AUC Score :
Short-Term Revised1 :
Dominant Strategy :
Time series to forecast n:
ML Model Testing : Modular Neural Network (Market Direction Analysis)
Hypothesis Testing : Logistic Regression
Surveillance : Major exchange and OTC
1The accuracy of the model is being monitored on a regular basis.(15-minute period)
2Time series is updated based on short-term trends.
Key Points
KBR is expected to benefit from continued government spending on defense and infrastructure projects, which should drive revenue growth in the near term. The company's strong backlog of projects and its focus on expanding into new markets, such as energy transition and digital engineering, also bode well for the future. However, KBR faces risks related to geopolitical instability, potential cost overruns on projects, and competition from other engineering and construction firms. The company's reliance on government contracts also makes it vulnerable to changes in government spending priorities.About KBR Inc.
KBR is a global engineering, construction, and services company headquartered in Houston, Texas. The company provides various services to the government and private sectors, including energy, infrastructure, technology, and defense. KBR's focus on advanced technologies, environmental solutions, and innovative project delivery methods positions them as a leading provider in several industries. KBR's diverse portfolio of projects contributes to the development of critical infrastructure and resources worldwide.
KBR's history dates back to the early 20th century and has a strong legacy in engineering and construction. The company has worked on numerous high-profile projects, including the construction of oil refineries, pipelines, and power plants. KBR's commitment to innovation and sustainability drives their efforts to deliver solutions that meet the evolving needs of clients and communities.
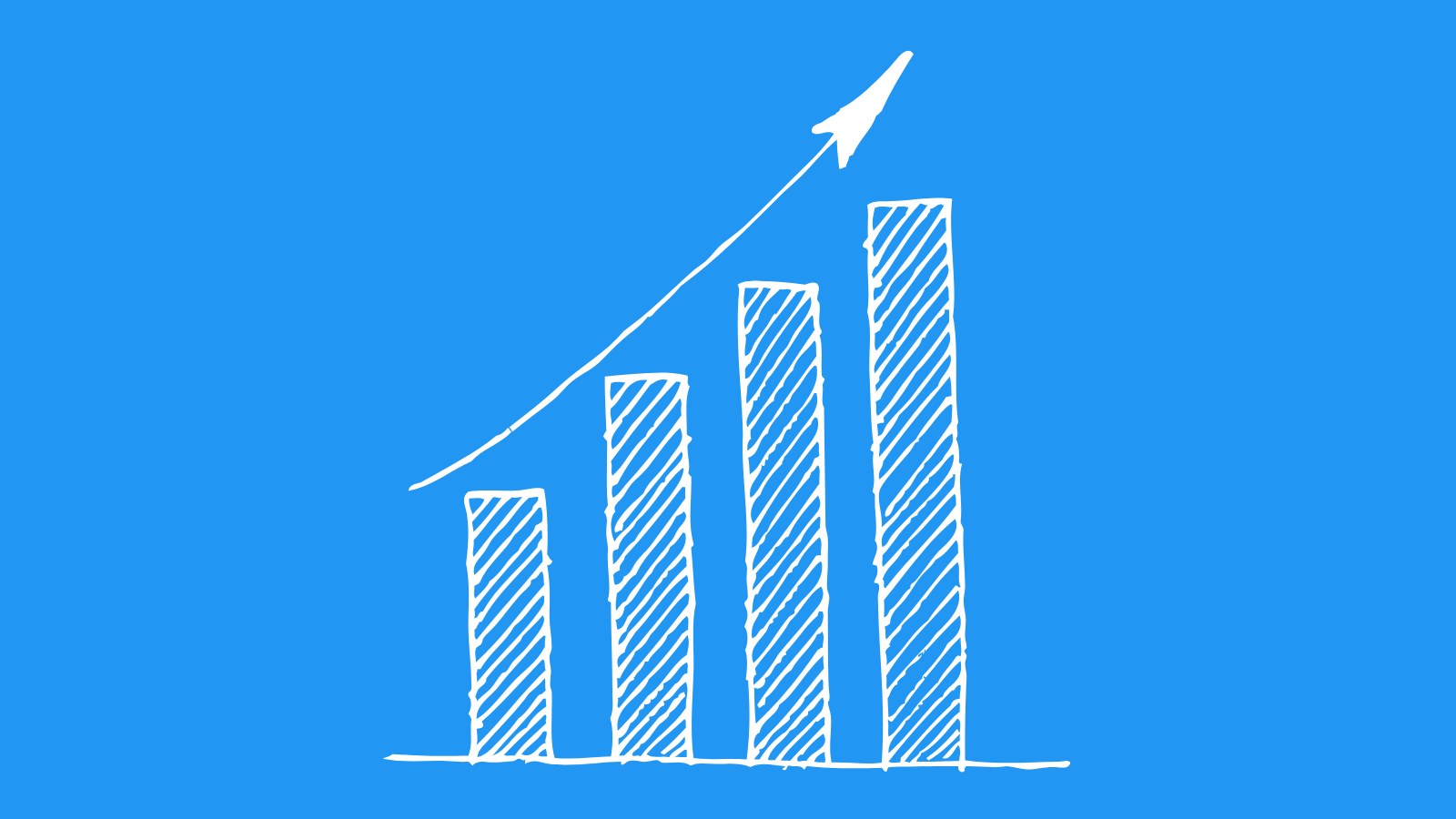
Predicting KBR Stock Movements: A Machine Learning Approach
To construct a robust machine learning model for predicting KBR Inc. stock movements, we would leverage a combination of historical stock data, relevant economic indicators, and company-specific information. Our approach involves several key steps. First, we will gather a comprehensive dataset encompassing historical KBR stock prices, trading volume, and relevant financial metrics. This dataset will serve as the foundation for training our model. Second, we will incorporate external economic indicators such as interest rates, inflation, and oil prices, recognizing their potential impact on KBR's operations and stock performance. Finally, we will analyze KBR's financial reports, press releases, and other relevant information to identify key drivers of its stock price fluctuations. This data will allow us to develop a predictive model that incorporates both macro- and microeconomic factors.
We will employ a variety of machine learning algorithms to develop our predictive model, including but not limited to: (1) **Regression models**, such as linear regression or support vector regression, to establish relationships between input variables and stock price changes. (2) **Recurrent neural networks (RNNs)**, which are particularly adept at capturing time-series dependencies within the stock data. (3) **Long Short-Term Memory (LSTM) networks**, which can handle complex temporal patterns and are suitable for predicting future stock price movements based on historical data. The specific algorithm chosen will depend on the characteristics of the data and the desired level of prediction accuracy.
Our model will be rigorously tested and validated using a combination of techniques, including cross-validation and backtesting. This process will ensure the model's robustness and ability to generalize to future data. We will continuously monitor the performance of our model and make necessary adjustments based on the evolving market conditions and any changes in KBR's business environment. Our goal is to provide KBR Inc. with a powerful tool for anticipating stock price movements, enabling informed decision-making and potentially enhancing investment returns.
ML Model Testing
n:Time series to forecast
p:Price signals of KBR stock
j:Nash equilibria (Neural Network)
k:Dominated move of KBR stock holders
a:Best response for KBR target price
For further technical information as per how our model work we invite you to visit the article below:
How do KappaSignal algorithms actually work?
KBR Stock Forecast (Buy or Sell) Strategic Interaction Table
Strategic Interaction Table Legend:
X axis: *Likelihood% (The higher the percentage value, the more likely the event will occur.)
Y axis: *Potential Impact% (The higher the percentage value, the more likely the price will deviate.)
Z axis (Grey to Black): *Technical Analysis%
KBR's Financial Outlook: Poised for Growth with Navigable Challenges
KBR's financial outlook is positive, driven by its strong backlog and diverse portfolio of government and commercial contracts. The company benefits from its robust position in key growth sectors like energy transition and national security. KBR's expertise in engineering, construction, and technology positions it well to capitalize on the increasing demand for sustainable solutions and defense modernization. A sizable backlog provides KBR with a strong foundation for future revenue generation and sustained growth. The company's geographical diversification across North America, the Middle East, and other regions further enhances its revenue stream and mitigates regional risks.
KBR faces challenges related to global economic uncertainty, supply chain disruptions, and intense competition in its key markets. While the company's focus on high-value projects and its diversified revenue streams provide a buffer against these headwinds, careful management of costs and resources will be crucial to maintain profitability.
Looking ahead, KBR is expected to benefit from the global push towards clean energy solutions and the increasing demand for infrastructure development. The company's focus on sustainable technologies and its strong track record in large-scale projects position it well to capitalize on these trends. Furthermore, KBR's expansion into new markets and its strategic acquisitions are expected to drive further growth and enhance its competitive position.
In summary, KBR's financial outlook is positive, supported by its robust backlog, diverse portfolio, and strong market position. While the company faces challenges related to global economic uncertainty and industry competition, its focus on high-value projects, sustainable technologies, and strategic expansion are expected to drive continued growth and profitability.
Rating | Short-Term | Long-Term Senior |
---|---|---|
Outlook | B2 | B1 |
Income Statement | Baa2 | C |
Balance Sheet | B1 | Baa2 |
Leverage Ratios | Caa2 | B1 |
Cash Flow | C | Baa2 |
Rates of Return and Profitability | B1 | Caa2 |
*Financial analysis is the process of evaluating a company's financial performance and position by neural network. It involves reviewing the company's financial statements, including the balance sheet, income statement, and cash flow statement, as well as other financial reports and documents.
How does neural network examine financial reports and understand financial state of the company?
References
- S. Bhatnagar, R. Sutton, M. Ghavamzadeh, and M. Lee. Natural actor-critic algorithms. Automatica, 45(11): 2471–2482, 2009
- Hill JL. 2011. Bayesian nonparametric modeling for causal inference. J. Comput. Graph. Stat. 20:217–40
- J. Ott. A Markov decision model for a surveillance application and risk-sensitive Markov decision processes. PhD thesis, Karlsruhe Institute of Technology, 2010.
- Künzel S, Sekhon J, Bickel P, Yu B. 2017. Meta-learners for estimating heterogeneous treatment effects using machine learning. arXiv:1706.03461 [math.ST]
- S. J. Russell and A. Zimdars. Q-decomposition for reinforcement learning agents. In Machine Learning, Proceedings of the Twentieth International Conference (ICML 2003), August 21-24, 2003, Washington, DC, USA, pages 656–663, 2003.
- Chernozhukov V, Chetverikov D, Demirer M, Duflo E, Hansen C, et al. 2018a. Double/debiased machine learning for treatment and structural parameters. Econom. J. 21:C1–68
- Ruiz FJ, Athey S, Blei DM. 2017. SHOPPER: a probabilistic model of consumer choice with substitutes and complements. arXiv:1711.03560 [stat.ML]