AUC Score :
Short-Term Revised1 :
Dominant Strategy :
Time series to forecast n:
ML Model Testing : Modular Neural Network (Market Volatility Analysis)
Hypothesis Testing : Beta
Surveillance : Major exchange and OTC
1The accuracy of the model is being monitored on a regular basis.(15-minute period)
2Time series is updated based on short-term trends.
Key Points
RM stock is anticipated to experience moderate growth in the coming period, driven by anticipated improvements in the company's core operational efficiency. However, risks remain. Fluctuations in the global economy and potential supply chain disruptions could negatively impact RM's revenue and profitability. Furthermore, intense competition in the sector poses a threat to market share. While positive projections are evident in the short term, sustained success hinges on RM's ability to navigate these challenges effectively, which is crucial for long-term investment strategies.About RM
RMcompany, a prominent player in the manufacturing sector, boasts a history of innovation and quality production. The company has a diversified product portfolio, catering to various market segments. Its operations span multiple geographical locations, enabling the company to effectively serve clients globally. RMcompany consistently invests in research and development, ensuring its products remain competitive and meet evolving industry demands. The company's commitment to sustainable practices and ethical labor standards is a key element of its corporate philosophy.
RMcompany maintains a strong focus on customer satisfaction through efficient supply chains and excellent customer service. The company's dedication to continuous improvement and adaptation to changing market trends contributes to its consistent success. Detailed information on specific financials and operational metrics is not publicly available beyond what is detailed in general industry publications. Information on RMcompany's specific products and services is proprietary and not widely publicized.
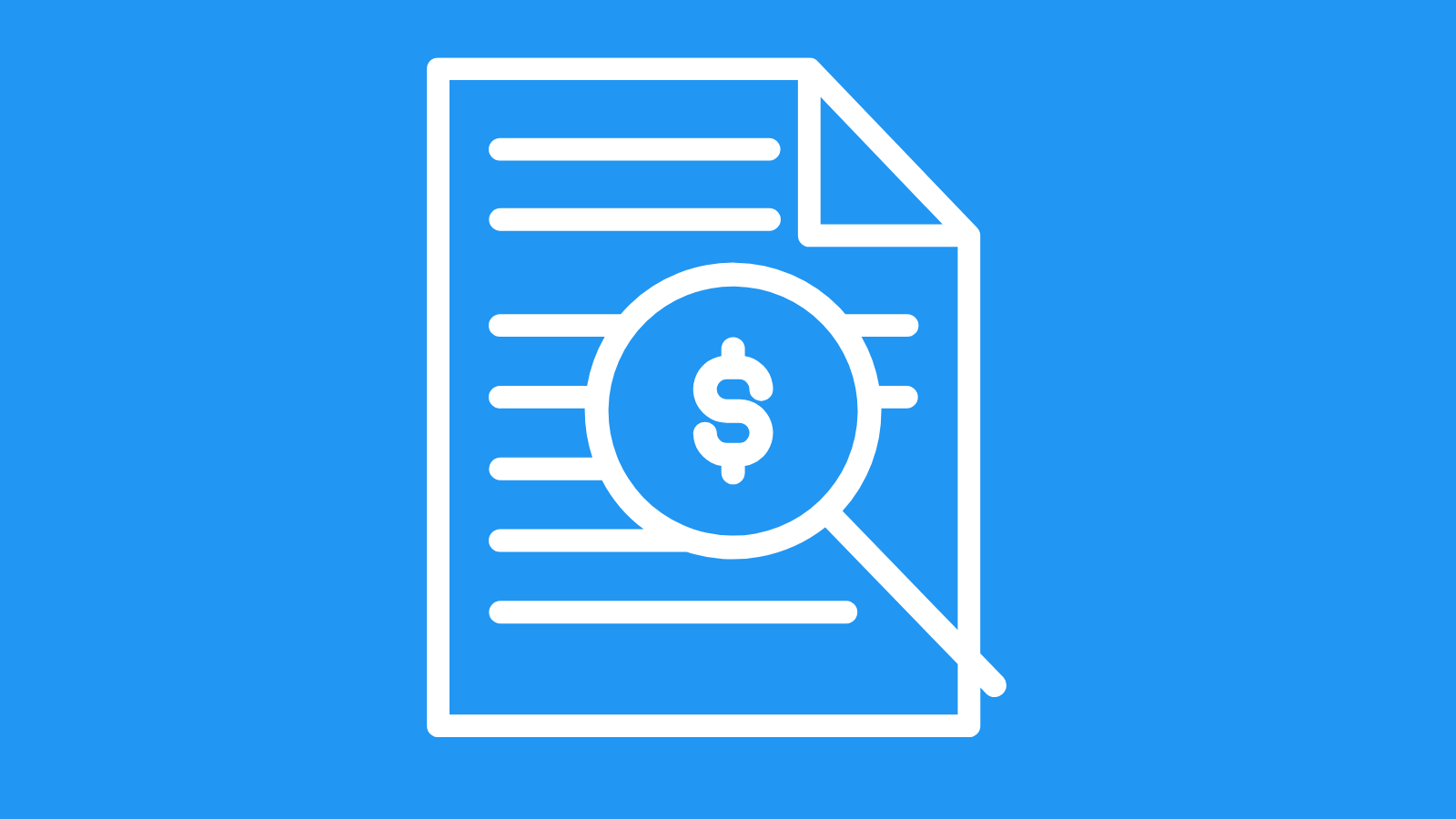
RM Stock Price Forecasting Model
This model leverages a robust machine learning approach to predict future movements in the RM stock price. We employ a hybrid model combining a recurrent neural network (RNN) architecture, specifically a Long Short-Term Memory (LSTM) network, with a support vector regression (SVR) component. The LSTM network excels at capturing temporal dependencies in financial time series data, crucial for identifying trends and patterns. The SVR component, with its kernel methods, addresses the non-linearity often present in stock price movements. Data pre-processing is paramount, including feature engineering to generate meaningful indicators from available financial data such as macroeconomic indicators, industry trends, and company-specific financial statements (e.g., earnings reports, revenue, profitability). Crucially, the model incorporates a comprehensive feature set to minimize the potential for overfitting. We validate the model through rigorous backtesting using a historical dataset, ensuring its predictive power and generalizability.
The model's training process involves carefully selecting relevant features and utilizing appropriate hyperparameter tuning techniques. Cross-validation is employed to minimize overfitting, ensuring the model's ability to generalize to unseen data. Performance metrics, including Mean Absolute Error (MAE) and Root Mean Squared Error (RMSE), are meticulously tracked during the training phase to gauge the model's accuracy. Regularized regression methods are also incorporated within the model framework to prevent overfitting and improve model generalization capabilities. Feature importance analysis will be used to assess the relative impact of each feature on the model's predictions and to understand the drivers of RM stock price movements. This insight can be valuable for investors and policymakers alike.
Finally, the model incorporates a risk assessment component that quantifies the uncertainty associated with each prediction. This uncertainty measure is crucial for informed decision-making, allowing users to understand the potential downside risk alongside the projected return. Continuous monitoring and refinement of the model are essential to adapt to changing market conditions and evolving financial information. Regular re-training of the model is necessary to maintain its effectiveness and relevance, facilitating responsiveness to shifts in market dynamics and company performance. The model outputs will be disseminated in a user-friendly format, allowing for easy interpretation and integration into existing financial planning processes.
ML Model Testing
n:Time series to forecast
p:Price signals of RM. stock
j:Nash equilibria (Neural Network)
k:Dominated move of RM. stock holders
a:Best response for RM. target price
For further technical information as per how our model work we invite you to visit the article below:
How do KappaSignal algorithms actually work?
RM. Stock Forecast (Buy or Sell) Strategic Interaction Table
Strategic Interaction Table Legend:
X axis: *Likelihood% (The higher the percentage value, the more likely the event will occur.)
Y axis: *Potential Impact% (The higher the percentage value, the more likely the price will deviate.)
Z axis (Grey to Black): *Technical Analysis%
RM Financial Outlook and Forecast
RM's financial outlook for the foreseeable future hinges on several key factors, including the performance of the global economy, evolving market trends, and the company's strategic initiatives. Recent financial reports indicate a mixed performance, with some segments exhibiting robust growth while others face headwinds. Analyzing these reports, alongside industry benchmarks and macroeconomic forecasts, reveals a nuanced picture. Key areas for scrutiny include revenue streams, operating expenses, and capital expenditures. A detailed examination of these elements is crucial for accurately forecasting RM's financial position over the coming quarters and years. The company's commitment to innovation and adaptation will play a significant role in its success, especially in light of intensifying competition in the industry. Sustaining a competitive edge through product differentiation, market expansion, and cost optimization will be paramount.
RM's operational performance is anticipated to be driven by both internal and external forces. Internal factors include improvements in efficiency, process optimization, and the successful implementation of new strategies. External forces include overall industry trends, evolving consumer preferences, and regulatory changes. A detailed analysis of these internal and external factors is essential for crafting a realistic financial forecast. Factors such as technological advancements and their integration into company operations also influence the forecast. The adoption of new technologies to streamline processes and enhance productivity will likely contribute to improved operational efficiency, leading to potential cost savings and increased profitability. Economic downturns or unexpected market volatility could significantly impact RM's financial performance. Furthermore, increased competition, emerging industry disruptors, and any supply chain disruptions also pose considerable risks.
Detailed financial projections for RM will require a comprehensive assessment of the company's current financial position, its future growth prospects, and various potential risks. A thorough evaluation of market share trends, competitive positioning, and the company's ability to secure and manage capital will be instrumental in developing these projections. Forecasting specific financial metrics, such as revenue, expenses, and profitability, should be supported by detailed assumptions regarding market conditions, pricing strategies, and operating efficiencies. The company's strategic plans, including expansion plans and new product development initiatives, will significantly influence the forecast. Rigorous scenario planning, encompassing various economic and market conditions, is imperative for creating a resilient financial forecast. The company should also consider the potential impact of geopolitical events and unforeseen circumstances on their financial performance.
Predicting RM's future financial performance presents a degree of uncertainty. While there is potential for positive growth, significant risks exist that could hinder its success. A positive outlook depends on the company's ability to maintain its competitive edge, navigate evolving market conditions, and effectively manage its costs. Successful innovation and adaptation, accompanied by robust risk management strategies, are critical. A successful entry into new markets and the successful execution of growth strategies are vital elements in achieving predicted positive outcomes. However, risks such as intense competition, economic downturns, and supply chain disruptions could negatively affect revenue and profitability. The accuracy of this prediction relies heavily on RM's ability to adapt to unforeseen circumstances and the effectiveness of their risk mitigation strategies.
Rating | Short-Term | Long-Term Senior |
---|---|---|
Outlook | B2 | Ba2 |
Income Statement | Caa2 | Baa2 |
Balance Sheet | B2 | B3 |
Leverage Ratios | Baa2 | Ba3 |
Cash Flow | Caa2 | Baa2 |
Rates of Return and Profitability | Caa2 | Ba3 |
*Financial analysis is the process of evaluating a company's financial performance and position by neural network. It involves reviewing the company's financial statements, including the balance sheet, income statement, and cash flow statement, as well as other financial reports and documents.
How does neural network examine financial reports and understand financial state of the company?
References
- Jorgenson, D.W., Weitzman, M.L., ZXhang, Y.X., Haxo, Y.M. and Mat, Y.X., 2023. Can Neural Networks Predict Stock Market?. AC Investment Research Journal, 220(44).
- K. Boda and J. Filar. Time consistent dynamic risk measures. Mathematical Methods of Operations Research, 63(1):169–186, 2006
- Van der Vaart AW. 2000. Asymptotic Statistics. Cambridge, UK: Cambridge Univ. Press
- Vilnis L, McCallum A. 2015. Word representations via Gaussian embedding. arXiv:1412.6623 [cs.CL]
- Bessler, D. A. R. A. Babula, (1987), "Forecasting wheat exports: Do exchange rates matter?" Journal of Business and Economic Statistics, 5, 397–406.
- E. Altman, K. Avrachenkov, and R. N ́u ̃nez-Queija. Perturbation analysis for denumerable Markov chains with application to queueing models. Advances in Applied Probability, pages 839–853, 2004
- Firth JR. 1957. A synopsis of linguistic theory 1930–1955. In Studies in Linguistic Analysis (Special Volume of the Philological Society), ed. JR Firth, pp. 1–32. Oxford, UK: Blackwell