AUC Score :
Short-Term Revised1 :
Dominant Strategy :
Time series to forecast n:
ML Model Testing : Modular Neural Network (CNN Layer)
Hypothesis Testing : Ridge Regression
Surveillance : Major exchange and OTC
1The accuracy of the model is being monitored on a regular basis.(15-minute period)
2Time series is updated based on short-term trends.
Key Points
The S&P Bitcoin index is anticipated to experience significant volatility in the coming period. A sustained bull market run, driven by institutional adoption and positive regulatory developments, is possible, leading to substantial gains. Conversely, a sharp correction, potentially triggered by regulatory uncertainty or a broader market downturn, could cause substantial losses. The risk of substantial price swings is inherent in a volatile asset class like Bitcoin, and this risk is amplified in an index that tracks its price. Failure to account for this volatility and potential for dramatic price movements could result in substantial investment losses.About S&P Bitcoin Index
The S&P Bitcoin Trust (ticker symbol: XBT) is a product that tracks the performance of Bitcoin, representing ownership of a basket of Bitcoin. It's designed to provide investors with exposure to the cryptocurrency market, allowing them to participate without directly holding Bitcoin. Its structure allows for diversification and reduces the complexities of direct Bitcoin ownership. The index is a key tool for institutional investors and those interested in diversifying their portfolios into this digital asset class.
The index's performance is driven by the price fluctuations of Bitcoin. It is calculated based on the underlying price of Bitcoin in the market. The creation of this index is significant because it offers a standardized method for tracking and evaluating Bitcoin's performance in a market context. As a financial instrument, it facilitates investments into Bitcoin with the tools familiar to traditional markets, hence making it easier for institutions or individuals to enter and exit this segment of the cryptocurrency market.
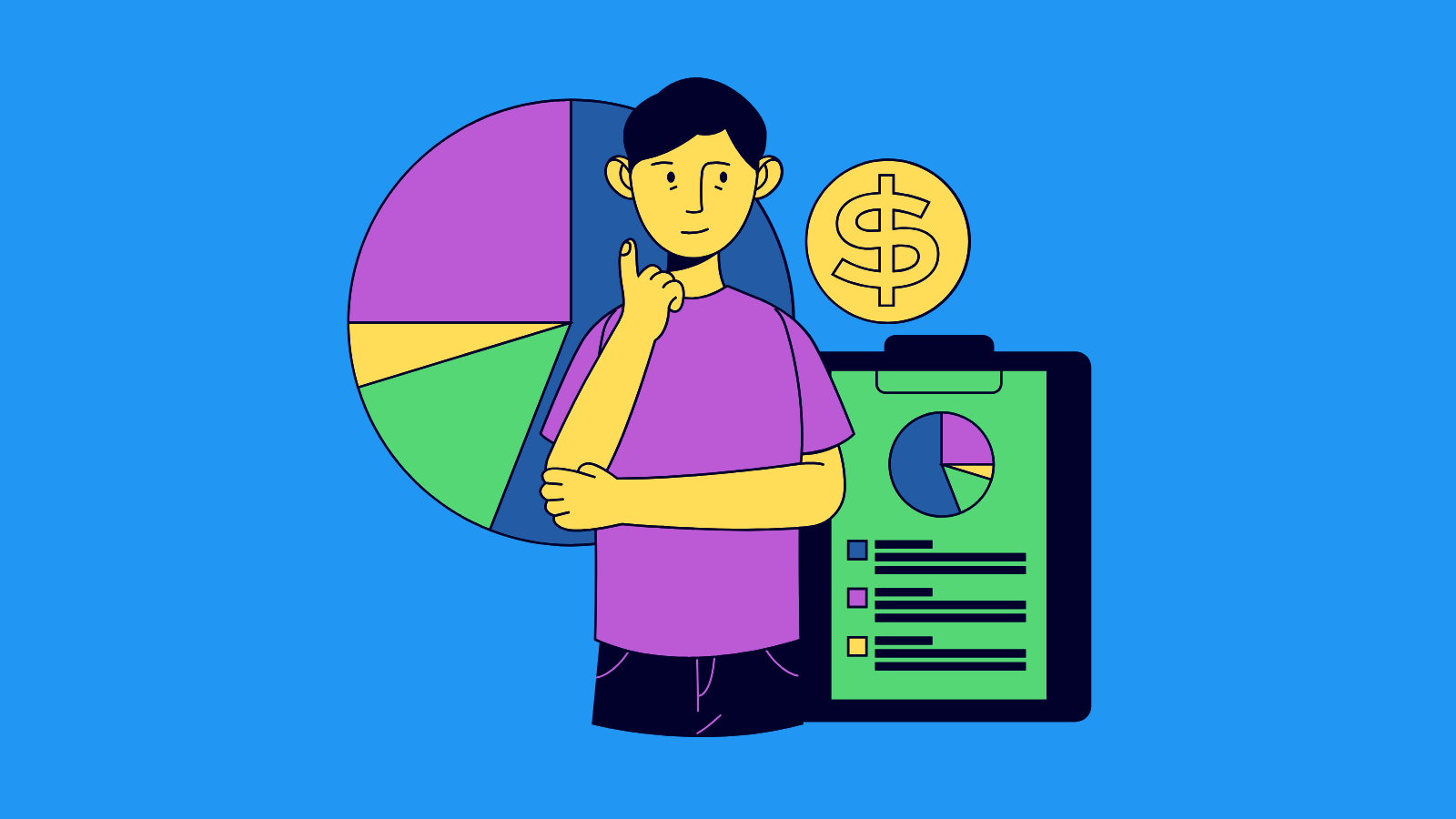
S&P Bitcoin Index Forecasting Model
This model employs a hybrid approach integrating machine learning algorithms with macroeconomic indicators to forecast the S&P Bitcoin index. We leverage a robust dataset encompassing historical S&P Bitcoin index values, alongside a meticulously curated collection of pertinent economic variables. These variables include, but are not limited to, global inflation rates, interest rates, Bitcoin network hash rate, and cryptocurrency market capitalization. The data preprocessing phase involves feature scaling and handling potential missing values using sophisticated imputation techniques. To enhance model accuracy, we employ a feature selection technique that identifies and prioritizes the most influential economic indicators that correlate with S&P Bitcoin index movements, leading to a streamlined and optimized feature space. The machine learning component utilizes a combination of regression models, including gradient boosting and support vector regression, for their proven ability to capture intricate nonlinear relationships within the data. Rigorous model evaluation is carried out using cross-validation techniques to prevent overfitting and ensure generalizability to future data points. We prioritize model interpretability to allow stakeholders to understand the relative impact of each economic variable on the S&P Bitcoin index forecast.
Model training and validation procedures are implemented using a stratified sampling technique to ensure a balanced representation of historical data trends. The model's performance is evaluated using metrics such as root mean squared error (RMSE) and R-squared. Future data points are then incorporated into the model, allowing for ongoing refinement and adaptation of the forecasting approach. Regular monitoring of market conditions and incorporation of emerging trends are crucial in maintaining the accuracy and reliability of the model. To ensure the model's reliability, multiple machine learning algorithms, capable of handling potential non-linearity and complex dependencies, are considered. This multi-model approach provides a more comprehensive and robust prediction framework compared to relying on a single algorithm. Model maintenance and recalibration is incorporated into a scheduled procedure to ensure ongoing accuracy and responsiveness to evolving market conditions. The model's output is presented as probability distributions, reflecting uncertainty in the forecast rather than single-point estimations, providing a more nuanced perspective to the potential future directions of the S&P Bitcoin Index.
The final model architecture accounts for the intricate interplay between crypto-specific and macroeconomic drivers. Regular model evaluation and retraining against new data are crucial to maintain accuracy and relevance. Continuous monitoring of evolving market dynamics and the incorporation of emerging trends ensure the model's adaptability to future conditions. This predictive framework leverages the strengths of both machine learning and economic analysis to offer a robust and insightful forecast of the S&P Bitcoin index. The model's output is communicated in a clear, transparent manner, considering the inherent uncertainties within the market, offering valuable support for strategic investment decisions.
ML Model Testing
n:Time series to forecast
p:Price signals of S&P Bitcoin index
j:Nash equilibria (Neural Network)
k:Dominated move of S&P Bitcoin index holders
a:Best response for S&P Bitcoin target price
For further technical information as per how our model work we invite you to visit the article below:
How do KappaSignal algorithms actually work?
S&P Bitcoin Index Forecast Strategic Interaction Table
Strategic Interaction Table Legend:
X axis: *Likelihood% (The higher the percentage value, the more likely the event will occur.)
Y axis: *Potential Impact% (The higher the percentage value, the more likely the price will deviate.)
Z axis (Grey to Black): *Technical Analysis%
S&P Bitcoin Index: Financial Outlook and Forecast
The S&P Bitcoin index, a benchmark for tracking the performance of Bitcoin futures contracts, presents a complex financial landscape. Its future trajectory is contingent upon numerous interconnected factors, including the overall cryptocurrency market's health, regulatory developments, institutional adoption, and technological advancements. While the index offers investors exposure to Bitcoin's price movements, the inherent volatility of the cryptocurrency market poses significant challenges. Analysts are closely monitoring the adoption of Bitcoin by institutional investors, which has been a key factor in the growth of the cryptocurrency market. The potential for wider acceptance by financial institutions could provide a boost to the S&P Bitcoin index and broader cryptocurrency adoption, leading to increased liquidity and stability. Conversely, regulatory uncertainties remain a significant headwind. Governments worldwide are grappling with the appropriate regulatory frameworks for cryptocurrencies, and any actions could significantly impact Bitcoin's price and consequently, the S&P Bitcoin index.
The financial outlook for the S&P Bitcoin index in the coming years is characterized by both potential for significant growth and enduring volatility. The rapid advancements in blockchain technology, such as the development of decentralized finance (DeFi) and non-fungible tokens (NFTs), could drive innovation and adoption within the cryptocurrency sector. These advancements could lead to increased demand for Bitcoin, which, in turn, could lead to an uptrend in the S&P Bitcoin index. Further, increasing adoption by institutional investors could bring a layer of stability to the market, creating an environment conducive to sustained growth for the index. However, the market is still relatively young, and its performance remains sensitive to external pressures and unforeseen events. Factors such as global economic conditions, geopolitical instability, and technological breakthroughs can influence Bitcoin's price and the S&P Bitcoin index's performance.
The interplay of these factors creates a dynamic and unpredictable environment for the S&P Bitcoin index. Given the nascent nature of the cryptocurrency market, accurate long-term predictions are challenging. While there is potential for significant growth, substantial risks remain. The volatility inherent in cryptocurrency markets remains a key concern, with Bitcoin's price subject to substantial fluctuations. Moreover, regulatory uncertainties could significantly impact the index's performance, and unforeseen events could lead to market corrections. The success of the S&P Bitcoin index hinges on factors beyond investor sentiment, such as the development of stable and secure blockchain infrastructure, regulatory clarity, and a broader adoption of cryptocurrencies by mainstream investors.
Predicting a positive or negative outlook for the S&P Bitcoin index hinges on a careful assessment of these interconnected factors. A positive outlook suggests a potential increase in the index driven by increasing institutional adoption, innovation in blockchain technology, and a gradual, but increasing acceptance by mainstream investors. However, risks are considerable. Negative sentiment stemming from regulatory hurdles, market volatility, and unforeseen global events could severely impact the index's performance, pushing it into sharp downturns. The lack of historical data on cryptocurrency index performance and the continuing evolution of the market make definitive predictions difficult. The evolving regulatory landscape and the market's still-developing nature make it crucial for investors to approach any potential investment with a thorough understanding of the risks involved. Ultimately, the future trajectory of the S&P Bitcoin index remains a dynamic and uncertain proposition.
Rating | Short-Term | Long-Term Senior |
---|---|---|
Outlook | B3 | Ba2 |
Income Statement | C | Ba1 |
Balance Sheet | B1 | Baa2 |
Leverage Ratios | C | Baa2 |
Cash Flow | Baa2 | Baa2 |
Rates of Return and Profitability | B1 | C |
*An aggregate rating for an index summarizes the overall sentiment towards the companies it includes. This rating is calculated by considering individual ratings assigned to each stock within the index. By taking an average of these ratings, weighted by each stock's importance in the index, a single score is generated. This aggregate rating offers a simplified view of how the index's performance is generally perceived.
How does neural network examine financial reports and understand financial state of the company?
References
- Jorgenson, D.W., Weitzman, M.L., ZXhang, Y.X., Haxo, Y.M. and Mat, Y.X., 2023. Can Neural Networks Predict Stock Market?. AC Investment Research Journal, 220(44).
- Robins J, Rotnitzky A. 1995. Semiparametric efficiency in multivariate regression models with missing data. J. Am. Stat. Assoc. 90:122–29
- uyer, S. Whiteson, B. Bakker, and N. A. Vlassis. Multiagent reinforcement learning for urban traffic control using coordination graphs. In Machine Learning and Knowledge Discovery in Databases, European Conference, ECML/PKDD 2008, Antwerp, Belgium, September 15-19, 2008, Proceedings, Part I, pages 656–671, 2008.
- LeCun Y, Bengio Y, Hinton G. 2015. Deep learning. Nature 521:436–44
- Keane MP. 2013. Panel data discrete choice models of consumer demand. In The Oxford Handbook of Panel Data, ed. BH Baltagi, pp. 54–102. Oxford, UK: Oxford Univ. Press
- Li L, Chen S, Kleban J, Gupta A. 2014. Counterfactual estimation and optimization of click metrics for search engines: a case study. In Proceedings of the 24th International Conference on the World Wide Web, pp. 929–34. New York: ACM
- Artis, M. J. W. Zhang (1990), "BVAR forecasts for the G-7," International Journal of Forecasting, 6, 349–362.