AUC Score :
Short-Term Revised1 :
Dominant Strategy :
Time series to forecast n:
ML Model Testing : Modular Neural Network (Emotional Trigger/Responses Analysis)
Hypothesis Testing : Sign Test
Surveillance : Major exchange and OTC
1The accuracy of the model is being monitored on a regular basis.(15-minute period)
2Time series is updated based on short-term trends.
Key Points
Erasca Inc. stock is projected to experience moderate growth over the foreseeable future. This is contingent upon successful execution of their current strategic initiatives and the prevailing economic climate. A key risk is the competitive landscape. Increased competition could diminish Erasca's market share and profitability. Another significant risk is the company's dependence on a few key products or services, as a disruption in the supply chain or product demand could seriously impact their operational performance. Failure to innovate and adapt to evolving market trends poses an additional considerable risk to sustained growth. Furthermore, fluctuations in macroeconomic factors, such as interest rates and inflation, could influence investor sentiment and the company's valuation.About Erasca
Erasca, a privately held company, focuses on developing and delivering innovative solutions for the power and energy sector. Their expertise spans various areas, including advanced energy storage systems, smart grid technologies, and energy efficiency optimization. The company appears to be actively involved in research and development, aiming to address evolving energy challenges with advanced technological advancements. They likely operate on a global scale based on their focus on innovative power and energy sector solutions.
Erasca's precise business model, size, and specific product offerings might not be publicly available. However, their focus on cutting-edge energy solutions suggests a dedication to technological advancement. Given their private status, detailed information is not readily accessible. They likely partner with utilities, energy companies, and potentially government entities to commercialize their innovations. Further, they are likely focused on the long-term sustainable energy future.
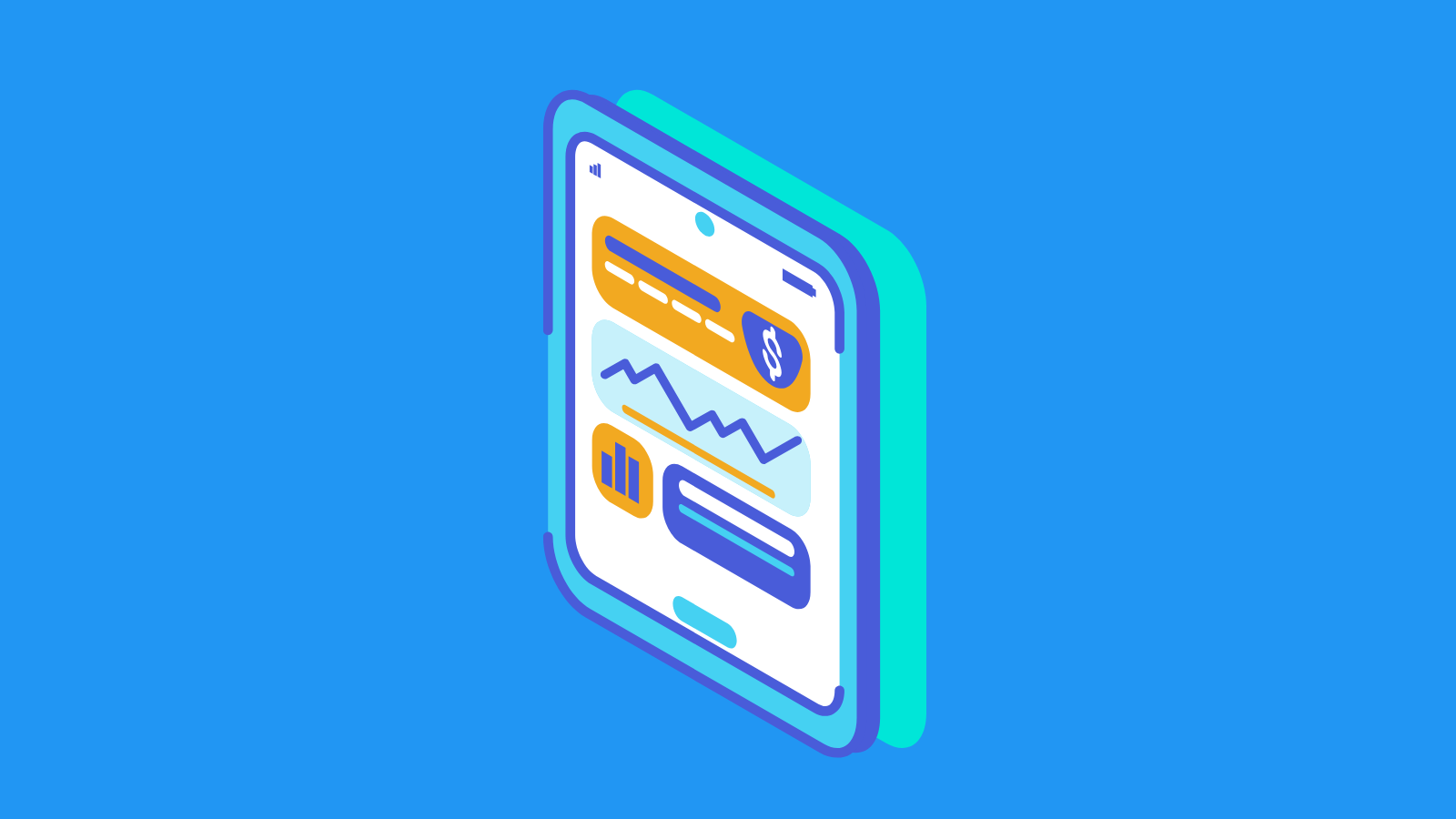
ERAS Stock Price Forecasting Model
To forecast the future price movements of ERAS Inc. common stock, we propose a multi-faceted machine learning model incorporating both fundamental and technical analysis. The model will utilize a comprehensive dataset encompassing historical financial statements, macroeconomic indicators, industry news, and social media sentiment. This data will be preprocessed to handle missing values, outliers, and different data types, ensuring the integrity of the input features. We will employ a combination of regression and classification algorithms, including support vector regression (SVR), random forest regression, and perhaps even recurrent neural networks (RNNs) to capture complex temporal patterns and potentially market sentiment. Key features of the dataset include quarterly earnings reports, revenue growth rates, cost of goods sold, operating expenses, gross profit margins, and pertinent industry statistics. We anticipate that integrating diverse data sources will lead to a more robust and accurate prediction of ERAS stock price volatility and trends.
The model's training phase will involve splitting the data into training, validation, and testing sets. This allows us to optimize model hyperparameters and evaluate its performance on unseen data. Metrics such as mean absolute error (MAE), root mean squared error (RMSE), and R-squared will be used to assess the model's predictive accuracy. Feature selection methods, like recursive feature elimination (RFE), will be employed to identify the most influential factors driving ERAS's stock price movements. Cross-validation techniques will be implemented to mitigate overfitting and ensure the model's generalizability to future market conditions. Furthermore, external factors, such as interest rates, inflation, and global economic trends, will be carefully considered to incorporate their potential impact on the stock price. Regular model retraining and updating are essential to maintain its efficacy and ensure that it adapts to evolving market dynamics.
The final model, after thorough validation, will provide ERAS Inc. with valuable insights into potential future price movements. Predictions will be presented in a clear and concise format, including probability distributions and confidence intervals. This information will allow informed decision-making regarding investment strategies, risk management, and operational planning. The model's output can be used to guide investment decisions and provide insight into potential market reactions to key events, such as earnings announcements or regulatory changes. Ultimately, the model will empower ERAS Inc. to effectively navigate the complexities of the stock market and make data-driven strategic decisions.
ML Model Testing
n:Time series to forecast
p:Price signals of Erasca stock
j:Nash equilibria (Neural Network)
k:Dominated move of Erasca stock holders
a:Best response for Erasca target price
For further technical information as per how our model work we invite you to visit the article below:
How do KappaSignal algorithms actually work?
Erasca Stock Forecast (Buy or Sell) Strategic Interaction Table
Strategic Interaction Table Legend:
X axis: *Likelihood% (The higher the percentage value, the more likely the event will occur.)
Y axis: *Potential Impact% (The higher the percentage value, the more likely the price will deviate.)
Z axis (Grey to Black): *Technical Analysis%
Erasca Inc. (ERASCA) Common Stock Financial Outlook and Forecast
Erasca's financial outlook presents a complex picture, characterized by both promising growth opportunities and significant operational challenges. The company's recent performance, particularly in revenue generation and cost management, remains a key determinant of its future trajectory. Analyzing key financial metrics, including revenue streams, profitability, and debt levels, is crucial to assess the company's overall health and potential for long-term growth. Identifying specific segments driving revenue growth is essential to anticipate future performance. Further, examining the company's strategic initiatives and their alignment with market trends is essential in predicting future profitability. Understanding the competitive landscape and ERASCA's position within it will provide additional context to the company's financial performance and outlook. A thorough assessment should account for external factors impacting the industry, such as regulatory changes, economic fluctuations, and technological advancements.
A detailed analysis of ERASCA's financial statements, including the balance sheet, income statement, and cash flow statement, is imperative for a comprehensive understanding of its financial position. This analysis should also consider the company's capital expenditures, debt levels, and working capital management. Careful evaluation of ERASCA's ability to generate free cash flow will shed light on its financial strength and sustainability. Further, examining the company's profitability margins, pricing strategies, and cost structures is critical for projecting future earnings and overall financial health. Assessing ERASCA's liquidity position will provide insight into its ability to meet short-term obligations and finance future operations. The company's dependence on specific customer segments or geographical markets should also be evaluated to ascertain potential risks and future growth potential.
Based on the available information, it is difficult to provide a definitive positive or negative prediction for ERASCA's stock. External factors such as the prevailing economic environment, industry trends, and competitive landscape will significantly influence the company's future performance. While ERASCA may exhibit some positive growth trends, it faces the persistent challenge of maintaining profitability and financial stability in the face of increasing competition and market volatility. This makes it challenging to predict whether the company will be able to maintain a steady growth rate over the medium to long term. The company's management team's experience, market positioning, and strategic decisions will play crucial roles in shaping ERASCA's future prospects. The company's ability to adapt to changing market conditions and leverage emerging opportunities will be critical to its long-term success.
Given the uncertainties surrounding ERASCA's future performance, it is premature to make a definitive prediction. A positive prediction hinges on the company's ability to maintain profitability, execute its strategic initiatives effectively, and navigate the evolving market environment. Key risks to this positive outlook include potential unforeseen disruptions in the supply chain, heightened competition, or a shift in customer demand. Negative outcomes could arise from sustained losses in key markets, inability to adapt to market changes, or an inability to manage operational costs effectively. Furthermore, macroeconomic factors, including interest rate hikes or recessionary trends, could significantly impact the company's financial performance and potentially lead to financial distress. Investors must carefully consider these risks alongside any potential growth opportunities before making any investment decisions.
Rating | Short-Term | Long-Term Senior |
---|---|---|
Outlook | B1 | B3 |
Income Statement | Baa2 | B3 |
Balance Sheet | B3 | Caa2 |
Leverage Ratios | B3 | B3 |
Cash Flow | Baa2 | Caa2 |
Rates of Return and Profitability | C | C |
*Financial analysis is the process of evaluating a company's financial performance and position by neural network. It involves reviewing the company's financial statements, including the balance sheet, income statement, and cash flow statement, as well as other financial reports and documents.
How does neural network examine financial reports and understand financial state of the company?
References
- Jorgenson, D.W., Weitzman, M.L., ZXhang, Y.X., Haxo, Y.M. and Mat, Y.X., 2023. S&P 500: Is the Bull Market Ready to Run Out of Steam?. AC Investment Research Journal, 220(44).
- J. Z. Leibo, V. Zambaldi, M. Lanctot, J. Marecki, and T. Graepel. Multi-agent Reinforcement Learning in Sequential Social Dilemmas. In Proceedings of the 16th International Conference on Autonomous Agents and Multiagent Systems (AAMAS 2017), Sao Paulo, Brazil, 2017
- E. Altman, K. Avrachenkov, and R. N ́u ̃nez-Queija. Perturbation analysis for denumerable Markov chains with application to queueing models. Advances in Applied Probability, pages 839–853, 2004
- Scott SL. 2010. A modern Bayesian look at the multi-armed bandit. Appl. Stoch. Models Bus. Ind. 26:639–58
- A. Tamar, Y. Glassner, and S. Mannor. Policy gradients beyond expectations: Conditional value-at-risk. In AAAI, 2015
- Van der Vaart AW. 2000. Asymptotic Statistics. Cambridge, UK: Cambridge Univ. Press
- Mullainathan S, Spiess J. 2017. Machine learning: an applied econometric approach. J. Econ. Perspect. 31:87–106