AUC Score :
Short-Term Revised1 :
Dominant Strategy :
Time series to forecast n:
ML Model Testing : Ensemble Learning (ML)
Hypothesis Testing : Beta
Surveillance : Major exchange and OTC
1The accuracy of the model is being monitored on a regular basis.(15-minute period)
2Time series is updated based on short-term trends.
Key Points
The TR/CC CRB Aluminum index is anticipated to exhibit moderate volatility in the coming period. Factors influencing price fluctuations include global economic conditions, raw material supply chain disruptions, and shifts in industrial demand. A potential increase in demand from emerging economies could drive prices upward, though this is contingent upon sustained economic growth and the availability of necessary inputs. Conversely, a slowdown in global manufacturing or increased supply could pressure prices downwards. The risk associated with these predictions is substantial. Significant unforeseen geopolitical events or unexpected technological advancements could drastically alter market trends, rendering predictions unreliable. Therefore, a cautious investment strategy is recommended, considering the inherent complexities and uncertainties embedded within the aluminum market.About TR/CC CRB Aluminum Index
The TR/CC CRB Aluminum index is a benchmark for the trading of aluminum, reflecting the market price of this commodity. It tracks the changes in the spot and futures prices of aluminum, offering a comprehensive view of market sentiment and supply-demand dynamics. The index's composition includes a variety of aluminum sources and trading venues, ensuring a representative picture of the global aluminum market. This index is crucial for investors, traders, and analysts to evaluate the aluminum market's performance and anticipate future trends.
The index's constituents, calculated through complex methodology, represent various grades and forms of aluminum, reflecting their relative market values. Historical data and ongoing updates are instrumental in identifying patterns and making informed investment decisions within the broader commodity market. This comprehensive dataset about aluminum prices provides insights into the commodity's economic impact and helps predict future price fluctuations. A core element of the index is its ability to provide a reliable and transparent assessment of the state of the aluminum market.
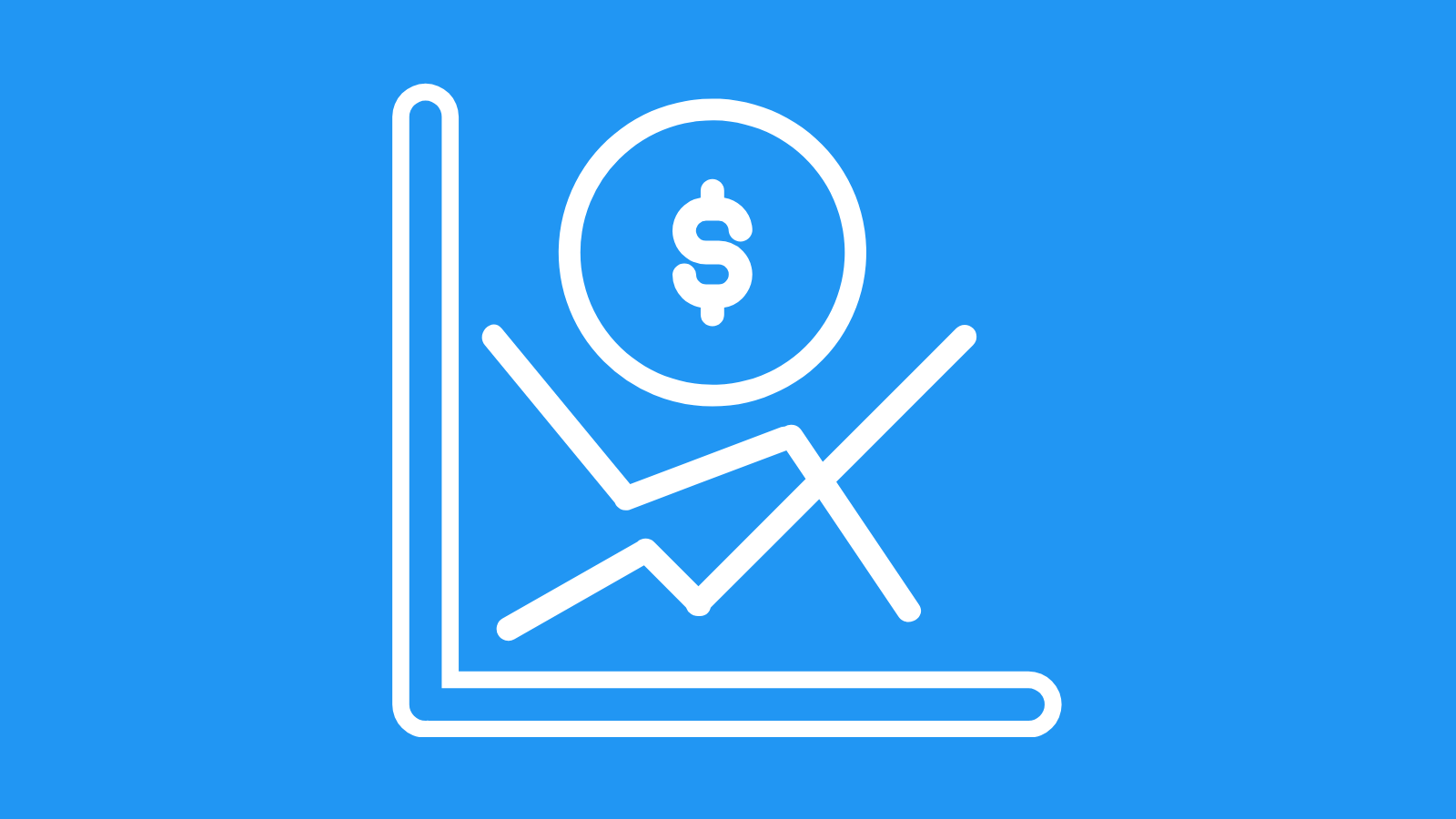
TR/CC CRB Aluminum Index Forecast Model
This model for forecasting the TR/CC CRB Aluminum index leverages a combination of time series analysis and machine learning techniques. A comprehensive dataset is crucial, incorporating historical price data for the TR/CC CRB Aluminum index, along with relevant macroeconomic indicators such as global aluminum demand, supply chain disruptions, geopolitical events, and prices of competing raw materials (like copper and zinc). Data preprocessing is paramount, involving handling missing values, outlier detection, and normalization to ensure data quality and consistency. Time series decomposition methods, like the decomposition of a time series into trend, seasonality, and residual components, will be employed to understand historical patterns. Subsequently, a machine learning model, such as a recurrent neural network (RNN) or a long short-term memory (LSTM) network, will be trained on this preprocessed data to capture complex temporal dependencies and predict future index values. These models are particularly well-suited to capturing non-linear relationships and handling volatility intrinsic to commodity markets. Crucially, feature engineering plays a critical role in improving predictive accuracy. This process involves creating new features based on existing ones, incorporating insights from economic and market analysis. For example, lagged variables and moving averages can be included to better capture the dynamic nature of market trends.
Model evaluation is performed using rigorous statistical methods to assess predictive accuracy and robustness. Cross-validation techniques will be employed to estimate the model's performance on unseen data. Metrics like mean absolute error (MAE), root mean squared error (RMSE), and R-squared will be utilized to quantitatively evaluate the model's predictive ability. A comparison with simpler forecasting methods such as autoregressive integrated moving average (ARIMA) models will be conducted to provide a comparative analysis of the model's performance. Moreover, this model will not be deployed without sensitivity analysis, exploring how the model's predictions change in response to varying inputs or feature engineering adjustments. Uncertainty quantification will also be a part of the model development process, allowing us to assess the confidence intervals around our forecasts, acknowledging the inherent uncertainty of commodity market predictions.
The model will be regularly updated and retrained using new data to maintain its predictive accuracy. This iterative approach is essential for adapting to changing market conditions. Monitoring the model's performance will be a critical element in the overall strategy, and any anomalies or unexpected results will trigger further investigation and model refinement. By incorporating various economic factors in the feature set and implementing robust statistical evaluation methods, the model's outputs will provide informed guidance for decision-making in the TR/CC CRB Aluminum market, reflecting a sophisticated understanding of market dynamics. Furthermore, the transparency of the model's structure and the documentation of the model's assumptions and limitations are crucial for building trust and facilitating informed interpretation of the forecasts.
ML Model Testing
n:Time series to forecast
p:Price signals of TR/CC CRB Aluminum index
j:Nash equilibria (Neural Network)
k:Dominated move of TR/CC CRB Aluminum index holders
a:Best response for TR/CC CRB Aluminum target price
For further technical information as per how our model work we invite you to visit the article below:
How do KappaSignal algorithms actually work?
TR/CC CRB Aluminum Index Forecast Strategic Interaction Table
Strategic Interaction Table Legend:
X axis: *Likelihood% (The higher the percentage value, the more likely the event will occur.)
Y axis: *Potential Impact% (The higher the percentage value, the more likely the price will deviate.)
Z axis (Grey to Black): *Technical Analysis%
TR/CC CRB Aluminum Index Financial Outlook and Forecast
The TR/CC CRB Aluminum index, a benchmark for the global aluminum market, reflects the fluctuating prices of this vital industrial metal. Factors influencing its trajectory are multifaceted, encompassing global economic conditions, industrial production levels, and geopolitical events. Supply and demand dynamics play a critical role, particularly given aluminum's extensive use in diverse sectors like transportation, construction, and consumer goods. Fluctuations in raw material costs, such as energy prices, and the availability of refining capacity also significantly impact the index. The ongoing transition towards renewable energy sources and its potential impact on aluminum demand are emerging considerations in long-term forecasting, as well as the sustainability practices implemented by aluminum producers.
A detailed examination of the current economic environment reveals a mixed bag of potential drivers. A robust global economy often translates to increased demand for industrial metals like aluminum, boosting the index. Conversely, economic slowdowns or recessions can depress demand and, consequently, aluminum prices. Inflationary pressures, if sustained, can exert upward pressure on aluminum prices as input costs rise, while interest rate increases may temper demand. The ongoing geopolitical landscape, including trade tensions and conflicts, can disrupt supply chains and influence the pricing of commodities like aluminum, introducing uncertainty into market forecasting.
Looking ahead, the aluminum market faces a complex interplay of influences. Analysts expect moderate growth in demand in the coming years, driven by robust construction activities and ongoing expansion in certain industrial sectors. However, the exact pace and scale of this growth remain uncertain. Technological advancements and the development of substitute materials could also pose a long-term threat to demand for aluminum, although existing aluminum production and consumption infrastructure presents a significant barrier. Furthermore, the sustainability initiatives within the aluminum industry, alongside the escalating focus on carbon reduction, could steer the market toward more environmentally friendly production methods, influencing prices and the index trajectory.
Predicting the index's future trajectory presents a challenge. While moderate growth in demand is anticipated, several risks could disrupt this outlook. A sharp economic downturn could lead to a significant contraction in aluminum demand and a corresponding price decline. Geopolitical instability and disruptions to supply chains could severely impact the availability of raw materials, driving up prices or creating shortages. Technological advancements and the rise of substitute materials could challenge aluminum's dominance in certain sectors. Conversely, a sustained increase in demand and infrastructure investments could support prices and the index. Therefore, the anticipated moderate growth outlook carries a significant degree of risk, contingent on the resolution of these uncertainties and unforeseen future events. The long-term stability of the TR/CC CRB Aluminum index depends heavily on global economic conditions, geopolitical factors, and the evolution of technological advancements.
Rating | Short-Term | Long-Term Senior |
---|---|---|
Outlook | Caa2 | B1 |
Income Statement | C | B1 |
Balance Sheet | Ba1 | B2 |
Leverage Ratios | C | Ba3 |
Cash Flow | C | B3 |
Rates of Return and Profitability | B2 | B2 |
*An aggregate rating for an index summarizes the overall sentiment towards the companies it includes. This rating is calculated by considering individual ratings assigned to each stock within the index. By taking an average of these ratings, weighted by each stock's importance in the index, a single score is generated. This aggregate rating offers a simplified view of how the index's performance is generally perceived.
How does neural network examine financial reports and understand financial state of the company?
References
- M. Ono, M. Pavone, Y. Kuwata, and J. Balaram. Chance-constrained dynamic programming with application to risk-aware robotic space exploration. Autonomous Robots, 39(4):555–571, 2015
- Schapire RE, Freund Y. 2012. Boosting: Foundations and Algorithms. Cambridge, MA: MIT Press
- D. S. Bernstein, S. Zilberstein, and N. Immerman. The complexity of decentralized control of Markov Decision Processes. In UAI '00: Proceedings of the 16th Conference in Uncertainty in Artificial Intelligence, Stanford University, Stanford, California, USA, June 30 - July 3, 2000, pages 32–37, 2000.
- Rumelhart DE, Hinton GE, Williams RJ. 1986. Learning representations by back-propagating errors. Nature 323:533–36
- Vapnik V. 2013. The Nature of Statistical Learning Theory. Berlin: Springer
- Dietterich TG. 2000. Ensemble methods in machine learning. In Multiple Classifier Systems: First International Workshop, Cagliari, Italy, June 21–23, pp. 1–15. Berlin: Springer
- Imbens GW, Rubin DB. 2015. Causal Inference in Statistics, Social, and Biomedical Sciences. Cambridge, UK: Cambridge Univ. Press