AUC Score :
Short-Term Revised1 :
Dominant Strategy :
Time series to forecast n:
ML Model Testing : Transductive Learning (ML)
Hypothesis Testing : Chi-Square
Surveillance : Major exchange and OTC
1The accuracy of the model is being monitored on a regular basis.(15-minute period)
2Time series is updated based on short-term trends.
Key Points
Ryman's future performance hinges heavily on the ongoing strength of the live entertainment sector. A sustained rebound in live music venue attendance and event bookings is crucial for sustained revenue growth. Failure to capitalize on this anticipated recovery could lead to disappointing occupancy rates and reduced earnings. Moreover, competition from other entertainment venues and evolving consumer preferences will pose significant challenges to maintaining market share. Economic downturns or unforeseen disruptions in the entertainment industry could negatively impact demand, resulting in reduced operating profits. However, Ryman's strong portfolio position and strategic acquisitions might provide a resilient buffer against these risks. The company's focus on diversification and potentially developing new revenue streams will likely influence investor confidence. Ultimately, long-term success depends on continued adaptation to market changes and a successful execution of their business strategies.About Ryman Hospitality Properties
Ryman Hospitality Properties (RHP) is a publicly traded real estate investment trust (REIT) focused on owning and operating live music venues. The company's portfolio includes a diverse mix of iconic music venues, theaters, and event spaces across the United States. Its venues host a variety of live performances, from concerts and shows to conferences and other events. RHP aims to provide stable and consistent income for investors by leveraging the strong demand for live entertainment. The company's strategy centers around maintaining and improving these properties to enhance their appeal and profitability.
RHP's operations encompass a range of activities including venue management, event booking, and property maintenance. The company's commitment to the live music and entertainment industry is evident in its dedication to fostering partnerships and collaborations. RHP's goal is to continue growing its portfolio and enhancing its properties to capitalize on the enduring popularity of live music and entertainment nationwide. Its long-term strategy likely involves identifying and acquiring similar properties in growth markets and continuing the company's focus on enhancing the customer experience at its venues.
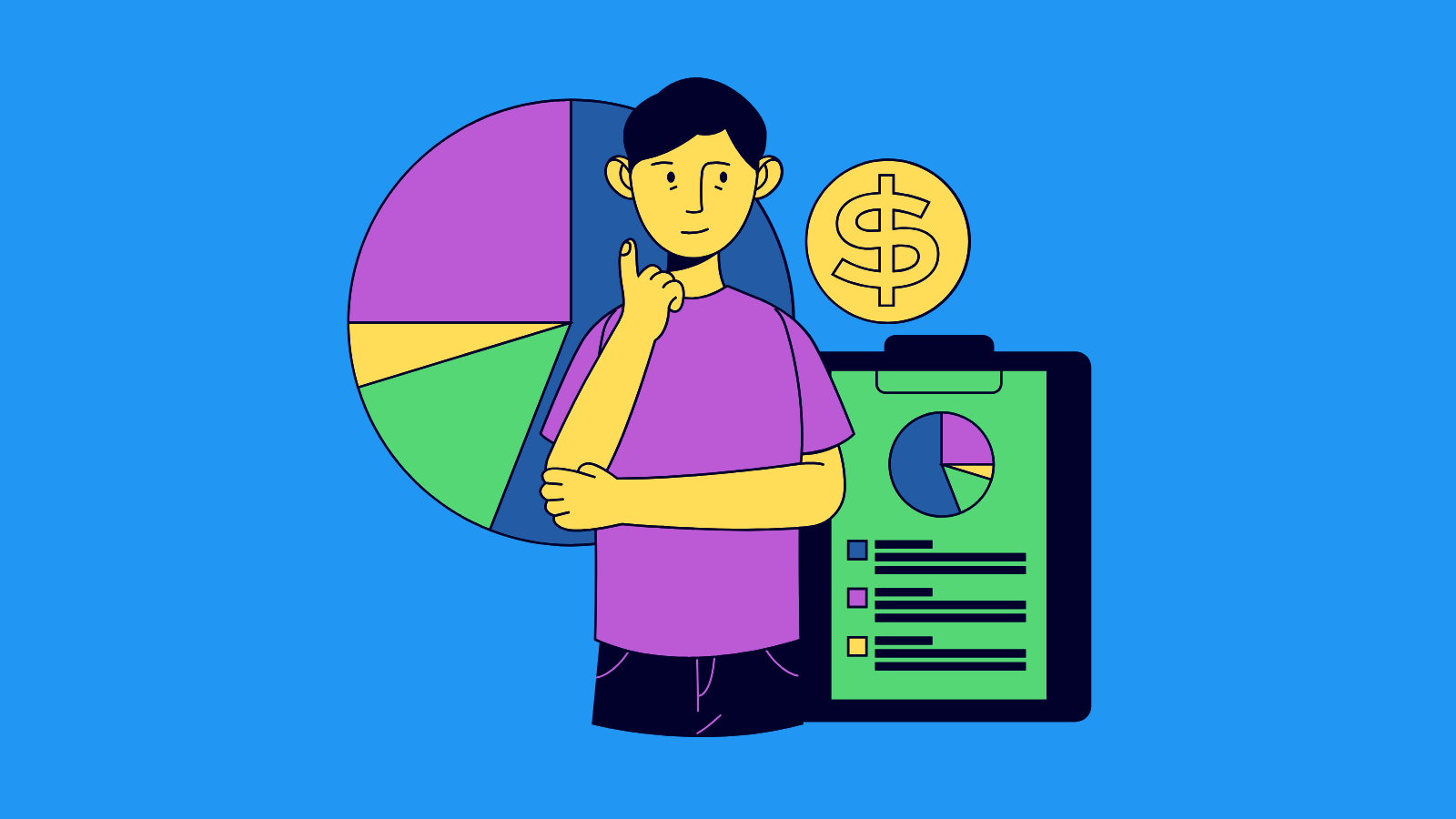
Ryman Hospitality Properties Inc. (RHP) Stock Price Forecasting Model
This model employs a hybrid approach integrating machine learning algorithms with macroeconomic indicators to forecast the stock price of Ryman Hospitality Properties Inc. (RHP). The core machine learning component utilizes a Gradient Boosting Regression model, specifically XGBoost. This algorithm is chosen for its superior performance in handling complex non-linear relationships within the dataset. Key features extracted from RHP's historical financial data include revenue, operating income, occupancy rates, and average daily rates (ADRs). Furthermore, macroeconomic variables are crucial and include inflation rates, interest rates, and GDP growth. These variables are anticipated to have a significant impact on RHP's performance given the nature of its business. The model pre-processes data through techniques such as standardization and handling missing values to ensure optimal performance. Data is split into training, validation, and testing sets for rigorous evaluation, preventing overfitting. We evaluate the model's performance through metrics such as Root Mean Squared Error (RMSE) and R-squared, crucial for assessing the model's accuracy and fit to the data. The inclusion of macroeconomic indicators is paramount for capturing external factors affecting RHP's performance, and the model will provide crucial insight into their influence.
The macroeconomic variables are integrated into the model by creating lagged variables to capture the potential impact of future events. We also employ feature engineering techniques, such as creating interaction terms between financial and macroeconomic variables, to capture complex relationships. Feature importance analysis will be performed to understand which factors most influence the RHP stock price. The XGBoost model's ability to handle high-dimensional data and complex interactions is leveraged to create a predictive model that captures the subtleties in RHP's stock performance. Critical to the model's accuracy and reliability is the careful selection of relevant features and the appropriate handling of data. We consider the historical relationship between RHP's performance and macroeconomic indicators using statistical analysis.The model is rigorously tested on unseen data and is continually updated to reflect new market trends. This ensures that our forecasts maintain their accuracy and relevance over time. Continuous monitoring and refinement of the model will be undertaken to maintain its predictive power.
The final output of this model will be a forecast of RHP's stock price over a specified future period. This forecast will be presented in a clear and easily interpretable format, including confidence intervals. We will provide insights and analysis into the drivers of the predicted price movements, highlighting the importance of both company-specific factors and macroeconomic trends. The model's output will be utilized for various investment strategies and decision-making processes. This information can aid in portfolio diversification, risk management, and potentially enhance the accuracy of investment decisions. Continuous monitoring and updates of the model will ensure the validity and accuracy of the forecast as the market evolves. This model aims to provide a valuable tool for investors and stakeholders seeking insights into RHP's future performance. Visualizations will be included to clearly convey the relationship between independent variables and the forecast.
ML Model Testing
n:Time series to forecast
p:Price signals of Ryman Hospitality Properties stock
j:Nash equilibria (Neural Network)
k:Dominated move of Ryman Hospitality Properties stock holders
a:Best response for Ryman Hospitality Properties target price
For further technical information as per how our model work we invite you to visit the article below:
How do KappaSignal algorithms actually work?
Ryman Hospitality Properties Stock Forecast (Buy or Sell) Strategic Interaction Table
Strategic Interaction Table Legend:
X axis: *Likelihood% (The higher the percentage value, the more likely the event will occur.)
Y axis: *Potential Impact% (The higher the percentage value, the more likely the price will deviate.)
Z axis (Grey to Black): *Technical Analysis%
Ryman Hospitality Properties Inc. Financial Outlook and Forecast
Ryman Hospitality Properties (RHP) operates as a publicly traded real estate investment trust (REIT) focused on owning and managing live music venues and related entertainment spaces. Their financial outlook hinges on several key factors, most notably the ongoing recovery of the live music industry following the COVID-19 pandemic. The company's portfolio's performance is closely tied to consumer confidence and spending patterns related to entertainment. Analyzing historical trends in concert attendance, event ticket sales, and overall economic activity provides valuable insight into potential future performance. Revenue generation for RHP is driven by rental income from these venues, along with potential ancillary revenue streams like food and beverage sales. The ability to attract and retain top-tier musical acts is crucial for maintaining occupancy levels and driving demand. Factors like changing consumer preferences, evolving event booking strategies, and potential disruptions in the entertainment industry will play a key role in shaping RHP's future financial performance.
The current economic climate presents both opportunities and challenges for RHP. A robust and healthy economy with increasing consumer spending on entertainment generally benefits the company. However, inflation, interest rate hikes, and potential recessions can impact consumer spending habits and attendance at live music venues. RHP's financial performance is intricately linked to the health of the broader economy. Moreover, competition in the live entertainment sector is a significant consideration. The emergence of alternative entertainment options, like streaming services, could potentially reduce demand for live performances. Successful venue management, including strategic pricing and promotional strategies, is essential for maximizing revenue streams. Furthermore, the effectiveness of their leasing strategies will directly impact the company's financial performance. Efficient utilization of venue space and maintaining a diverse lineup of events are also crucial for long-term success.
A forecast for RHP's financial outlook requires careful consideration of these complex factors. Based on the current trends and potential future developments in the entertainment industry and the broader economy, there is potential for a moderate yet consistent growth in revenue and earnings in the near future, assuming the live music sector continues its recovery post-pandemic. Careful analysis of leasing agreements, occupancy rates, and overall financial statements are needed to understand the potential financial performance. The ability to adapt to changing consumer trends and effectively market their venues will be crucial. The long-term outlook for RHP relies heavily on the sustained growth and health of the live music industry, and the effectiveness of the company's risk management strategies. Understanding and mitigating potential risks, such as changes in consumer preferences, economic downturns, or unforeseen competition, is fundamental to achieving sustainable success.
Predicting the future is inherently uncertain. A positive outlook hinges on the sustained recovery of the live music industry and a stable economic environment. Success relies on efficient management practices, adaptable strategies for the entertainment sector, and successful adaptation to potential risks. However, significant risks could negatively impact this forecast. For example, a prolonged economic downturn, a decline in concert attendance due to shifting consumer preferences, or an unforeseen industry-wide disruption could negatively impact revenue and profitability. Another risk to consider is the rising interest rate environment, which could impact borrowing costs and negatively influence the company's financial performance. Competition for talent and venue bookings, changes in consumer behavior, or unforeseen issues with the economy are also crucial factors to observe. Therefore, while a positive outlook is possible, a thorough understanding of these potential risks is essential for evaluating the financial stability and future performance of Ryman Hospitality Properties.
Rating | Short-Term | Long-Term Senior |
---|---|---|
Outlook | Ba3 | Ba3 |
Income Statement | B2 | Baa2 |
Balance Sheet | Baa2 | Baa2 |
Leverage Ratios | C | Baa2 |
Cash Flow | B3 | B2 |
Rates of Return and Profitability | Baa2 | Caa2 |
*Financial analysis is the process of evaluating a company's financial performance and position by neural network. It involves reviewing the company's financial statements, including the balance sheet, income statement, and cash flow statement, as well as other financial reports and documents.
How does neural network examine financial reports and understand financial state of the company?
References
- Friedberg R, Tibshirani J, Athey S, Wager S. 2018. Local linear forests. arXiv:1807.11408 [stat.ML]
- Candès E, Tao T. 2007. The Dantzig selector: statistical estimation when p is much larger than n. Ann. Stat. 35:2313–51
- S. J. Russell and P. Norvig. Artificial Intelligence: A Modern Approach. Prentice Hall, Englewood Cliffs, NJ, 3nd edition, 2010
- Bamler R, Mandt S. 2017. Dynamic word embeddings via skip-gram filtering. In Proceedings of the 34th Inter- national Conference on Machine Learning, pp. 380–89. La Jolla, CA: Int. Mach. Learn. Soc.
- D. White. Mean, variance, and probabilistic criteria in finite Markov decision processes: A review. Journal of Optimization Theory and Applications, 56(1):1–29, 1988.
- P. Milgrom and I. Segal. Envelope theorems for arbitrary choice sets. Econometrica, 70(2):583–601, 2002
- Z. Wang, T. Schaul, M. Hessel, H. van Hasselt, M. Lanctot, and N. de Freitas. Dueling network architectures for deep reinforcement learning. In Proceedings of the International Conference on Machine Learning (ICML), pages 1995–2003, 2016.