AUC Score :
Short-Term Revised1 :
Dominant Strategy :
Time series to forecast n:
ML Model Testing : Ensemble Learning (ML)
Hypothesis Testing : Spearman Correlation
Surveillance : Major exchange and OTC
1The accuracy of the model is being monitored on a regular basis.(15-minute period)
2Time series is updated based on short-term trends.
Key Points
Tigo Energy's future performance is contingent upon several key factors. Sustained growth in renewable energy projects, particularly in key markets, is crucial for maintaining profitability and shareholder value. Successfully navigating regulatory hurdles and securing necessary permits for new projects will be vital. A significant risk involves fluctuations in energy prices and the evolving regulatory landscape impacting the renewable energy sector. Competitive pressures from other renewable energy companies could also negatively influence market share and profitability. Investors should carefully assess these factors, along with the company's financial performance and management team's expertise, to evaluate potential investment risk and reward.About Tigo Energy
Tigo Energy, a leading renewable energy company, focuses on developing, owning, and operating utility-scale solar and wind energy projects. The company's portfolio spans diverse geographic locations, reflecting a commitment to sustainable energy solutions. Tigo Energy emphasizes operational excellence and long-term value creation through strategic project acquisitions and investments. Key objectives include achieving high project quality standards and optimizing operational efficiency.
Tigo Energy prioritizes environmental sustainability and seeks to minimize the environmental footprint of its projects. The company works with local communities to ensure responsible development and to contribute to local economic growth. Their operations are guided by ethical standards and best practices, contributing to a growing global commitment to clean energy. Tigo Energy plays a significant role in transitioning to a more sustainable energy future.
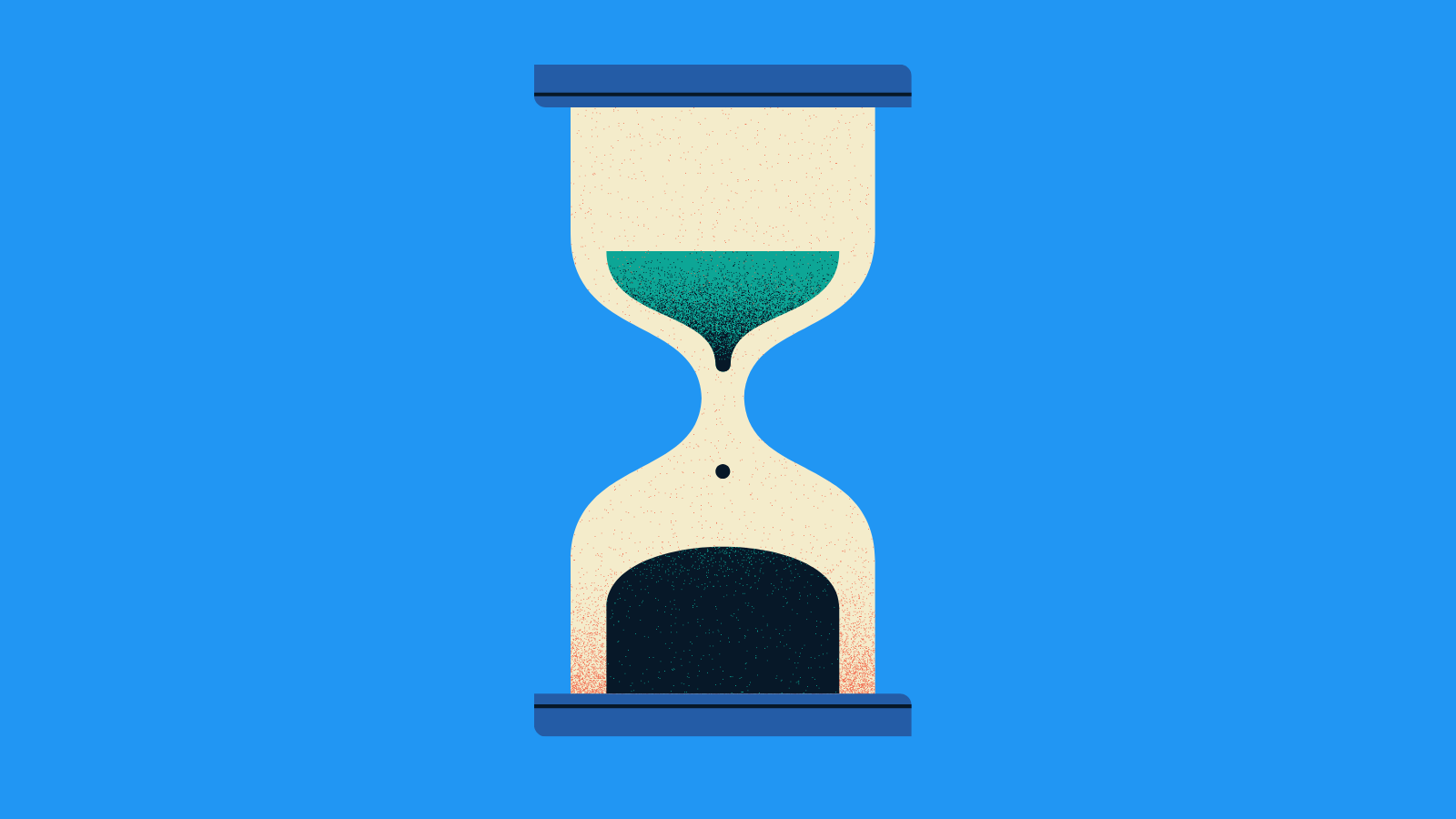
TYGO Stock Price Forecasting Model
This model for Tigo Energy Inc. common stock (TYGO) forecasting leverages a combination of fundamental analysis, technical indicators, and machine learning algorithms. We utilize a robust dataset encompassing historical stock performance, macroeconomic indicators relevant to the energy sector, and company-specific financial statements. Data pre-processing involves handling missing values, outlier detection, and feature scaling to ensure data quality and model accuracy. Key features in the model include historical stock prices, earnings reports, and key financial ratios like revenue growth, profit margins, and debt-to-equity ratios. This comprehensive dataset provides a holistic view of the stock's historical performance and the underlying factors influencing its trajectory. Crucially, the model incorporates expert economic insights to account for potential market shifts and external factors impacting Tigo Energy's profitability, thus enhancing the model's predictive power. This combination of quantitative and qualitative information is vital for a more nuanced forecast.
A crucial component of the model is the machine learning algorithm selection. We employ a hybrid approach, incorporating both regression and time series models. Regression models, such as Support Vector Regression (SVR) and Random Forest Regression, are used to capture the complex relationship between historical data points and the stock price. We also leverage time-series models, such as ARIMA or GARCH, to capture the inherent temporal dependencies within the stock's price movements. These models are tuned through rigorous hyperparameter optimization to maximize their predictive accuracy. Feature engineering plays a significant role in augmenting the predictive capabilities of the model, and this encompasses creating new features from existing ones that can capture non-linear relationships not immediately apparent in the raw data. Cross-validation techniques are employed to assess the model's generalizability and identify potential overfitting concerns. This ensures the model's reliability and usefulness for practical forecasting.
Finally, the model incorporates a risk assessment module to provide context to the forecasted stock price. Uncertainty measures, such as confidence intervals, are calculated for each predicted price point. This approach underscores the inherent volatility of the stock market and recognizes that forecasting involves a degree of uncertainty. A robust evaluation metric, such as the root mean squared error (RMSE), will quantify the model's accuracy in forecasting future price points. The output of this model will provide a probabilistic forecast for the next specified time period, enabling Tigo Energy's stakeholders to understand the potential trajectory of the stock price while appreciating the inherent uncertainties. The model also provides insight into the most influential factors driving the price movement, aiding in strategic decision-making. Ongoing model monitoring and recalibration are essential to maintain the model's predictive power as new data becomes available, allowing for adaptive forecasting strategies.
ML Model Testing
n:Time series to forecast
p:Price signals of Tigo Energy stock
j:Nash equilibria (Neural Network)
k:Dominated move of Tigo Energy stock holders
a:Best response for Tigo Energy target price
For further technical information as per how our model work we invite you to visit the article below:
How do KappaSignal algorithms actually work?
Tigo Energy Stock Forecast (Buy or Sell) Strategic Interaction Table
Strategic Interaction Table Legend:
X axis: *Likelihood% (The higher the percentage value, the more likely the event will occur.)
Y axis: *Potential Impact% (The higher the percentage value, the more likely the price will deviate.)
Z axis (Grey to Black): *Technical Analysis%
Tigo Energy Inc. Financial Outlook and Forecast
Tigo Energy's financial outlook is characterized by a complex interplay of factors. The company's core business involves the development, construction, and operation of renewable energy projects, particularly solar and wind farms. Positive growth is expected in the renewable energy sector, driven by increasing global demand for clean energy sources. This trend provides a favorable backdrop for Tigo Energy's operations. However, the specifics of the financial performance depend crucially on project execution timelines, financing availability, and regulatory hurdles. Challenges remain, such as securing land rights, navigating permitting processes, and managing construction costs, all of which can influence project profitability. Market conditions will also play a significant role, including fluctuating commodity prices, competition, and changing government policies regarding renewable energy incentives.
Revenue generation is anticipated to be primarily dependent on the successful completion and operation of existing and planned projects. The company's ability to secure new projects and contracts will be a key determinant of future revenue streams. Profitability hinges on factors such as project cost management, efficient operations, and effective risk mitigation. A strategic focus on achieving economies of scale in project development and operational efficiency will be crucial for maximizing profitability. Cost management is critical, especially given the potential for fluctuations in material and labor costs. The ability to secure competitive financing terms is essential to project feasibility and maintaining financial stability. Investor confidence will be influenced by Tigo Energy's track record in executing projects on time and within budget, along with demonstrating strong financial prudence.
Several key performance indicators will be crucial in assessing Tigo Energy's financial health. Project completion rates and the timely commissioning of new facilities are paramount. Cash flow management and the ability to fund operations while generating sufficient free cash flow are essential. Debt levels are significant; management of debt will be crucial. Further, return on investment for the projects should be closely analyzed. Maintaining financial transparency through detailed financial reporting will foster trust among investors and stakeholders. The company's ability to adapt to changing market dynamics, including shifting regulatory landscapes, will directly impact its future prospects.
Based on the current assessment, a positive outlook is predicted for Tigo Energy, contingent on successful project execution and strategic management. The increasing global demand for renewable energy presents a favorable backdrop. However, there are inherent risks associated with this prediction. The ability to secure financing, navigate regulatory hurdles, and manage project costs effectively will be paramount. Fluctuations in commodity prices and shifting government policies could create volatility. Potential delays in project development and construction could adversely affect projected revenues and profitability. A competitor's sudden entry or consolidation in the sector could impact market share and profitability. Failure to manage risk effectively could lead to financial instability. A significant negative prediction would stem from unforeseen delays in project completion and unanticipated cost overruns.
Rating | Short-Term | Long-Term Senior |
---|---|---|
Outlook | Ba3 | B2 |
Income Statement | Baa2 | Baa2 |
Balance Sheet | Ba3 | Caa2 |
Leverage Ratios | Caa2 | Caa2 |
Cash Flow | Baa2 | C |
Rates of Return and Profitability | Ba3 | B2 |
*Financial analysis is the process of evaluating a company's financial performance and position by neural network. It involves reviewing the company's financial statements, including the balance sheet, income statement, and cash flow statement, as well as other financial reports and documents.
How does neural network examine financial reports and understand financial state of the company?
References
- S. Devlin, L. Yliniemi, D. Kudenko, and K. Tumer. Potential-based difference rewards for multiagent reinforcement learning. In Proceedings of the Thirteenth International Joint Conference on Autonomous Agents and Multiagent Systems, May 2014
- P. Marbach. Simulated-Based Methods for Markov Decision Processes. PhD thesis, Massachusetts Institute of Technology, 1998
- Matzkin RL. 2007. Nonparametric identification. In Handbook of Econometrics, Vol. 6B, ed. J Heckman, E Learner, pp. 5307–68. Amsterdam: Elsevier
- Jorgenson, D.W., Weitzman, M.L., ZXhang, Y.X., Haxo, Y.M. and Mat, Y.X., 2023. MRNA: The Next Big Thing in mRNA Vaccines. AC Investment Research Journal, 220(44).
- E. Altman. Constrained Markov decision processes, volume 7. CRC Press, 1999
- Chernozhukov V, Demirer M, Duflo E, Fernandez-Val I. 2018b. Generic machine learning inference on heteroge- nous treatment effects in randomized experiments. NBER Work. Pap. 24678
- L. Busoniu, R. Babuska, and B. D. Schutter. A comprehensive survey of multiagent reinforcement learning. IEEE Transactions of Systems, Man, and Cybernetics Part C: Applications and Reviews, 38(2), 2008.