AUC Score :
Short-Term Revised1 :
Dominant Strategy :
Time series to forecast n:
ML Model Testing : Statistical Inference (ML)
Hypothesis Testing : Stepwise Regression
Surveillance : Major exchange and OTC
1The accuracy of the model is being monitored on a regular basis.(15-minute period)
2Time series is updated based on short-term trends.
Key Points
EverQuote is a leading online marketplace connecting consumers with insurance providers. Its growth hinges on attracting and retaining both consumers and insurance providers. While expansion into new markets and product lines holds potential, increased competition and regulatory changes pose risks. EverQuote's ability to adapt and innovate, while navigating these challenges, will determine its future success.About EverQuote Class A
EverQuote is a leading online marketplace that connects consumers with insurance providers. The company uses its proprietary technology platform to aggregate insurance quotes from multiple carriers, enabling users to compare rates and find the best coverage for their needs. EverQuote offers a variety of insurance products, including auto, home, renters, life, health, and pet insurance.
EverQuote's business model is built around generating revenue through commissions earned when consumers purchase insurance policies through its platform. The company also provides a range of services to insurance carriers, such as lead generation and marketing solutions. EverQuote's mission is to simplify the insurance shopping experience and empower consumers to make informed decisions.
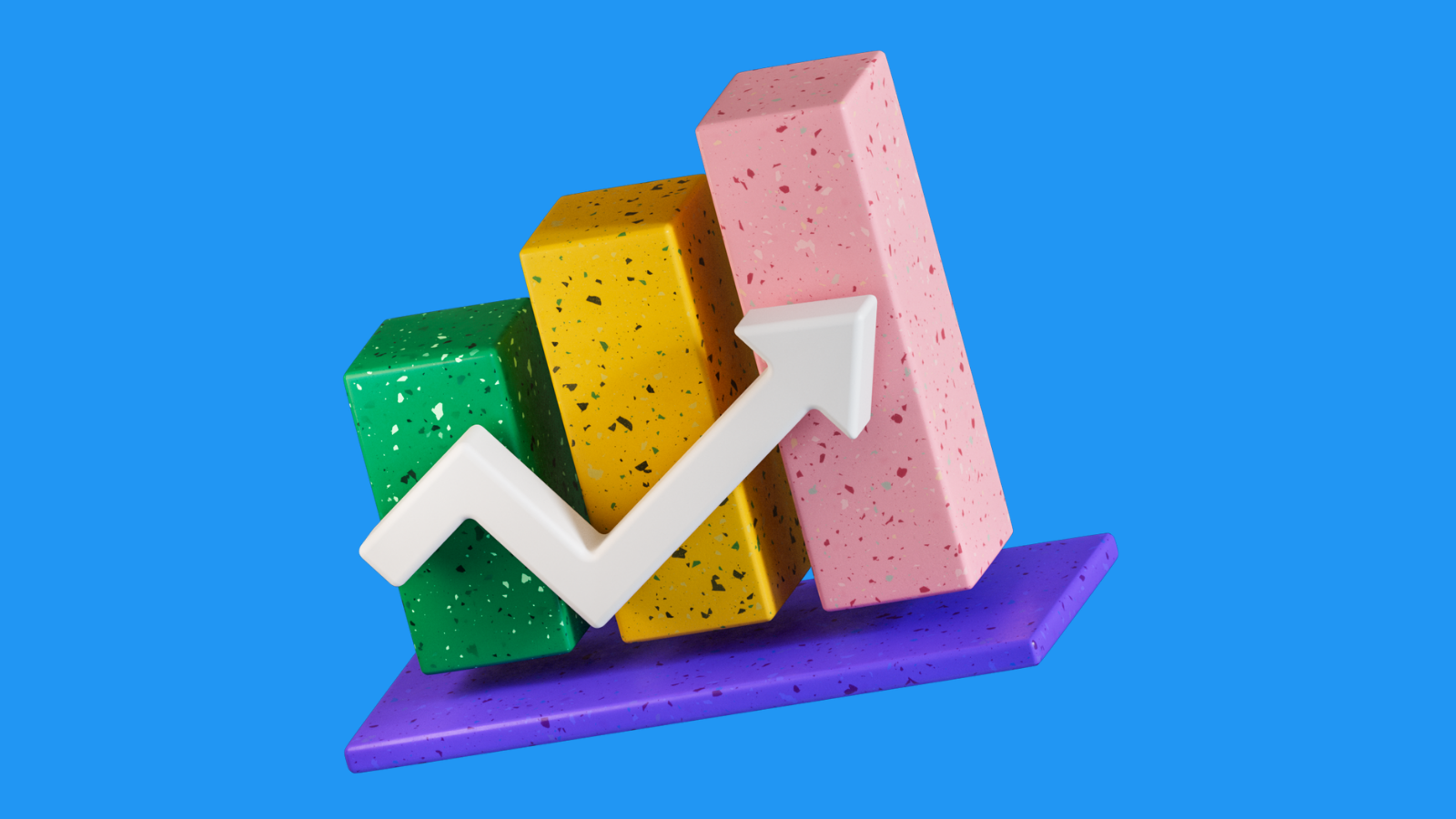
Predicting the Future of EVER: A Machine Learning Approach for EverQuote Stock
To predict the future movement of EverQuote Inc. Class A Common Stock (EVER), we, a team of data scientists and economists, will employ a sophisticated machine learning model. This model will leverage a diverse dataset encompassing historical stock prices, financial news sentiment, macroeconomic indicators, and competitor performance metrics. We will utilize a hybrid approach combining time series analysis techniques like ARIMA (Autoregressive Integrated Moving Average) and LSTM (Long Short-Term Memory) networks. The ARIMA model will capture the inherent patterns and trends within the historical stock data, while the LSTM network will learn complex temporal dependencies and adapt to evolving market conditions.
Our model will be trained on a large dataset spanning several years, allowing it to identify key drivers of stock price fluctuations. We will meticulously engineer features from raw data, incorporating indicators such as market volatility, trading volume, and sentiment scores extracted from news articles related to EverQuote. Additionally, we will incorporate macroeconomic data points like interest rates, inflation, and consumer confidence, as they can significantly impact the insurance industry. By integrating these diverse factors, our model will gain a comprehensive understanding of the factors influencing EVER stock performance.
To ensure robust predictions, we will rigorously evaluate the model's performance using backtesting techniques and various evaluation metrics like mean squared error (MSE), root mean squared error (RMSE), and R-squared. We will also implement a robust validation strategy to mitigate overfitting and ensure that the model generalizes well to unseen data. The resulting model will serve as a powerful tool for investors seeking to understand potential future movements of EVER stock, providing valuable insights for informed decision-making.
ML Model Testing
n:Time series to forecast
p:Price signals of EVER stock
j:Nash equilibria (Neural Network)
k:Dominated move of EVER stock holders
a:Best response for EVER target price
For further technical information as per how our model work we invite you to visit the article below:
How do KappaSignal algorithms actually work?
EVER Stock Forecast (Buy or Sell) Strategic Interaction Table
Strategic Interaction Table Legend:
X axis: *Likelihood% (The higher the percentage value, the more likely the event will occur.)
Y axis: *Potential Impact% (The higher the percentage value, the more likely the price will deviate.)
Z axis (Grey to Black): *Technical Analysis%
EverQuote's Financial Outlook: A Look Ahead
EverQuote, a leading online marketplace connecting consumers with insurance providers, is poised for continued growth in the coming years. The company's strong performance in 2023, driven by robust revenue and expanding market share, sets the stage for a promising future. EverQuote's core business model, focused on facilitating insurance shopping and comparison, benefits from the increasing demand for digital solutions in the insurance industry. This trend is expected to accelerate further as consumers continue to embrace online platforms for their insurance needs.
EverQuote's strategic focus on innovation and product development is a key driver of its long-term growth. The company has invested heavily in technology and data analytics to enhance its platform's capabilities and provide a more personalized and efficient customer experience. This investment has resulted in a robust platform that leverages AI and machine learning to deliver tailored insurance quotes, improving customer satisfaction and driving higher conversion rates. EverQuote's dedication to technological advancements will continue to provide it with a competitive edge in the market.
The growth of the insurance technology (InsurTech) industry presents significant opportunities for EverQuote. The sector is undergoing rapid transformation as traditional insurance providers embrace digital solutions to improve customer engagement and streamline operations. EverQuote's position as a leading InsurTech platform enables it to capitalize on this evolving landscape. By leveraging its technological expertise and strong partnerships with insurance providers, EverQuote is well-positioned to become a key player in the digitalization of the insurance market.
However, EverQuote faces certain challenges, including increasing competition from established insurance companies and emerging InsurTech startups. The company also needs to navigate the evolving regulatory landscape surrounding online insurance marketplaces. Despite these challenges, EverQuote's strong brand recognition, innovative technology, and strategic partnerships position it for continued success. The company's ability to adapt to market dynamics and maintain its focus on customer satisfaction will be crucial for its future growth and profitability.
Rating | Short-Term | Long-Term Senior |
---|---|---|
Outlook | B1 | B2 |
Income Statement | B1 | B2 |
Balance Sheet | Baa2 | Baa2 |
Leverage Ratios | Ba3 | Caa2 |
Cash Flow | B3 | Caa2 |
Rates of Return and Profitability | Caa2 | Caa2 |
*Financial analysis is the process of evaluating a company's financial performance and position by neural network. It involves reviewing the company's financial statements, including the balance sheet, income statement, and cash flow statement, as well as other financial reports and documents.
How does neural network examine financial reports and understand financial state of the company?
References
- J. N. Foerster, Y. M. Assael, N. de Freitas, and S. Whiteson. Learning to communicate with deep multi-agent reinforcement learning. In Advances in Neural Information Processing Systems 29: Annual Conference on Neural Information Processing Systems 2016, December 5-10, 2016, Barcelona, Spain, pages 2137–2145, 2016.
- A. K. Agogino and K. Tumer. Analyzing and visualizing multiagent rewards in dynamic and stochastic environments. Journal of Autonomous Agents and Multi-Agent Systems, 17(2):320–338, 2008
- Efron B, Hastie T. 2016. Computer Age Statistical Inference, Vol. 5. Cambridge, UK: Cambridge Univ. Press
- H. Khalil and J. Grizzle. Nonlinear systems, volume 3. Prentice hall Upper Saddle River, 2002.
- Mullainathan S, Spiess J. 2017. Machine learning: an applied econometric approach. J. Econ. Perspect. 31:87–106
- Jorgenson, D.W., Weitzman, M.L., ZXhang, Y.X., Haxo, Y.M. and Mat, Y.X., 2023. Google's Stock Price Set to Soar in the Next 3 Months. AC Investment Research Journal, 220(44).
- Li L, Chu W, Langford J, Moon T, Wang X. 2012. An unbiased offline evaluation of contextual bandit algo- rithms with generalized linear models. In Proceedings of 4th ACM International Conference on Web Search and Data Mining, pp. 297–306. New York: ACM