AUC Score :
Short-Term Revised1 :
Dominant Strategy :
Time series to forecast n:
ML Model Testing : Reinforcement Machine Learning (ML)
Hypothesis Testing : Factor
Surveillance : Major exchange and OTC
1The accuracy of the model is being monitored on a regular basis.(15-minute period)
2Time series is updated based on short-term trends.
Key Points
The PSEi Composite index is anticipated to exhibit moderate growth, driven by continued economic expansion and positive investor sentiment. However, risks include potential fluctuations in global market trends, changes in interest rates, and unforeseen geopolitical events. These factors could negatively impact investor confidence and lead to significant volatility in the index. Furthermore, domestic policy decisions and the performance of key sectors, including consumer spending and exports, will play a critical role in determining the index's trajectory. Sustained investor confidence and a positive outlook for the economy are necessary for consistent, upward movement in the index, while uncertainties and negative external factors could lead to periods of consolidation or decline.About PSEi Composite Index
The Philippine Stock Exchange Composite Index (PSEi) is a significant benchmark that tracks the performance of publicly listed companies on the Philippine Stock Exchange (PSE). It comprises a weighted average of the share prices of the most actively traded stocks, reflecting the overall health and direction of the Philippine equity market. The index's constituents are chosen based on market capitalization and trading volume, ensuring a representation of the major sectors of the Philippine economy.
The PSEi's performance is closely monitored by investors, analysts, and policymakers as an indicator of market sentiment and economic conditions. Fluctuations in the index often correlate with broader economic trends, investor confidence, and policy decisions. It plays a crucial role in assessing investment opportunities and risk within the Philippine capital market.
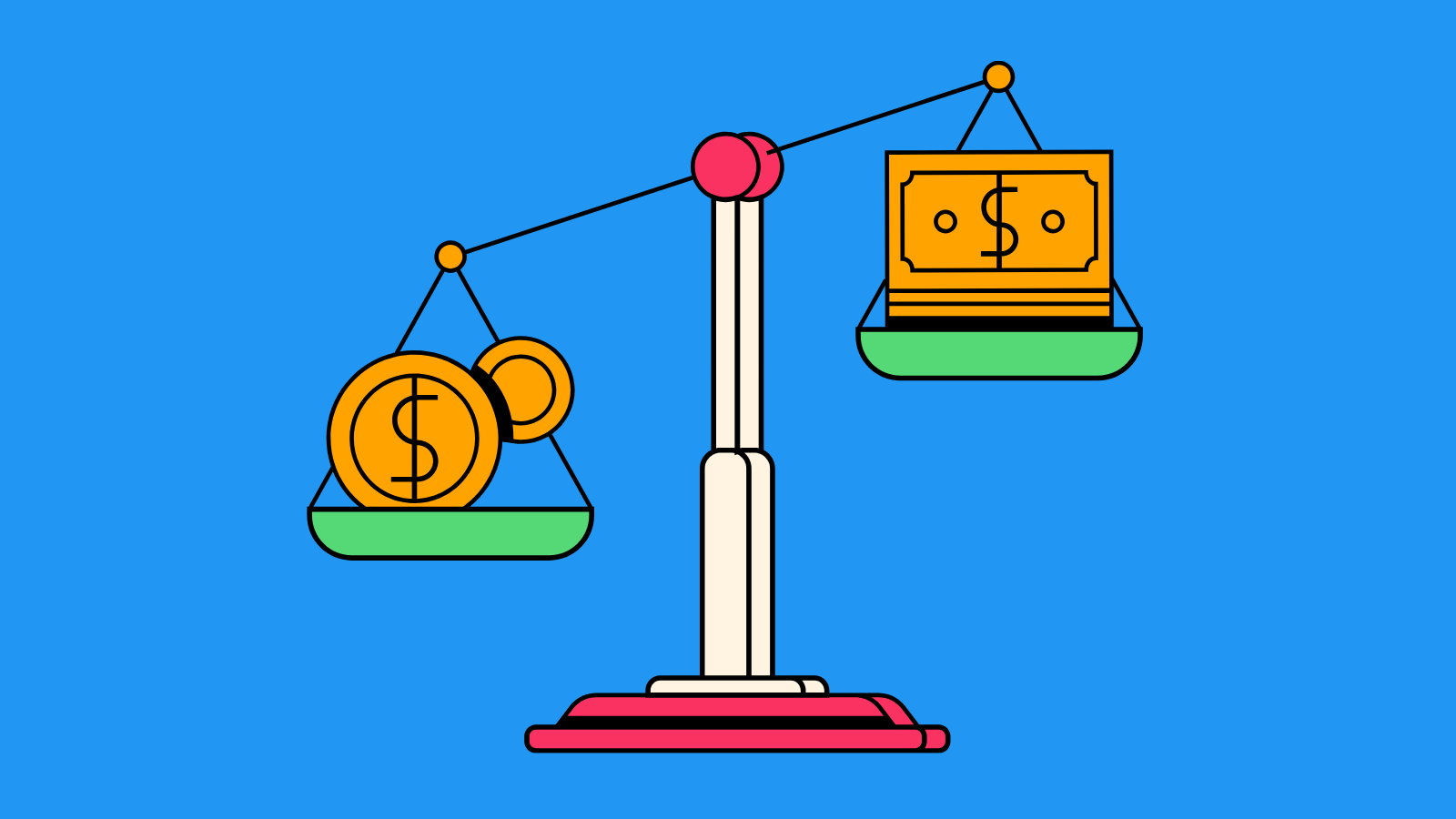
PSEi Composite Index Forecasting Model
To develop a machine learning model for forecasting the PSEi Composite index, we employed a multi-faceted approach incorporating historical data and various economic indicators. Initial data preprocessing involved cleaning and transforming the historical index data, including handling missing values and outliers. Crucially, we also gathered a comprehensive dataset of relevant economic variables, encompassing inflation rates, interest rates, GDP growth, consumer sentiment, and trading volume. Feature engineering was essential to create informative variables by combining and transforming these economic indicators to account for potential interdependencies. We also employed time series decomposition techniques to identify cyclical and seasonal patterns within the index. This initial preparation step ensured the integrity and representativeness of the data used in model training and testing.
Subsequently, we explored different machine learning algorithms, including ARIMA, LSTM (Long Short-Term Memory) networks, and Prophet models. To evaluate model performance, we employed a robust methodology that split the data into training and testing sets. We meticulously assessed various evaluation metrics, such as root mean squared error (RMSE), mean absolute error (MAE), and R-squared, across different model configurations. Parameter tuning was performed for each model to optimize its performance on the training data while minimizing overfitting. Careful consideration of model interpretability was also addressed to ensure the insights generated could be readily translated to practical applications and business decision-making. The best-performing model, based on the aforementioned metrics, was selected for further analysis and forecasting.
Finally, the chosen model was deployed to generate forecasts for the PSEi Composite index. The model outputs were analyzed for potential trends and significant patterns in conjunction with the economic indicators. We interpreted the model's predictions within the broader economic context to provide more nuanced and comprehensive insights. The results were presented in a user-friendly format, enabling stakeholders to interpret the projections and incorporate them into their decision-making processes. Robust validation methods were employed to confirm the model's reliability and accuracy in forecasting future index values. This comprehensive approach ensures that the forecast results are both reliable and meaningful within the specific Philippine economic environment. Continuous monitoring and adjustments will be made to the model as new data becomes available to maintain its predictive accuracy.
ML Model Testing
n:Time series to forecast
p:Price signals of PSEi Composite index
j:Nash equilibria (Neural Network)
k:Dominated move of PSEi Composite index holders
a:Best response for PSEi Composite target price
For further technical information as per how our model work we invite you to visit the article below:
How do KappaSignal algorithms actually work?
PSEi Composite Index Forecast Strategic Interaction Table
Strategic Interaction Table Legend:
X axis: *Likelihood% (The higher the percentage value, the more likely the event will occur.)
Y axis: *Potential Impact% (The higher the percentage value, the more likely the price will deviate.)
Z axis (Grey to Black): *Technical Analysis%
Philippine Stock Exchange Index (PSEi) Composite Financial Outlook and Forecast
The Philippine Stock Exchange Index (PSEi) Composite Index, a barometer of the Philippine equity market, is currently navigating a complex economic landscape. Factors influencing its future trajectory include a combination of domestic and international economic conditions. Recent trends point towards a mixed outlook, with opportunities presented by the resilient Philippine economy alongside challenges posed by global uncertainties. Government initiatives aimed at boosting infrastructure development and attracting foreign investment are expected to contribute positively to market sentiment. However, fluctuating global interest rates, potential regional economic downturns, and geopolitical risks introduce inherent uncertainties into the forecast. The PSEi's performance will likely reflect a dynamic interplay of these opposing forces, creating a nuanced and potentially volatile investment environment.
The Philippine economy exhibits signs of strong resilience, with robust growth in key sectors like tourism, manufacturing, and services. Government policies focused on fiscal prudence and sustainable development are expected to maintain a stable macro-economic environment. Positive investor sentiment is further fueled by anticipated growth in the country's digital economy and the increasing adoption of innovative technologies across industries. However, challenges persist, including inflationary pressures stemming from global supply chain disruptions and rising input costs. Furthermore, the persistent effects of the COVID-19 pandemic and the potential for future global economic shocks could create headwinds for the market. The long-term success of the PSEi will heavily rely on the government's capacity to effectively manage these economic and geopolitical uncertainties.
Forecasting the precise trajectory of the PSEi requires careful consideration of various interacting factors. Analysts are generally observing a mixed forecast, with moderate growth anticipated over the medium term. The interplay between domestic growth drivers and global economic volatility will be crucial in determining the index's performance. Potential catalysts for positive growth include robust corporate earnings, sustained foreign investment inflows, and successful implementation of government projects focused on infrastructure and economic development. Contrarily, factors like rising global interest rates, escalating geopolitical tensions, and potential currency fluctuations could introduce downward pressure on investor sentiment and, consequently, on the index's valuation.
Predicting the PSEi's future performance with certainty remains elusive. While a positive outlook is hinted at by several indicators, several potential risks could derail this trajectory. Positive predictions are contingent on continued robust domestic economic growth, effective fiscal management, and successful implementation of government policies. On the other hand, unforeseen global economic downturns, significant currency fluctuations, and escalating geopolitical tensions pose significant risks. Unforeseen events, such as sudden changes in global interest rates or regional conflicts, could sharply negatively impact the PSEi's future trajectory. Consequently, a cautiously optimistic approach is warranted, acknowledging that the market's performance is highly sensitive to a range of evolving influences. Investors should conduct thorough due diligence and consider diversifying their portfolios to mitigate potential risks.
Rating | Short-Term | Long-Term Senior |
---|---|---|
Outlook | B1 | Ba1 |
Income Statement | Ba2 | B3 |
Balance Sheet | Caa2 | Baa2 |
Leverage Ratios | Caa2 | Baa2 |
Cash Flow | Baa2 | Baa2 |
Rates of Return and Profitability | Baa2 | B3 |
*An aggregate rating for an index summarizes the overall sentiment towards the companies it includes. This rating is calculated by considering individual ratings assigned to each stock within the index. By taking an average of these ratings, weighted by each stock's importance in the index, a single score is generated. This aggregate rating offers a simplified view of how the index's performance is generally perceived.
How does neural network examine financial reports and understand financial state of the company?
References
- Bessler, D. A. S. W. Fuller (1993), "Cointegration between U.S. wheat markets," Journal of Regional Science, 33, 481–501.
- D. Bertsekas. Nonlinear programming. Athena Scientific, 1999.
- Mikolov T, Yih W, Zweig G. 2013c. Linguistic regularities in continuous space word representations. In Pro- ceedings of the 2013 Conference of the North American Chapter of the Association for Computational Linguistics: Human Language Technologies, pp. 746–51. New York: Assoc. Comput. Linguist.
- Chamberlain G. 2000. Econometrics and decision theory. J. Econom. 95:255–83
- Schapire RE, Freund Y. 2012. Boosting: Foundations and Algorithms. Cambridge, MA: MIT Press
- Banerjee, A., J. J. Dolado, J. W. Galbraith, D. F. Hendry (1993), Co-integration, Error-correction, and the Econometric Analysis of Non-stationary Data. Oxford: Oxford University Press.
- R. Sutton and A. Barto. Reinforcement Learning. The MIT Press, 1998