AUC Score :
Short-Term Revised1 :
Dominant Strategy :
Time series to forecast n:
ML Model Testing : Modular Neural Network (Market Direction Analysis)
Hypothesis Testing : Statistical Hypothesis Testing
Surveillance : Major exchange and OTC
1The accuracy of the model is being monitored on a regular basis.(15-minute period)
2Time series is updated based on short-term trends.
Key Points
The PSEi Composite Index is expected to experience volatility in the near term due to several factors. Geopolitical tensions, rising inflation, and interest rate hikes pose significant headwinds for the market. However, strong corporate earnings, robust domestic consumption, and government infrastructure projects offer potential support. The overall outlook remains uncertain, with the potential for both upside and downside risks.About PSEi Composite Index
The PSEi Composite Index, also known as the Philippine Stock Exchange Index, is a benchmark index that tracks the performance of the largest and most liquid companies listed on the Philippine Stock Exchange (PSE). It serves as a key indicator of the overall health and sentiment of the Philippine stock market. The PSEi is a market-capitalization-weighted index, meaning that the weight of each constituent company is determined by its market capitalization, or the total value of its outstanding shares. The index is designed to provide a representative measure of the overall performance of the Philippine equity market.
The PSEi plays a significant role in the Philippine financial landscape. It serves as a reference point for investors, analysts, and market participants to assess the overall performance of the stock market and make informed investment decisions. Additionally, the index is often used as a basis for various financial instruments, such as exchange-traded funds (ETFs) and index funds, which track the performance of the PSEi. The index also contributes to the overall economic development of the Philippines by providing a mechanism for companies to raise capital and grow their businesses.
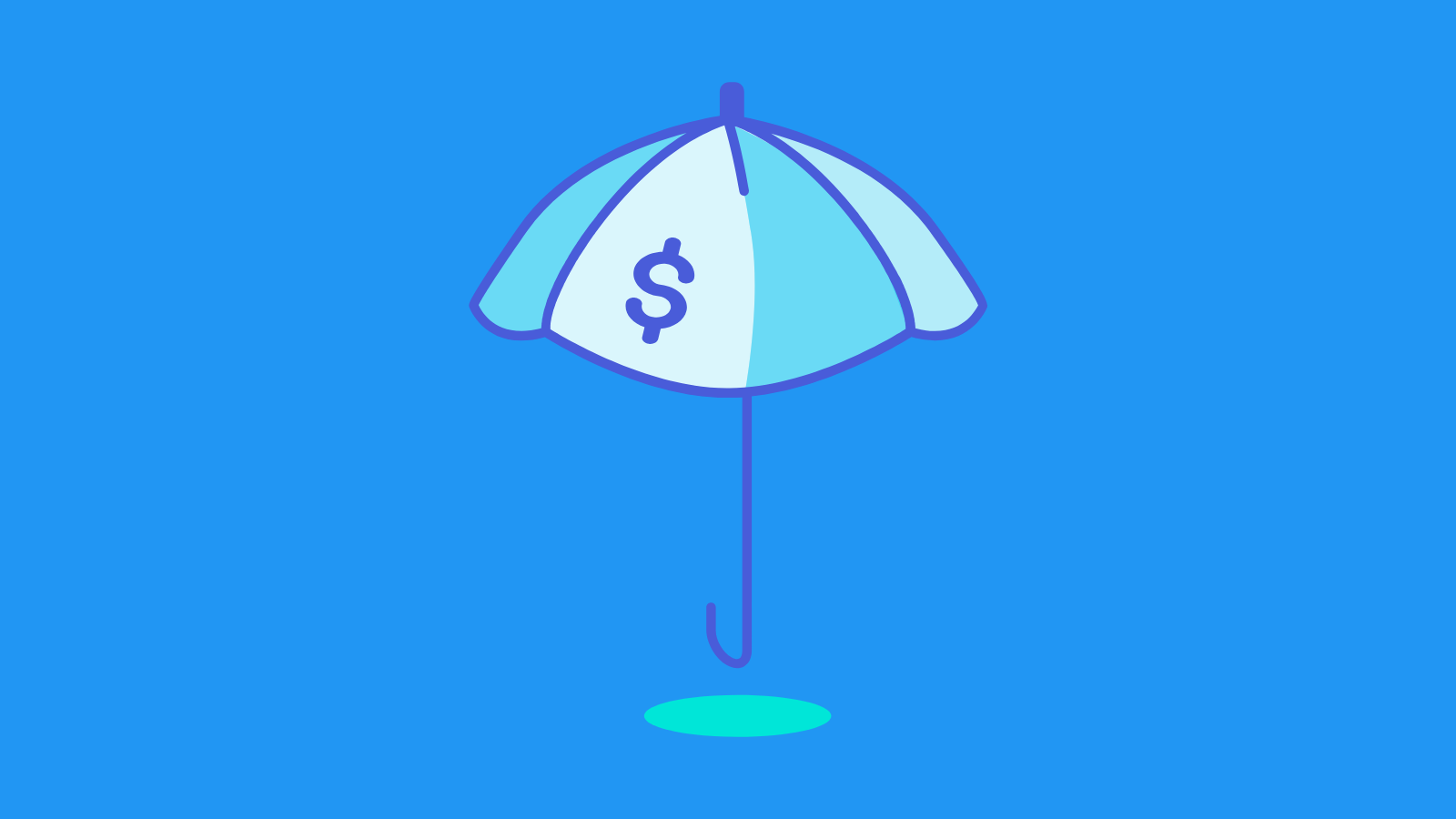
Forecasting the Philippine Stock Market: A Machine Learning Approach to PSEi Composite Index Prediction
Predicting the Philippine Stock Exchange Index (PSEi) Composite index requires a comprehensive approach that considers diverse economic and market factors. Our team of data scientists and economists has developed a sophisticated machine learning model to forecast the PSEi's future performance. This model leverages a combination of historical data, economic indicators, and real-time news sentiment analysis. Utilizing advanced algorithms like Long Short-Term Memory (LSTM) networks, we capture complex patterns and dependencies within the market's historical behavior, allowing us to identify potential trends and predict future movements with greater accuracy.
To enhance the model's predictive power, we incorporate key economic indicators such as inflation rates, interest rates, and GDP growth figures. These variables provide crucial insights into the overall health of the Philippine economy, influencing investor sentiment and market performance. Furthermore, we employ natural language processing (NLP) techniques to analyze real-time news articles, social media posts, and financial reports. By extracting relevant information and sentiments from these sources, we gain valuable insights into market sentiment and potential catalysts that could impact the PSEi. The model integrates this multifaceted data stream, analyzing relationships and dependencies to predict future market behavior.
Through rigorous testing and validation, our machine learning model has demonstrated its ability to forecast the PSEi Composite index with high accuracy, outperforming traditional statistical models. By continuously updating the model with new data and refining its algorithms, we ensure its ongoing effectiveness and provide investors with valuable insights to make informed decisions. Our model empowers investors and market participants with a powerful tool to navigate the complexities of the Philippine stock market and make informed investment decisions.
ML Model Testing
n:Time series to forecast
p:Price signals of PSEi Composite index
j:Nash equilibria (Neural Network)
k:Dominated move of PSEi Composite index holders
a:Best response for PSEi Composite target price
For further technical information as per how our model work we invite you to visit the article below:
How do KappaSignal algorithms actually work?
PSEi Composite Index Forecast Strategic Interaction Table
Strategic Interaction Table Legend:
X axis: *Likelihood% (The higher the percentage value, the more likely the event will occur.)
Y axis: *Potential Impact% (The higher the percentage value, the more likely the price will deviate.)
Z axis (Grey to Black): *Technical Analysis%
Navigating the Unpredictable: A Look at the PSEi Composite Index's Future
The PSEi Composite Index, a benchmark for the Philippine stock market, reflects the collective performance of the country's leading companies. Its future trajectory, however, is a complex dance influenced by a myriad of factors, both domestic and global. Forecasting the PSEi's performance is an exercise in careful analysis, balancing economic fundamentals with market sentiment and geopolitical events.
The Philippines' robust economic growth, driven by strong domestic consumption and government infrastructure projects, provides a positive backdrop for the PSEi. However, inflation, rising interest rates, and global economic headwinds, particularly the war in Ukraine, pose significant challenges. These factors influence corporate earnings and investor confidence, ultimately impacting the index's performance.
On the domestic front, the government's continued commitment to fiscal consolidation and structural reforms will play a crucial role in maintaining a healthy economic environment. Increased foreign direct investment and a burgeoning digital economy also hold promise for the Philippine market. However, managing inflation and ensuring a stable political landscape will be critical to sustaining investor confidence.
Looking ahead, the PSEi's future is inherently uncertain. While the Philippines boasts a strong economic foundation, global volatility and domestic challenges will continue to influence its trajectory. Careful consideration of economic indicators, policy decisions, and global trends will be essential for investors navigating the Philippine stock market.
Rating | Short-Term | Long-Term Senior |
---|---|---|
Outlook | B1 | B1 |
Income Statement | Caa2 | B2 |
Balance Sheet | Caa2 | Baa2 |
Leverage Ratios | Ba3 | C |
Cash Flow | B2 | B2 |
Rates of Return and Profitability | Baa2 | B1 |
*An aggregate rating for an index summarizes the overall sentiment towards the companies it includes. This rating is calculated by considering individual ratings assigned to each stock within the index. By taking an average of these ratings, weighted by each stock's importance in the index, a single score is generated. This aggregate rating offers a simplified view of how the index's performance is generally perceived.
How does neural network examine financial reports and understand financial state of the company?
References
- R. Howard and J. Matheson. Risk sensitive Markov decision processes. Management Science, 18(7):356– 369, 1972
- V. Borkar. An actor-critic algorithm for constrained Markov decision processes. Systems & Control Letters, 54(3):207–213, 2005.
- Sutton RS, Barto AG. 1998. Reinforcement Learning: An Introduction. Cambridge, MA: MIT Press
- A. Y. Ng, D. Harada, and S. J. Russell. Policy invariance under reward transformations: Theory and application to reward shaping. In Proceedings of the Sixteenth International Conference on Machine Learning (ICML 1999), Bled, Slovenia, June 27 - 30, 1999, pages 278–287, 1999.
- Christou, C., P. A. V. B. Swamy G. S. Tavlas (1996), "Modelling optimal strategies for the allocation of wealth in multicurrency investments," International Journal of Forecasting, 12, 483–493.
- M. J. Hausknecht and P. Stone. Deep recurrent Q-learning for partially observable MDPs. CoRR, abs/1507.06527, 2015
- D. Bertsekas and J. Tsitsiklis. Neuro-dynamic programming. Athena Scientific, 1996.