AUC Score :
Short-Term Revised1 :
Dominant Strategy :
Time series to forecast n:
ML Model Testing : Ensemble Learning (ML)
Hypothesis Testing : Statistical Hypothesis Testing
Surveillance : Major exchange and OTC
1The accuracy of the model is being monitored on a regular basis.(15-minute period)
2Time series is updated based on short-term trends.
Key Points
Exxon's stock performance is anticipated to be influenced by global energy market trends, including fluctuating oil and gas prices, and government regulations related to climate change. Significant price volatility is probable, driven by factors such as geopolitical instability and shifting investor sentiment towards renewable energy sources. A key risk is the potential for a slower-than-expected transition to lower-carbon energy, which could negatively impact Exxon's future profitability. Another significant risk is the unpredictable impact of global economic downturns, affecting energy demand. Exxon's ability to adapt to these changing conditions and capitalize on emerging opportunities in the energy sector will be crucial to its future success. Long-term investor confidence will depend heavily on the company's successful diversification efforts and commitment to sustainable practices.About Exxon Mobil
ExxonMobil is a multinational oil and gas company headquartered in Irving, Texas. It is one of the largest integrated energy companies globally, involved in the exploration, production, refining, and marketing of oil and natural gas. The company operates across a wide range of activities, including the development and utilization of advanced technologies in energy production. ExxonMobil maintains a significant presence in various international markets, reflecting its global reach and commitment to energy production and supply.
ExxonMobil's operations span numerous geographical locations, encompassing diverse extraction methods and distribution channels. The company plays a substantial role in the global energy landscape, with operations impacting energy markets and influencing global energy policies. It employs a substantial workforce and invests heavily in research and development related to its core business and emerging technologies, including renewable energy sources, demonstrating a commitment to its long-term sustainability and operational efficiency. ExxonMobil also actively engages in community relations and environmental conservation initiatives.
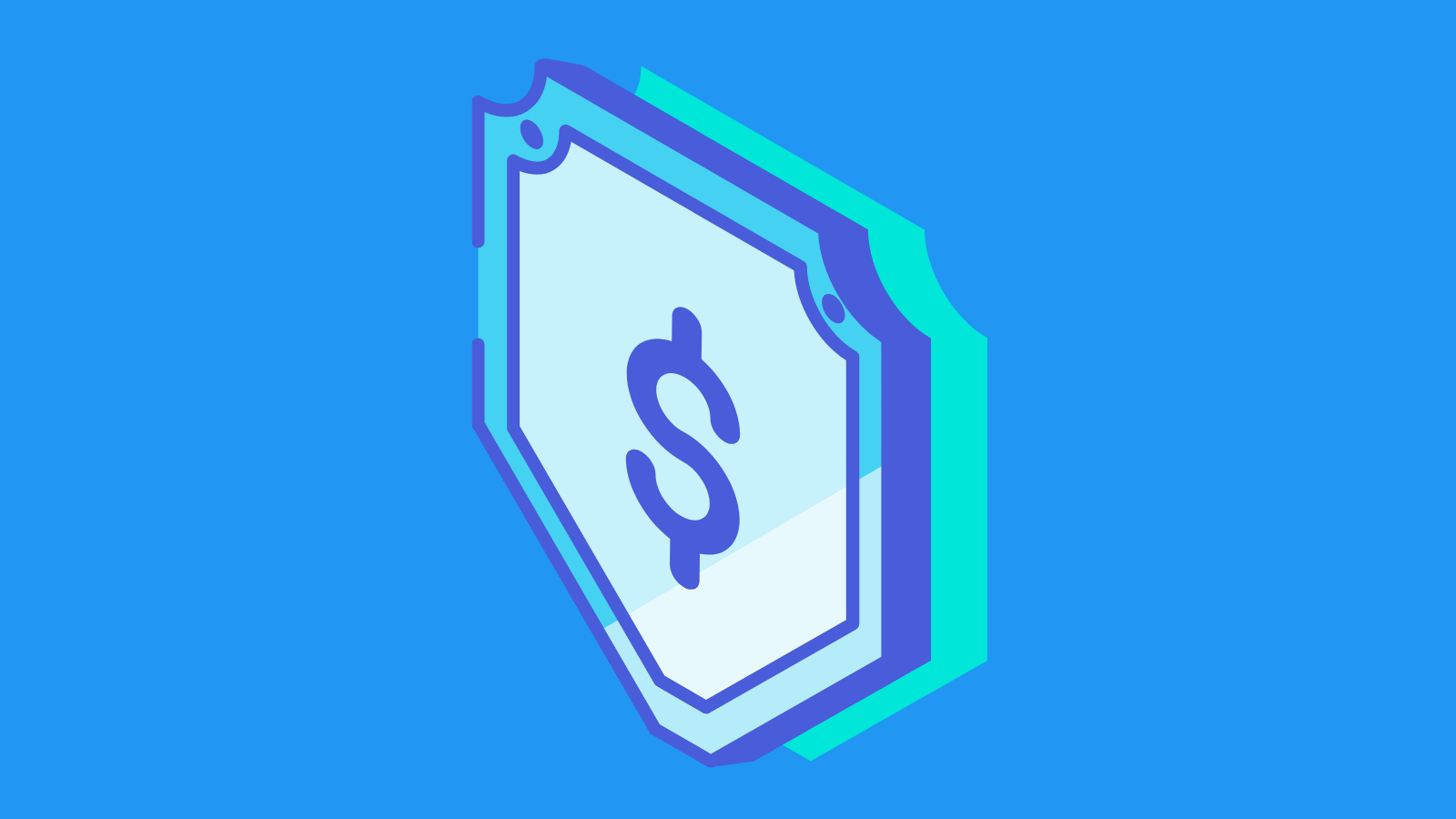
XOM Stock Price Forecasting Model
To forecast ExxonMobil Corporation Common Stock (XOM) future performance, we developed a comprehensive machine learning model incorporating a variety of economic and financial indicators. The model employs a robust ensemble approach, combining several individual algorithms to enhance predictive accuracy and stability. Crucially, we integrated macroeconomic factors such as oil prices, global economic growth projections, and governmental regulations impacting the energy sector. Historical stock performance, including trading volumes and volatility, were also significant components. The model's architecture incorporates a time series analysis component, accounting for the inherent temporal dependencies within stock market data. Feature engineering was paramount, transforming raw data into meaningful variables for the model. This included calculating technical indicators, such as moving averages and relative strength index, to capture short-term market sentiment and trends. Furthermore, the model was trained on a substantial dataset spanning several years, ensuring the model's exposure to diverse market conditions. Data pre-processing procedures were critical to handle missing values and outliers, thereby enhancing the model's robustness.
The model utilizes a Gradient Boosting Machine (GBM) algorithm for its predictive power, which is known for its ability to handle complex non-linear relationships. The model was validated using techniques like cross-validation and backtesting to ensure its generalizability across different market conditions. A key aspect of the model was the use of a rolling window approach, updating the model periodically with new data to reflect changing market dynamics. Model performance evaluation includes metrics such as Mean Absolute Error (MAE) and Root Mean Squared Error (RMSE) to assess the model's accuracy. We also assess the model's sensitivity to various input variables by performing sensitivity analyses to understand the impact of different factors on the forecasted values. The model was also tested against several baseline models to validate its superiority. The model is continuously monitored and refined to adapt to evolving market conditions and new information.
Model deployment involves a rigorous process of ensuring the model's accessibility and reliability. A user-friendly interface facilitates the input of relevant data, enabling efficient forecasting and real-time updates. Furthermore, transparency in the model's workings and prediction methodologies is maintained, allowing for a clear understanding of the forecast's rationale and contributing factors. The model's output is presented in a user-friendly format, highlighting key predictions and potential risks. Importantly, the model is regularly audited and monitored to identify and address any potential issues, safeguarding the integrity of the forecasting process and ensuring ongoing reliability. Continuous improvement is a key component of our approach. Regular updates to the dataset and model architecture ensure optimal performance in the face of changing market dynamics.
ML Model Testing
n:Time series to forecast
p:Price signals of XOM stock
j:Nash equilibria (Neural Network)
k:Dominated move of XOM stock holders
a:Best response for XOM target price
For further technical information as per how our model work we invite you to visit the article below:
How do KappaSignal algorithms actually work?
XOM Stock Forecast (Buy or Sell) Strategic Interaction Table
Strategic Interaction Table Legend:
X axis: *Likelihood% (The higher the percentage value, the more likely the event will occur.)
Y axis: *Potential Impact% (The higher the percentage value, the more likely the price will deviate.)
Z axis (Grey to Black): *Technical Analysis%
ExxonMobil Financial Outlook and Forecast
ExxonMobil's financial outlook is characterized by a complex interplay of factors, primarily driven by the volatile global energy market. The company's substantial reserves of oil and natural gas provide a foundation for continued production and revenue generation, but this is increasingly juxtaposed with the accelerating shift towards renewable energy sources. Government regulations, evolving consumer preferences, and technological advancements are all shaping the long-term energy landscape and impacting ExxonMobil's profitability and growth prospects. A key element of the company's financial outlook is its capital expenditure strategy. Investments in exploration and production (E&P) activities are crucial for maintaining reserves and future output, and these expenditures are typically sensitive to market prices for oil and natural gas. ExxonMobil's ability to efficiently allocate capital across various operational segments, while simultaneously preparing for a transition to a lower carbon future, will significantly determine its long-term financial success. Crucially, the company's hedging strategies play a vital role in mitigating price fluctuations and ensuring financial stability in an uncertain market environment. Financial performance will also hinge on the company's successful execution of its diversification strategy in various renewable energy sectors, demonstrating its commitment to adapting to the changing energy mix.
Revenue streams for ExxonMobil are predominantly derived from its core fossil fuel operations. However, the company has demonstrably invested in alternative energy technologies, reflecting an acknowledgement of the long-term imperative for diversification. The transition to renewable energy sources is not expected to happen overnight, and it's likely that fossil fuels will remain a key component of the energy mix for the foreseeable future. This means that ExxonMobil's continued reliance on these sources of revenue remains important and should be considered. Long-term growth potential depends on their ability to integrate and monetize these newer technologies. The company's extensive refining and marketing infrastructure creates a significant revenue stream, and the efficient operation of these facilities remains crucial to maintaining profitability and market share. Fluctuations in global oil prices, as well as regional geopolitical events, significantly impact the company's reported income and profitability. Ultimately, ExxonMobil must successfully navigate this complex and dynamic environment to secure its position as a major energy player in the face of rapidly evolving technological and market demands.
ExxonMobil faces significant challenges stemming from the global transition to a lower-carbon energy economy. The company's position as a significant producer of fossil fuels could expose it to increased regulatory scrutiny and potentially lower demand for its products in the future. Investors will be intently watching its efforts to embrace sustainable energy alternatives, assessing its ability to make strategic investments in renewable energy technologies while still maintaining substantial profits in the current market. Moreover, ongoing volatility in global markets and potential supply chain disruptions pose a risk to the company's operations and profitability. Political and economic instability in certain regions can directly impact its production and distribution capabilities, creating operational and financial difficulties. The company's success is heavily reliant on its ability to adapt and innovate in response to these shifting dynamics. This adaptation requires both a strategic and a financial commitment to emerging technologies and markets, which are necessary for sustaining the company's future growth in a low-carbon economy.
Predictive forecast: A positive financial outlook for ExxonMobil hinges on its ability to execute its diversification strategy effectively, navigate the complexities of a global energy transition, and manage risks related to market volatility and geopolitical factors. The long-term financial performance of ExxonMobil is highly dependent on its successful implementation of measures to mitigate these risks and capitalize on potential opportunities. While the shift towards a lower-carbon energy future presents challenges, the company's extensive infrastructure and proven expertise in energy production offer substantial advantages. The prediction is cautiously optimistic, given the inherent risks involved in transitioning to a low-carbon energy system. Risks: Failure to adapt to the changing energy landscape, slower-than-projected transition to renewables, increased regulatory scrutiny related to environmental concerns, or prolonged periods of low oil prices could significantly impact financial performance, resulting in lower profitability and reduced growth.
Rating | Short-Term | Long-Term Senior |
---|---|---|
Outlook | Ba3 | Baa2 |
Income Statement | B3 | B2 |
Balance Sheet | Baa2 | Baa2 |
Leverage Ratios | Caa2 | Baa2 |
Cash Flow | Baa2 | Baa2 |
Rates of Return and Profitability | Caa2 | B1 |
*Financial analysis is the process of evaluating a company's financial performance and position by neural network. It involves reviewing the company's financial statements, including the balance sheet, income statement, and cash flow statement, as well as other financial reports and documents.
How does neural network examine financial reports and understand financial state of the company?
References
- J. Peters, S. Vijayakumar, and S. Schaal. Natural actor-critic. In Proceedings of the Sixteenth European Conference on Machine Learning, pages 280–291, 2005.
- V. Mnih, A. P. Badia, M. Mirza, A. Graves, T. P. Lillicrap, T. Harley, D. Silver, and K. Kavukcuoglu. Asynchronous methods for deep reinforcement learning. In Proceedings of the 33nd International Conference on Machine Learning, ICML 2016, New York City, NY, USA, June 19-24, 2016, pages 1928–1937, 2016
- R. Rockafellar and S. Uryasev. Optimization of conditional value-at-risk. Journal of Risk, 2:21–42, 2000.
- M. J. Hausknecht. Cooperation and Communication in Multiagent Deep Reinforcement Learning. PhD thesis, The University of Texas at Austin, 2016
- Babula, R. A. (1988), "Contemporaneous correlation and modeling Canada's imports of U.S. crops," Journal of Agricultural Economics Research, 41, 33–38.
- Breusch, T. S. (1978), "Testing for autocorrelation in dynamic linear models," Australian Economic Papers, 17, 334–355.
- Burkov A. 2019. The Hundred-Page Machine Learning Book. Quebec City, Can.: Andriy Burkov