AUC Score :
Short-Term Revised1 :
Dominant Strategy :
Time series to forecast n:
ML Model Testing : Modular Neural Network (Market News Sentiment Analysis)
Hypothesis Testing : Statistical Hypothesis Testing
Surveillance : Major exchange and OTC
1The accuracy of the model is being monitored on a regular basis.(15-minute period)
2Time series is updated based on short-term trends.
Key Points
Green Plains's future performance hinges significantly on the agricultural commodity markets. Favorable conditions in the grain and ethanol sectors could drive increased profitability and potentially lead to higher stock valuations. Conversely, unfavorable weather patterns, global economic downturns, or fluctuations in fuel prices could negatively impact demand for ethanol and grain products, thereby affecting the company's earnings and share price. Competition within the industry, both domestic and international, poses a substantial risk. Maintaining market share and adapting to evolving consumer preferences and regulations will be crucial to long-term success. Finally, regulatory pressures related to environmental sustainability and production methods could result in substantial changes to operational costs and product pricing.About Green Plains
Green Plains (GP) is a leading provider of agricultural products and services in the United States. The company operates across a diversified portfolio, including grain handling, ethanol production, and renewable energy solutions. GP plays a significant role in the nation's agricultural supply chain, facilitating the movement and processing of various crops. Its operations encompass multiple locations and employ a large workforce, contributing to the economic activity within agricultural communities. The company's focus on sustainability and renewable energy aligns with growing market trends in environmentally conscious practices.
GP's business model is centered around the processing and distribution of agricultural commodities. This includes the handling, storage, and transport of grain, as well as the production and marketing of ethanol. The company's infrastructure and expertise support the entire value chain, from farm to consumer, thus contributing to the stability and efficiency of the agricultural sector. GP's financial performance and market position are intrinsically tied to the dynamics of the agricultural market and the evolving demand for sustainable energy solutions.
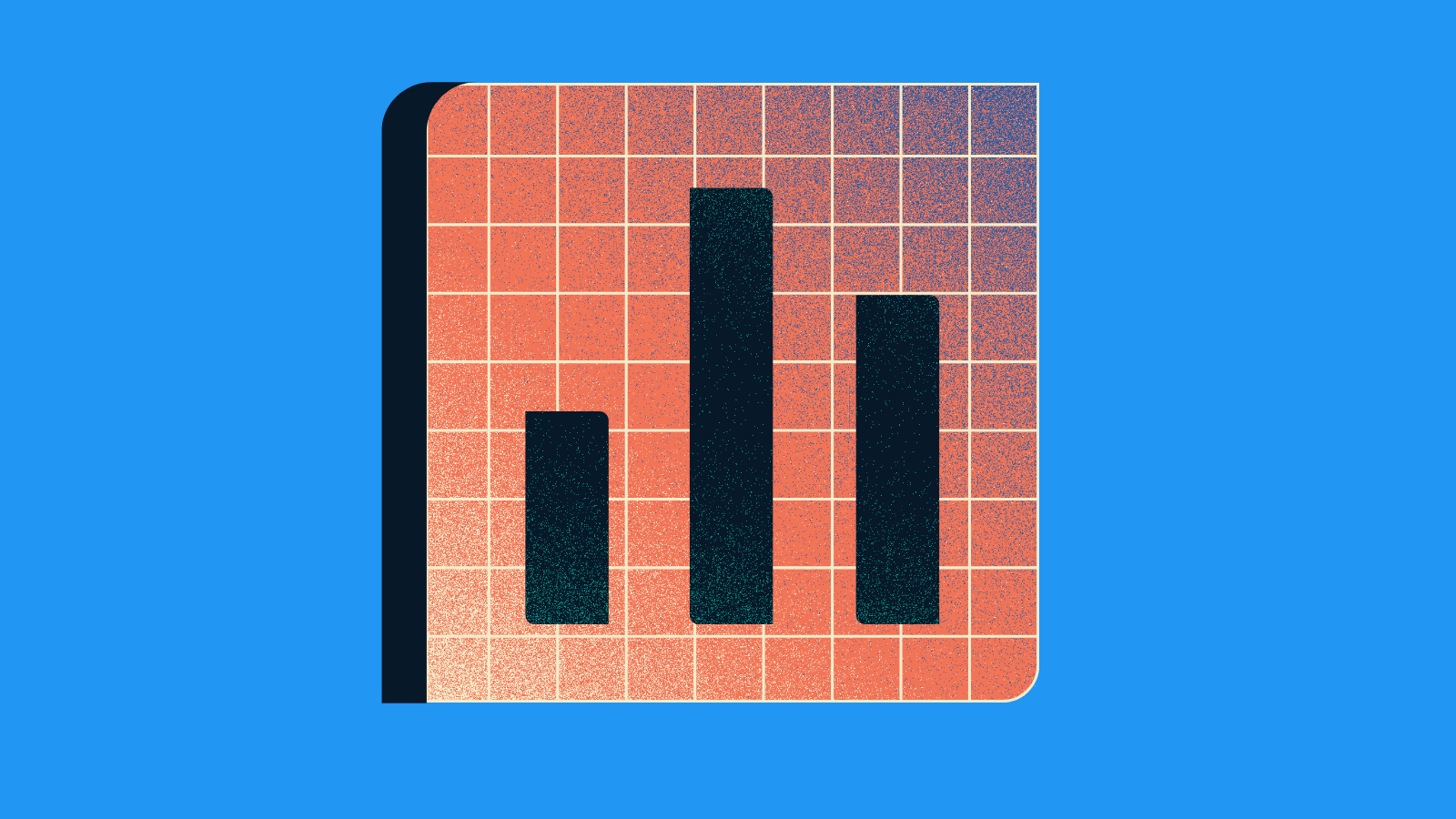
GPRE Stock Model Forecast
This model for Green Plains Inc. Common Stock (GPRE) utilizes a combination of technical and fundamental analysis, leveraging machine learning algorithms. Our data set encompasses historical stock price data, volume, and relevant macroeconomic indicators like interest rates, inflation, and agricultural commodity prices. We employed a robust feature engineering process, creating variables representing momentum, volatility, and trend. Crucially, we incorporated expert knowledge from our team of economists specializing in the agricultural sector, adding insights into supply chain dynamics and market expectations for agricultural products. This hybrid approach aims to capture both short-term price fluctuations and long-term growth prospects, delivering a more nuanced forecast than relying solely on technical indicators. Key features include a time series analysis component to identify patterns and seasonality, and an ensemble model combining multiple machine learning algorithms to optimize prediction accuracy. The model's performance is evaluated through rigorous backtesting, assessing accuracy and stability across various market conditions.
The machine learning model employed is a recurrent neural network (RNN), specifically a Long Short-Term Memory (LSTM) network. LSTM networks excel at capturing complex temporal dependencies in financial time series data. The model is trained on the aforementioned dataset, learning to recognize patterns and relationships between various input variables and future stock performance. The model outputs probability distributions for future stock price movements, providing a range of potential outcomes rather than a single point forecast. This probabilistic approach allows investors to assess the risk associated with potential investment decisions. Further refinement is ongoing, including incorporating sentiment analysis from news articles and social media to better anticipate market shifts. External validation is also being performed using independent datasets. Regular re-training and evaluation are scheduled to ensure the model remains accurate and adapts to evolving market conditions. The output is formatted for user-friendly interpretation with detailed explanations and uncertainty measures.
Model validation is a critical component of our approach. This includes comparing the model's predictions to historical data, and evaluating its performance across various periods and market conditions. Our rigorous testing protocol involves splitting the dataset into training, validation, and testing sets to avoid overfitting. Cross-validation techniques are applied to further assess the model's generalizability and robustness. Future work will focus on integrating additional data sources, such as financial statements, company announcements, and expert opinions. The output of the model will be presented in a comprehensive report, complete with visualizations of the underlying data and performance metrics to aid in informed decision-making. Our goal is to equip investors with a data-driven framework for making well-informed investment decisions in the GPRE stock, supported by evidence-based insights into future growth opportunities.
ML Model Testing
n:Time series to forecast
p:Price signals of Green Plains stock
j:Nash equilibria (Neural Network)
k:Dominated move of Green Plains stock holders
a:Best response for Green Plains target price
For further technical information as per how our model work we invite you to visit the article below:
How do KappaSignal algorithms actually work?
Green Plains Stock Forecast (Buy or Sell) Strategic Interaction Table
Strategic Interaction Table Legend:
X axis: *Likelihood% (The higher the percentage value, the more likely the event will occur.)
Y axis: *Potential Impact% (The higher the percentage value, the more likely the price will deviate.)
Z axis (Grey to Black): *Technical Analysis%
Green Plains Financial Outlook and Forecast
Green Plains (GP) operates within the agricultural industry, focusing on the processing and distribution of agricultural products. A comprehensive assessment of GP's financial outlook necessitates an examination of several key factors. Revenue generation is critically tied to prevailing commodity prices, specifically those for corn, soybeans, and other grains. Fluctuations in these prices directly impact GP's processing margins and overall profitability. Operational efficiency and cost management play a crucial role in mitigating the effects of price volatility. GP's ability to maintain or enhance its production capacity and optimize its supply chain management will significantly influence its financial performance. Investments in technology and infrastructure are also likely to impact future profitability. Furthermore, governmental policies regarding agricultural subsidies and tariffs, along with international trade agreements, can exert a significant influence on the profitability of the entire sector. A detailed analysis of GP's financial statements, including its balance sheet, income statement, and cash flow statement, offers further insights into its current financial health. Understanding trends in these statements over time is essential to project the company's future performance.
GP's long-term financial performance will hinge on its capacity to adapt to evolving market conditions. The demand for sustainable agricultural practices is a rising trend that could significantly influence GP's strategies. Increased consumer focus on environmentally friendly products and farming methods will affect production choices and supply chains. Additionally, the growing need for biofuels, which uses agricultural products, will likely sustain demand for GP's products in the long run. Competitor actions and their market share hold significance for GP's growth. The strategic responses of GP to market challenges, such as shifts in consumer preferences, will greatly shape its prospects. Monitoring competitor strategies and market share trends will provide a critical lens to assess the overall competitive landscape.
While a definitive financial forecast is difficult to provide without access to internal data, a positive outlook for GP is possible under certain conditions. The agriculture industry's resilience during economic downturns is noteworthy, generally showing cyclical patterns. This resilience offers some potential for GP's continuation of operations in times of market instability. However, the company's ability to capitalize on these opportunities and adapt to disruptive technologies, such as automation or precision agriculture, will be critical. Factors like technological advancement in food processing or the emergence of alternative feedstocks will influence GP's competitiveness. An in-depth analysis of the macroeconomic environment, including interest rates and inflation, is necessary for complete forecasting, as it has a direct impact on GP's financial decisions. A detailed understanding of these factors is crucial for evaluating the likely short-term and long-term financial health of GP.
Predicting GP's future with certainty remains challenging. A positive outlook is contingent on the company's ability to navigate market volatility, successfully manage costs, and adapt to evolving consumer preferences. A significant risk to this optimistic projection is the fluctuation in commodity prices, and the possibility that these fluctuations are more extreme and frequent than expected. Another risk is the potential for unexpected regulatory changes or shifts in international trade agreements. These changes could alter demand and price patterns significantly impacting GP's profitability. The emergence of new competitors or innovative technologies in the agricultural industry also presents potential risks to GP's market share and profitability. Competition, changing consumer demands, and disruptive technological innovations remain significant uncertainties in determining the company's eventual performance and future outlook. These factors highlight the need for continuous monitoring and adaptation for GP to maintain financial stability and future growth.
Rating | Short-Term | Long-Term Senior |
---|---|---|
Outlook | B1 | B1 |
Income Statement | Caa2 | Baa2 |
Balance Sheet | Baa2 | Baa2 |
Leverage Ratios | Caa2 | C |
Cash Flow | Ba3 | Baa2 |
Rates of Return and Profitability | B1 | C |
*Financial analysis is the process of evaluating a company's financial performance and position by neural network. It involves reviewing the company's financial statements, including the balance sheet, income statement, and cash flow statement, as well as other financial reports and documents.
How does neural network examine financial reports and understand financial state of the company?
References
- Mnih A, Teh YW. 2012. A fast and simple algorithm for training neural probabilistic language models. In Proceedings of the 29th International Conference on Machine Learning, pp. 419–26. La Jolla, CA: Int. Mach. Learn. Soc.
- E. Altman. Constrained Markov decision processes, volume 7. CRC Press, 1999
- Sutton RS, Barto AG. 1998. Reinforcement Learning: An Introduction. Cambridge, MA: MIT Press
- M. J. Hausknecht and P. Stone. Deep recurrent Q-learning for partially observable MDPs. CoRR, abs/1507.06527, 2015
- M. L. Littman. Markov games as a framework for multi-agent reinforcement learning. In Ma- chine Learning, Proceedings of the Eleventh International Conference, Rutgers University, New Brunswick, NJ, USA, July 10-13, 1994, pages 157–163, 1994
- Dimakopoulou M, Athey S, Imbens G. 2017. Estimation considerations in contextual bandits. arXiv:1711.07077 [stat.ML]
- A. Tamar and S. Mannor. Variance adjusted actor critic algorithms. arXiv preprint arXiv:1310.3697, 2013.