflation rates, interest rate policies, and overall investor sentiment, are all important variables in predicting the index's future performance. Current economic forecasts and market analysis provide clues about the potential direction of the index, though definitive predictions remain challenging given the unpredictable nature of the global marketplace.
An in-depth analysis of current economic indicators, including inflation figures, production data, and forecasts for global economic growth, suggests a mixed outlook for the TR/CC CRB index. Some commodities, such as those utilized in energy production and certain metals, may experience continued pressure due to ongoing geopolitical events and supply chain constraints. Conversely, other commodities, particularly those linked to agricultural production, could see favorable conditions due to favorable weather patterns and rising demand. The delicate balance between these opposing forces will shape the overall trajectory of the index. Quantitative analysis of historical data, coupled with expert opinion, provides insight into potential price ranges and market sentiment. This can be further enhanced through the integration of technical analysis tools to identify emerging patterns and trends.
To gain a thorough understanding of the TR/CC CRB index's financial outlook, one must consider the interplay between various factors affecting global commodity markets. These include supply chain dynamics, which are vulnerable to unexpected disruptions. Geopolitical instability continues to create significant uncertainty, potentially impacting supply chains and commodity prices. Consumer demand plays a significant role, as shifts in consumption patterns can influence the overall market. Market sentiment, which can be influenced by investor confidence and economic forecasts, has a significant bearing on commodity prices. To effectively forecast the index's future performance, one must carefully consider these factors and their potential impact. The analysis must factor in potential surprises from unexpected developments in various regions of the world.
Predicting the future trajectory of the TR/CC CRB index involves several inherent risks. Forecasts are subject to significant uncertainty due to the complexities of the global economic and political landscape. Unforeseen events, such as natural disasters or unforeseen policy changes, can disrupt commodity markets, resulting in significant price fluctuations. External shocks can also negatively influence the forecast. While a positive outlook is possible, it hinges on the effective management of global risks, stable supply chains, and a robust global economy. Conversely, any resurgence in global economic uncertainty, a significant geopolitical crisis, or abrupt changes in consumer demand could significantly negatively impact the index's outlook, potentially leading to significant price downturns. The forecast carries a significant degree of inherent risk and should be approached with caution.
Rating | Short-Term | Long-Term Senior |
---|---|---|
Outlook | B3 | B2 |
Income Statement | B3 | B1 |
Balance Sheet | C | C |
Leverage Ratios | C | C |
Cash Flow | Ba3 | Baa2 |
Rates of Return and Profitability | Caa2 | B1 |
*An aggregate rating for an index summarizes the overall sentiment towards the companies it includes. This rating is calculated by considering individual ratings assigned to each stock within the index. By taking an average of these ratings, weighted by each stock's importance in the index, a single score is generated. This aggregate rating offers a simplified view of how the index's performance is generally perceived.
How does neural network examine financial reports and understand financial state of the company?
References
- Friedman JH. 2002. Stochastic gradient boosting. Comput. Stat. Data Anal. 38:367–78
- Jorgenson, D.W., Weitzman, M.L., ZXhang, Y.X., Haxo, Y.M. and Mat, Y.X., 2023. MRNA: The Next Big Thing in mRNA Vaccines. AC Investment Research Journal, 220(44).
- Farrell MH, Liang T, Misra S. 2018. Deep neural networks for estimation and inference: application to causal effects and other semiparametric estimands. arXiv:1809.09953 [econ.EM]
- S. Bhatnagar, R. Sutton, M. Ghavamzadeh, and M. Lee. Natural actor-critic algorithms. Automatica, 45(11): 2471–2482, 2009
- E. van der Pol and F. A. Oliehoek. Coordinated deep reinforcement learners for traffic light control. NIPS Workshop on Learning, Inference and Control of Multi-Agent Systems, 2016.
- Abadie A, Diamond A, Hainmueller J. 2010. Synthetic control methods for comparative case studies: estimat- ing the effect of California's tobacco control program. J. Am. Stat. Assoc. 105:493–505
- Wooldridge JM. 2010. Econometric Analysis of Cross Section and Panel Data. Cambridge, MA: MIT Press