AUC Score :
Short-Term Revised1 :
Dominant Strategy :
Time series to forecast n:
ML Model Testing : Modular Neural Network (Market Direction Analysis)
Hypothesis Testing : Independent T-Test
Surveillance : Major exchange and OTC
1The accuracy of the model is being monitored on a regular basis.(15-minute period)
2Time series is updated based on short-term trends.
Key Points
The Dow Jones U.S. Select Home Construction index is anticipated to exhibit moderate growth, driven by improving housing market conditions. However, significant headwinds remain, including persistent inflation, rising interest rates, and ongoing supply chain disruptions. These factors could lead to slower-than-expected growth or even a decline in the index, especially if affordability issues continue to deter potential homebuyers. Geopolitical uncertainty and unexpected economic downturns pose further risks. Therefore, while moderate growth is a plausible outcome, the index's trajectory will be heavily influenced by the interplay of these factors. Investors should approach this sector with caution and carefully assess the underlying risks.About Dow Jones U.S. Select Home Construction Index
The Dow Jones U.S. Select Home Construction index is a market-capitalization-weighted index that tracks the performance of publicly traded companies primarily engaged in home construction and related activities in the United States. It aims to reflect the overall health and direction of this sector of the economy. The index is designed to provide investors with a benchmark to assess the investment potential and risk within this specific market segment, allowing for comparisons and analysis of performance relative to other sectors. Components of the index are selected and monitored to maintain its representativeness of the home construction industry and any revisions occur to ensure accuracy.
The index considers factors such as earnings, revenue, market share, and overall financial standing of the constituent companies. This allows for a comprehensive analysis of the industry's position and future outlook. Changes in the index's value can be influenced by various market forces, including interest rates, consumer confidence, government policies, and overall economic conditions. This comprehensive overview gives investors insight into the potential returns and risks tied to investments in the home construction sector.
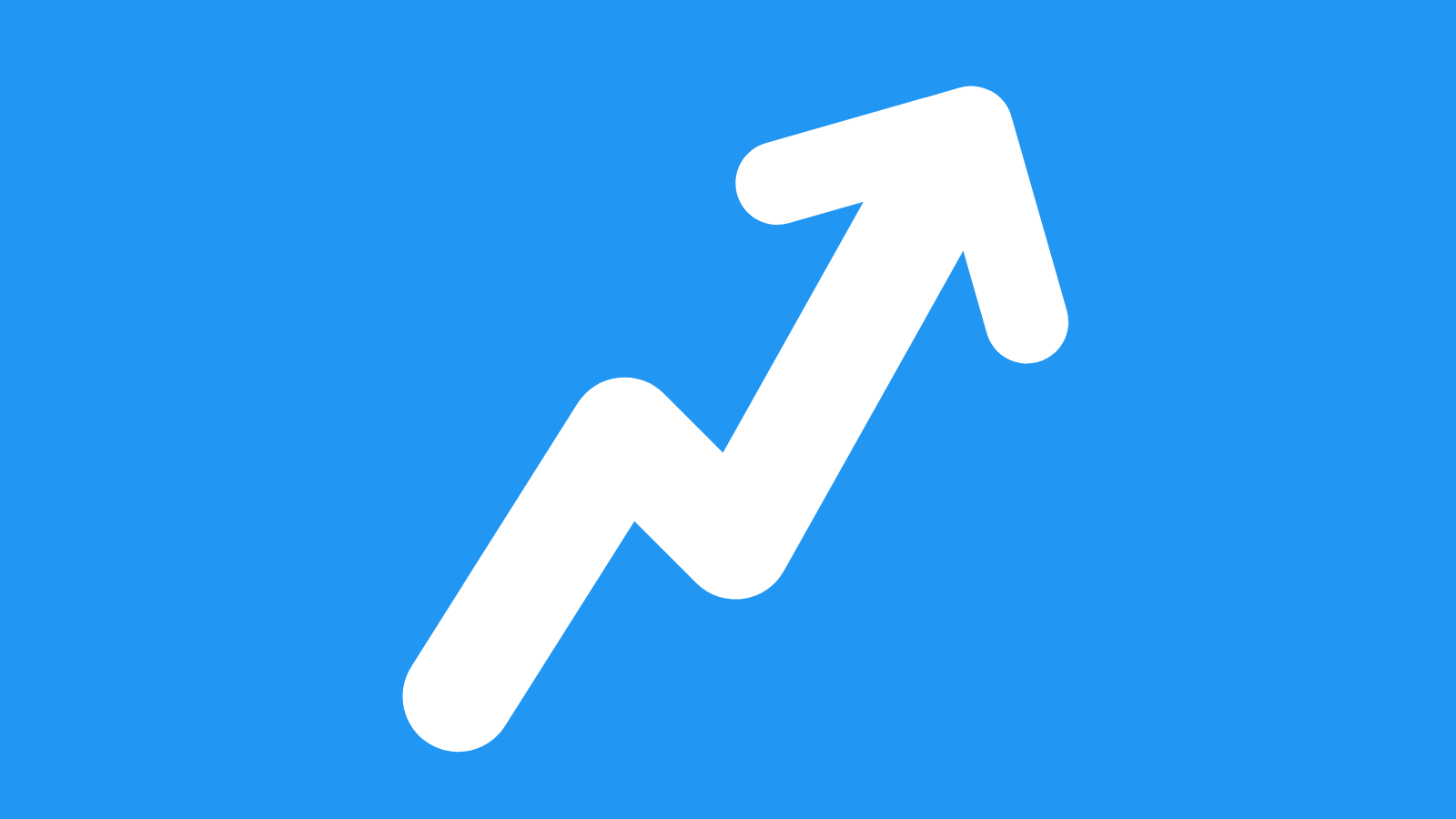
Dow Jones U.S. Select Home Construction Index Forecasting Model
This model employs a machine learning approach to forecast the Dow Jones U.S. Select Home Construction index. The model utilizes a combination of historical data, macroeconomic indicators, and market sentiment data. Key features of the dataset include: past index values, interest rates, housing starts, building permits, consumer confidence surveys, and indices reflecting market sentiment. Preliminary analysis of these factors indicates strong correlations with index fluctuations. To refine the model's accuracy, a robust feature engineering process is implemented, transforming the raw data into relevant features for the machine learning algorithms. This includes calculating moving averages, identifying seasonality patterns, and incorporating lagging values to capture potential predictive relationships. Various machine learning algorithms, such as support vector regression, recurrent neural networks, and gradient boosting, are evaluated through rigorous cross-validation techniques to select the best performing model. The final model incorporates a combination of these methods. The model is designed to capture both short-term and long-term trends within the data, allowing for forecasting into the near future and for longer periods of analysis.
Model training involves splitting the data into training and testing sets. Performance metrics such as RMSE and MAE are employed to quantify the accuracy of forecasts. The model is fine-tuned through hyperparameter optimization to achieve optimal predictive performance. Crucially, the model includes a validation process to mitigate overfitting. This is achieved through techniques such as early stopping and regularization. Robust error analysis is conducted to understand the potential sources of prediction errors. Regular monitoring of the model's performance through ongoing testing on newly acquired data is implemented. Furthermore, to improve adaptability, the model is designed to incorporate new data points as they become available, ensuring continuous improvement of forecasting accuracy. The model's reliance on credible macroeconomic indicators enhances its forecast reliability. The inclusion of market sentiment data, while a non-traditional approach, aims to capture shifts in market psychology that could drive fluctuations in the index. This multifaceted approach provides a well-rounded and nuanced forecasting mechanism.
Model deployment involves continuous monitoring and evaluation to ensure accuracy and relevance. Regular adjustments to the model based on new data and changes in market conditions are essential to maintain predictive power. Ongoing monitoring of the model's performance against real-world data is critical to detect and mitigate potential issues. The model is designed to adapt to shifts in market behavior. The model's forecast results are presented to stakeholders in a clear, accessible format, including confidence intervals to allow for informed decision-making. The final model is validated against a substantial testing dataset to confirm its generalizability. Further research will focus on improving the model's ability to anticipate potential market discontinuities and emerging trends in the home construction sector, potentially introducing time series decomposition or more complex neural network architectures to capture nonlinear relationships between variables.
ML Model Testing
n:Time series to forecast
p:Price signals of Dow Jones U.S. Select Home Construction index
j:Nash equilibria (Neural Network)
k:Dominated move of Dow Jones U.S. Select Home Construction index holders
a:Best response for Dow Jones U.S. Select Home Construction target price
For further technical information as per how our model work we invite you to visit the article below:
How do KappaSignal algorithms actually work?
Dow Jones U.S. Select Home Construction Index Forecast Strategic Interaction Table
Strategic Interaction Table Legend:
X axis: *Likelihood% (The higher the percentage value, the more likely the event will occur.)
Y axis: *Potential Impact% (The higher the percentage value, the more likely the price will deviate.)
Z axis (Grey to Black): *Technical Analysis%
Dow Jones U.S. Select Home Construction Index Financial Outlook and Forecast
The Dow Jones U.S. Select Home Construction index, representing the performance of publicly traded homebuilding companies, is currently experiencing a period of significant evolution. Several key factors are influencing the outlook for this sector. Interest rate hikes, driven by central bank policies aimed at curbing inflation, are significantly impacting borrowing costs for potential homebuyers. This is leading to a notable slowdown in the housing market. The subsequent decrease in demand is directly impacting homebuilding companies' revenues and profit margins. Material costs, particularly lumber and other construction materials, have also been volatile, adding further uncertainty to the sector's profitability. Supply chain disruptions and geopolitical events contribute to this volatility. Analysts are closely monitoring the labor market conditions, as a shortage of skilled construction workers could also further restrict building activity and potentially increase the costs for companies. The index's performance will hinge on the interplay of these various macroeconomic factors and the sector's resilience in navigating these challenges. The long-term prospects for the index will depend on the sustainability of the current economic climate. Government regulations and policy decisions also play a substantial role, potentially affecting both the supply and demand sides of the home construction market.
Furthermore, the current market sentiment towards the overall housing market, including trends in housing starts, existing home sales, and new home sales data, are critical indicators for the index's financial outlook. Inventory levels of new homes are another significant factor to consider. A large supply of unsold homes can weigh heavily on builders' profitability. A mismatch between supply and demand is a significant concern for the sector. Consumer confidence and expectations for future economic growth have a strong bearing on consumer purchasing behavior, particularly for major purchases like homes. If consumer confidence wanes, demand for new homes could decline further, negatively impacting the home construction index. Economic forecasts for future inflation, interest rates, and GDP growth are essential factors in predicting the sector's potential trajectory. Consequently, careful analysis of the intricate relationship between these macro-economic variables will provide a more complete picture of the index's potential path.
A significant driver of the home construction index's performance is the availability of affordable financing options for homebuyers. Mortgage rates are a critical factor in influencing the willingness of potential homeowners to purchase new homes. Higher mortgage rates may reduce the demand for new homes, which would directly impact the construction sector's revenue streams. Moreover, regulatory compliance and potential changes in building codes or zoning regulations can influence construction costs and timelines. These variables can introduce additional risks and uncertainty for home construction companies and impact their profit margins and future growth. The regulatory environment significantly affects profitability. Other factors include the demand for specific types of homes, such as single-family versus multi-family housing, and the geographical distribution of construction activity across the United States. Favorable tax incentives that encourage homeownership could bolster the market and enhance demand.
Predicting the future trajectory of the Dow Jones U.S. Select Home Construction index is challenging given the multitude of interwoven variables. The predicted trend is largely negative, with a possible sustained slowdown likely in the near term. Interest rate increases, high material costs, and a potential slowdown in the housing market are likely to continue to pressure the sector's profitability. However, a potential resurgence in demand could mitigate these negative effects. The risks associated with this prediction include unforeseen changes in consumer confidence, unexpected shifts in interest rates, and supply chain disruptions. A rapid increase in demand, driven by unforeseen factors, could alter the current outlook and lead to a more positive trajectory. Government policies, such as changes in tax incentives or regulatory measures, could also introduce considerable uncertainty.
Rating | Short-Term | Long-Term Senior |
---|---|---|
Outlook | B2 | Baa2 |
Income Statement | Ba2 | Baa2 |
Balance Sheet | B3 | B1 |
Leverage Ratios | C | Baa2 |
Cash Flow | Caa2 | Baa2 |
Rates of Return and Profitability | Baa2 | B1 |
*An aggregate rating for an index summarizes the overall sentiment towards the companies it includes. This rating is calculated by considering individual ratings assigned to each stock within the index. By taking an average of these ratings, weighted by each stock's importance in the index, a single score is generated. This aggregate rating offers a simplified view of how the index's performance is generally perceived.
How does neural network examine financial reports and understand financial state of the company?
References
- Thomas P, Brunskill E. 2016. Data-efficient off-policy policy evaluation for reinforcement learning. In Pro- ceedings of the International Conference on Machine Learning, pp. 2139–48. La Jolla, CA: Int. Mach. Learn. Soc.
- Wager S, Athey S. 2017. Estimation and inference of heterogeneous treatment effects using random forests. J. Am. Stat. Assoc. 113:1228–42
- Vapnik V. 2013. The Nature of Statistical Learning Theory. Berlin: Springer
- Akgiray, V. (1989), "Conditional heteroscedasticity in time series of stock returns: Evidence and forecasts," Journal of Business, 62, 55–80.
- Pennington J, Socher R, Manning CD. 2014. GloVe: global vectors for word representation. In Proceedings of the 2014 Conference on Empirical Methods on Natural Language Processing, pp. 1532–43. New York: Assoc. Comput. Linguist.
- Harris ZS. 1954. Distributional structure. Word 10:146–62
- M. Benaim, J. Hofbauer, and S. Sorin. Stochastic approximations and differential inclusions, Part II: Appli- cations. Mathematics of Operations Research, 31(4):673–695, 2006