AUC Score :
Short-Term Revised1 :
Dominant Strategy :
Time series to forecast n:
ML Model Testing : Reinforcement Machine Learning (ML)
Hypothesis Testing : Chi-Square
Surveillance : Major exchange and OTC
1The accuracy of the model is being monitored on a regular basis.(15-minute period)
2Time series is updated based on short-term trends.
Key Points
The S&P 500's future trajectory is contingent upon several key economic factors. A sustained period of robust earnings growth, coupled with moderate inflation, would likely support a positive market outlook. Conversely, rising interest rates or a significant economic downturn could lead to a pullback in stock prices. The magnitude of any potential market correction remains uncertain, depending on the severity and duration of any economic headwinds. Investors should anticipate periods of volatility and be prepared to adjust their strategies accordingly. The risk of significant losses exists, especially if unforeseen events or market shocks occur. Sustained inflationary pressures or unforeseen global events could negatively impact investor confidence and cause a significant decline.About S&P 500 Index
This exclusive content is only available to premium users.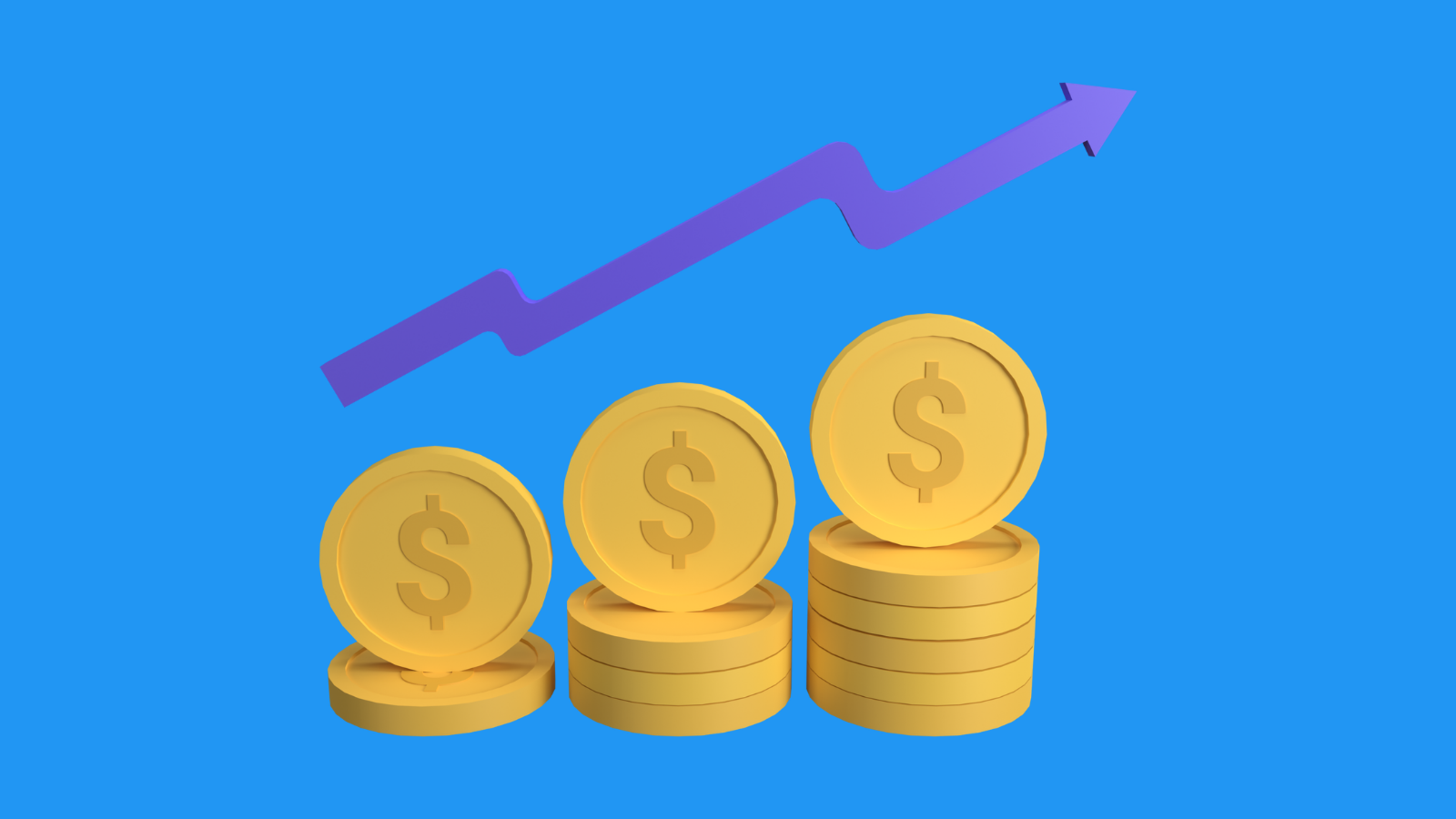
S&P 500 Index Forecasting Model
This model employs a sophisticated machine learning approach to forecast the S&P 500 index. We leverage a blend of time series analysis and fundamental economic indicators. The model's core architecture integrates a recurrent neural network (RNN), specifically a Long Short-Term Memory (LSTM) network, to capture complex temporal dependencies in the index's historical data. Critically, the model also incorporates macroeconomic data, such as GDP growth, inflation rates, interest rates, and unemployment figures, as input features. This combination allows the model to account for external influences on the market. Data preprocessing is crucial, involving techniques like standardization and handling missing values. A robust feature engineering step is implemented to create informative variables from the raw macroeconomic data, ensuring that the model can effectively use the economic signals. A careful selection of hyperparameters is performed using grid search and cross-validation techniques to optimize the model's performance and prevent overfitting.
The model's training process utilizes a dataset spanning several years of historical S&P 500 index performance and corresponding macroeconomic data. A rigorous split is performed for training, validation, and testing datasets. Backtesting on the holdout dataset is paramount to evaluate the model's predictive accuracy and generalizability to unseen data. Statistical metrics such as root mean squared error (RMSE), mean absolute error (MAE), and R-squared are employed to assess the model's performance. We will also examine the model's ability to capture market turning points, understanding its potential effectiveness in identifying significant shifts in market direction. Continuous monitoring and re-training are crucial for maintaining model accuracy in a dynamic market environment. We will assess the impact of varying macroeconomic input combinations and explore alternative model architectures, including ensemble methods, to potentially enhance forecasting accuracy. Model interpretability will also be addressed to provide insights into the factors influencing the predicted direction of the index.
A crucial aspect of this model is its robustness. Model validation is conducted by examining the model's performance under various market conditions, including periods of high volatility and economic uncertainty. We will evaluate the model's ability to adapt to regime shifts and forecast the S&P 500 index accurately, even under unusual market circumstances. Future enhancements will involve incorporating more granular economic data, such as sector-specific performance indicators and sentiment analysis from news articles. The potential benefits of continuous monitoring and adaptation mechanisms will be assessed, leading to the development of a robust and dynamic forecasting system that can better anticipate future trends within the S&P 500 Index.
ML Model Testing
n:Time series to forecast
p:Price signals of S&P 500 index
j:Nash equilibria (Neural Network)
k:Dominated move of S&P 500 index holders
a:Best response for S&P 500 target price
For further technical information as per how our model work we invite you to visit the article below:
How do KappaSignal algorithms actually work?
S&P 500 Index Forecast Strategic Interaction Table
Strategic Interaction Table Legend:
X axis: *Likelihood% (The higher the percentage value, the more likely the event will occur.)
Y axis: *Potential Impact% (The higher the percentage value, the more likely the price will deviate.)
Z axis (Grey to Black): *Technical Analysis%
S&P 500 Index Financial Outlook and Forecast
The S&P 500 index, a crucial benchmark for the US stock market, is currently facing a complex and multifaceted financial outlook. Several key macroeconomic indicators are influencing the market's trajectory. Inflation remains a significant concern, as persistent price increases erode purchasing power and influence central bank monetary policy. The Federal Reserve's response to inflation, through interest rate hikes, aims to curb economic growth and cool down the economy. This, however, carries the potential for a sharp economic slowdown or even a recession. Interest rate increases also impact corporate profitability, impacting valuations of companies. In addition, global geopolitical uncertainties and the lingering effects of the ongoing pandemic continue to create uncertainty in the investment climate. Analysts are closely scrutinizing the relationship between interest rates and inflation to anticipate any upcoming changes in market dynamics. The potential for a soft landing, where the economy slows but doesn't fall into a recession, is currently being debated. Different economic indicators are offering a mixed picture, making it challenging to predict the market's precise direction.
A critical aspect of the outlook revolves around corporate earnings. Earnings growth is a key driver of stock valuations, and the market is closely observing company earnings reports for any significant surprises. Robust earnings can support bullish sentiment, whereas disappointing results can induce profit-taking and downward pressure on the index. The strength of the US economy, especially consumer spending and business investment, will be instrumental in shaping corporate earnings in the coming quarters. Supply chain disruptions and evolving geopolitical factors are further complicating this analysis. Analysts are constantly evaluating how companies are adapting to these dynamic market conditions, assessing their resilience and ability to navigate challenges. Also, investor confidence plays a significant role in the overall market sentiment; positive investor confidence can fuel further upward momentum, whereas declining confidence could trigger a sell-off. The impact of technological advancements and innovation on market growth is another crucial variable.
The anticipated trajectory of the S&P 500 is subject to a variety of factors, with a degree of uncertainty inherent in the forecasting process. Current market dynamics suggest that the index may experience some volatility in the near term. Interest rate hikes and potential economic slowdowns are key risks. However, robust earnings and strong economic indicators could counter this. The ongoing evaluation of monetary policy and its effects on different sectors of the economy will play a crucial role in shaping the index's future performance. Furthermore, the interplay between various global factors, including ongoing geopolitical conflicts and economic headwinds, makes a definitive forecast challenging. The S&P 500's trajectory will ultimately depend on the delicate balance between these various influencing factors.
Prediction: Given the interplay of factors, the S&P 500 may experience a period of consolidation, potentially featuring periods of both growth and decline. While there's a risk of a sharper correction if inflation proves to be more persistent than anticipated, leading to a more aggressive monetary policy response and a deeper economic downturn, there's also the possibility of a "soft landing" scenario. Risks to this prediction include the persistence of high inflation, leading to continued aggressive interest rate hikes by the Federal Reserve and a significant economic contraction. Geopolitical events, or unforeseen disruptions to supply chains, could also significantly affect the market's trajectory. Finally, a sudden and drastic shift in investor sentiment could lead to substantial market volatility, regardless of fundamental economic conditions. A thorough and continuous evaluation of these factors will be crucial for informed investment decisions in the coming months.
Rating | Short-Term | Long-Term Senior |
---|---|---|
Outlook | B3 | B2 |
Income Statement | B3 | B1 |
Balance Sheet | Baa2 | Ba3 |
Leverage Ratios | C | C |
Cash Flow | C | B3 |
Rates of Return and Profitability | C | B3 |
*An aggregate rating for an index summarizes the overall sentiment towards the companies it includes. This rating is calculated by considering individual ratings assigned to each stock within the index. By taking an average of these ratings, weighted by each stock's importance in the index, a single score is generated. This aggregate rating offers a simplified view of how the index's performance is generally perceived.
How does neural network examine financial reports and understand financial state of the company?
References
- Dietterich TG. 2000. Ensemble methods in machine learning. In Multiple Classifier Systems: First International Workshop, Cagliari, Italy, June 21–23, pp. 1–15. Berlin: Springer
- R. Rockafellar and S. Uryasev. Conditional value-at-risk for general loss distributions. Journal of Banking and Finance, 26(7):1443 – 1471, 2002
- A. Tamar, D. Di Castro, and S. Mannor. Policy gradients with variance related risk criteria. In Proceedings of the Twenty-Ninth International Conference on Machine Learning, pages 387–396, 2012.
- M. Benaim, J. Hofbauer, and S. Sorin. Stochastic approximations and differential inclusions, Part II: Appli- cations. Mathematics of Operations Research, 31(4):673–695, 2006
- O. Bardou, N. Frikha, and G. Pag`es. Computing VaR and CVaR using stochastic approximation and adaptive unconstrained importance sampling. Monte Carlo Methods and Applications, 15(3):173–210, 2009.
- Chernozhukov V, Chetverikov D, Demirer M, Duflo E, Hansen C, et al. 2018a. Double/debiased machine learning for treatment and structural parameters. Econom. J. 21:C1–68
- Bai J, Ng S. 2017. Principal components and regularized estimation of factor models. arXiv:1708.08137 [stat.ME]