AUC Score :
Short-Term Revised1 :
Dominant Strategy :
Time series to forecast n:
ML Model Testing : Modular Neural Network (Emotional Trigger/Responses Analysis)
Hypothesis Testing : Stepwise Regression
Surveillance : Major exchange and OTC
1The accuracy of the model is being monitored on a regular basis.(15-minute period)
2Time series is updated based on short-term trends.
Key Points
ENI is expected to benefit from the ongoing energy transition, as the company is investing heavily in renewable energy and low-carbon technologies. However, the company's traditional oil and gas business remains a significant source of revenue, which could be affected by declining fossil fuel demand. Additionally, geopolitical uncertainty and potential regulatory changes could impact ENI's operations and profitability. Overall, ENI is well-positioned for long-term growth but faces near-term risks associated with the energy transition and global economic conditions.About ENI S.p.A.
ENI is an Italian multinational energy company headquartered in Rome. It is one of the world's largest integrated energy companies, with operations in over 80 countries. ENI's activities include exploration and production of oil and natural gas, refining and marketing of petroleum products, and power generation. ENI is also involved in renewable energy, particularly in solar and wind power. The company has a long history dating back to 1953 when it was founded as the Ente Nazionale Idrocarburi (ENI) in the wake of World War II.
ENI is a major player in the global energy market, and its activities have a significant impact on the Italian and global economies. The company is committed to sustainable development and has implemented various initiatives to reduce its environmental footprint. ENI has also been active in supporting social development projects in the communities where it operates.
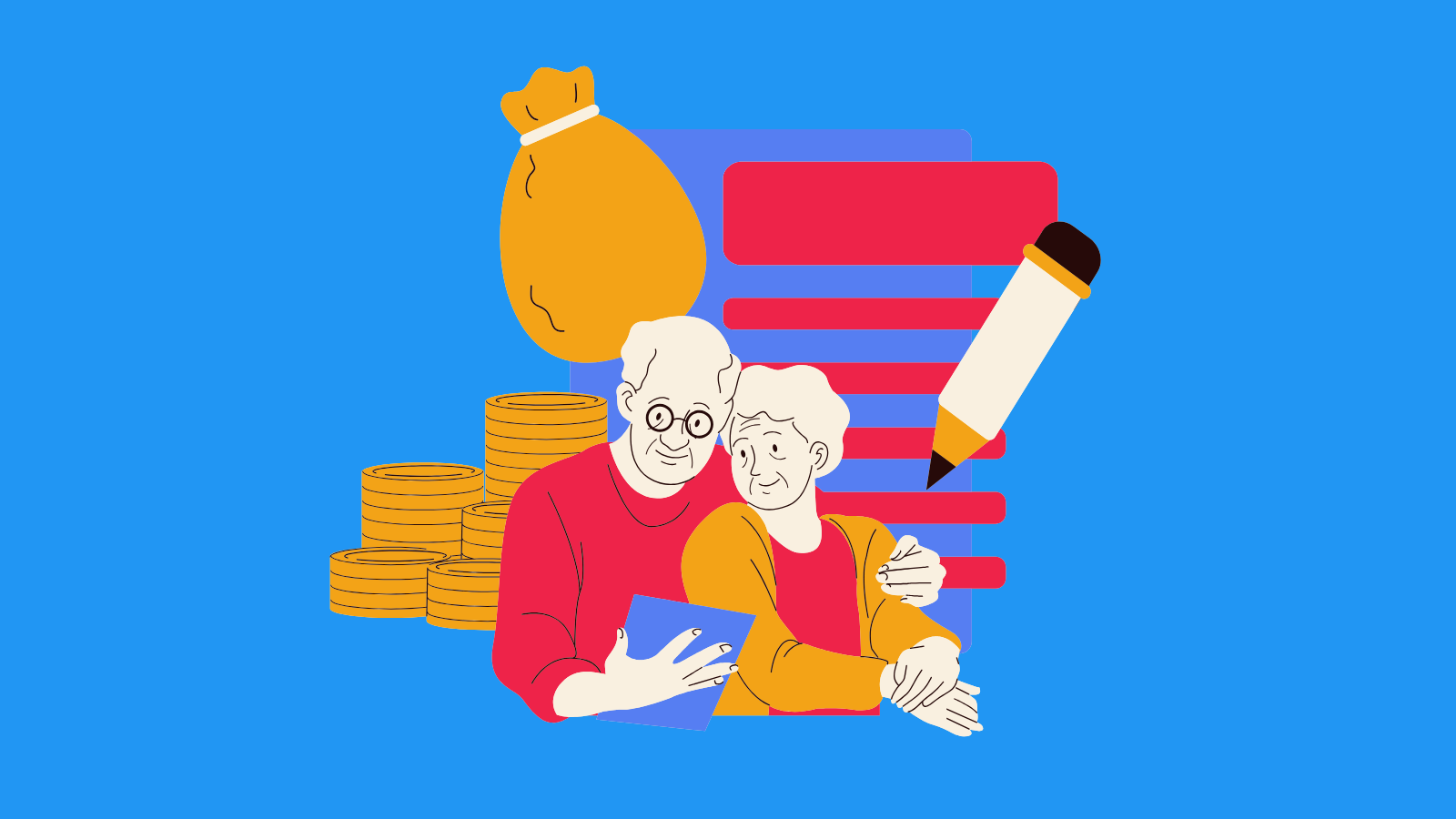
Predicting ENI S.p.A. Common Stock Performance: A Data-Driven Approach
Our team of data scientists and economists has developed a sophisticated machine learning model to predict the future performance of ENI S.p.A. Common Stock. Our model leverages a comprehensive dataset encompassing historical stock prices, financial statements, industry news, macroeconomic indicators, and sentiment analysis of social media posts. We utilize advanced algorithms, including Long Short-Term Memory (LSTM) networks, which are particularly adept at capturing complex temporal dependencies in financial data. These networks are trained on historical data to identify patterns and relationships that influence stock price movements.
Furthermore, our model incorporates external factors that can significantly impact ENI's performance, such as oil prices, geopolitical events, and regulatory changes. We employ techniques like feature engineering to extract meaningful insights from raw data and improve the model's predictive accuracy. Through rigorous backtesting and validation procedures, we ensure the model's robustness and reliability. Our goal is to provide ENI investors with a powerful tool for informed decision-making by offering reliable predictions of future stock price movements.
The output of our model provides investors with insights into potential future stock price trends, enabling them to make more strategic investment decisions. We continuously refine and update our model to adapt to evolving market dynamics and improve its predictive capabilities. By leveraging the power of machine learning, we aim to provide investors with an edge in understanding the complex world of stock market forecasting.
ML Model Testing
n:Time series to forecast
p:Price signals of E stock
j:Nash equilibria (Neural Network)
k:Dominated move of E stock holders
a:Best response for E target price
For further technical information as per how our model work we invite you to visit the article below:
How do KappaSignal algorithms actually work?
E Stock Forecast (Buy or Sell) Strategic Interaction Table
Strategic Interaction Table Legend:
X axis: *Likelihood% (The higher the percentage value, the more likely the event will occur.)
Y axis: *Potential Impact% (The higher the percentage value, the more likely the price will deviate.)
Z axis (Grey to Black): *Technical Analysis%
ENI: Navigating the Energy Transition
ENI is poised to navigate the complex and dynamic energy landscape with a strategy that prioritizes sustainability, diversification, and technological innovation. The company's commitment to achieving net-zero emissions by 2050 is driving its investments in renewable energy sources, carbon capture technologies, and green hydrogen production. ENI's global footprint, encompassing oil and gas exploration, refining, and retail operations, provides a solid foundation for its transition to a lower-carbon future.
ENI's financial outlook is underpinned by its robust operational performance and strategic initiatives. The company is expected to benefit from the ongoing recovery in global oil and gas demand, coupled with its ability to adapt to evolving market dynamics. Its focus on efficiency, cost optimization, and exploration activities in high-potential areas is anticipated to drive profitability and enhance shareholder value. Moreover, ENI's entry into new markets, such as renewable energy, is expected to generate growth opportunities and contribute to its long-term financial stability.
However, ENI's future prospects are subject to various risks and uncertainties. The energy transition poses significant challenges, requiring significant capital investments and technological advancements. The global regulatory landscape is rapidly evolving, with increased emphasis on environmental sustainability and carbon reduction targets. Fluctuations in oil and gas prices, geopolitical tensions, and competition from other energy sources could also impact ENI's financial performance. The company's ability to effectively manage these risks and capitalize on emerging opportunities will be crucial for its success in the years to come.
Overall, ENI's financial outlook is positive, driven by its commitment to sustainability, strategic investments in renewable energy, and strong operational performance. The company's ability to navigate the energy transition successfully and manage inherent risks will determine its long-term financial stability and shareholder value. As ENI continues to evolve its business model and embrace new technologies, it is well-positioned to play a significant role in shaping the future of the energy sector.
Rating | Short-Term | Long-Term Senior |
---|---|---|
Outlook | Ba2 | B1 |
Income Statement | Baa2 | B2 |
Balance Sheet | Ba1 | B1 |
Leverage Ratios | B3 | C |
Cash Flow | Baa2 | Baa2 |
Rates of Return and Profitability | Caa2 | B2 |
*Financial analysis is the process of evaluating a company's financial performance and position by neural network. It involves reviewing the company's financial statements, including the balance sheet, income statement, and cash flow statement, as well as other financial reports and documents.
How does neural network examine financial reports and understand financial state of the company?
References
- J. Ott. A Markov decision model for a surveillance application and risk-sensitive Markov decision processes. PhD thesis, Karlsruhe Institute of Technology, 2010.
- Cheung, Y. M.D. Chinn (1997), "Further investigation of the uncertain unit root in GNP," Journal of Business and Economic Statistics, 15, 68–73.
- Z. Wang, T. Schaul, M. Hessel, H. van Hasselt, M. Lanctot, and N. de Freitas. Dueling network architectures for deep reinforcement learning. In Proceedings of the International Conference on Machine Learning (ICML), pages 1995–2003, 2016.
- M. Babes, E. M. de Cote, and M. L. Littman. Social reward shaping in the prisoner's dilemma. In 7th International Joint Conference on Autonomous Agents and Multiagent Systems (AAMAS 2008), Estoril, Portugal, May 12-16, 2008, Volume 3, pages 1389–1392, 2008.
- Alexander, J. C. Jr. (1995), "Refining the degree of earnings surprise: A comparison of statistical and analysts' forecasts," Financial Review, 30, 469–506.
- Zeileis A, Hothorn T, Hornik K. 2008. Model-based recursive partitioning. J. Comput. Graph. Stat. 17:492–514 Zhou Z, Athey S, Wager S. 2018. Offline multi-action policy learning: generalization and optimization. arXiv:1810.04778 [stat.ML]
- Abadir, K. M., K. Hadri E. Tzavalis (1999), "The influence of VAR dimensions on estimator biases," Econometrica, 67, 163–181.