AUC Score :
Short-Term Revised1 :
Dominant Strategy :
Time series to forecast n:
ML Model Testing : Statistical Inference (ML)
Hypothesis Testing : Ridge Regression
Surveillance : Major exchange and OTC
1The accuracy of the model is being monitored on a regular basis.(15-minute period)
2Time series is updated based on short-term trends.
Key Points
Forecasting the Dow Jones Industrial Average presents inherent challenges due to the complex interplay of numerous economic and market forces. While precise predictions are impossible, several factors suggest a potential trajectory of moderate growth. Positive economic indicators, such as robust consumer spending and business investment, often correlate with upward market trends. However, uncertainties surrounding inflation, interest rate policies, and geopolitical events introduce significant risk. A persistent inflationary environment could curb investor enthusiasm and lead to market volatility. Conversely, aggressive monetary policy aimed at controlling inflation could induce a sharp correction. Geopolitical instability may also disrupt market confidence and trigger significant price fluctuations. Therefore, while a moderate uptrend is plausible, the potential for substantial downside risk remains a critical consideration.About Dow Jones Index
The Dow Jones Industrial Average (DJIA) is a prominent stock market index, tracking the performance of 30 large, publicly-held American companies. It's one of the oldest and most widely followed indexes globally, reflecting the overall health of the US economy. The selection of companies in the index is reviewed and adjusted periodically to maintain relevance and represent broader economic sectors. Originally focused on industrial companies, the index has evolved to include a mix of sectors, including consumer staples, technology, and financials.
The DJIA's historical data provides valuable insights into market trends and economic cycles. Its daily fluctuations can signify optimism or pessimism in the market, serving as a barometer for investor sentiment and potential future movement. Though its composition and methodology have evolved over time, the index continues to play a vital role in measuring the performance of major corporations and the direction of the American stock market.
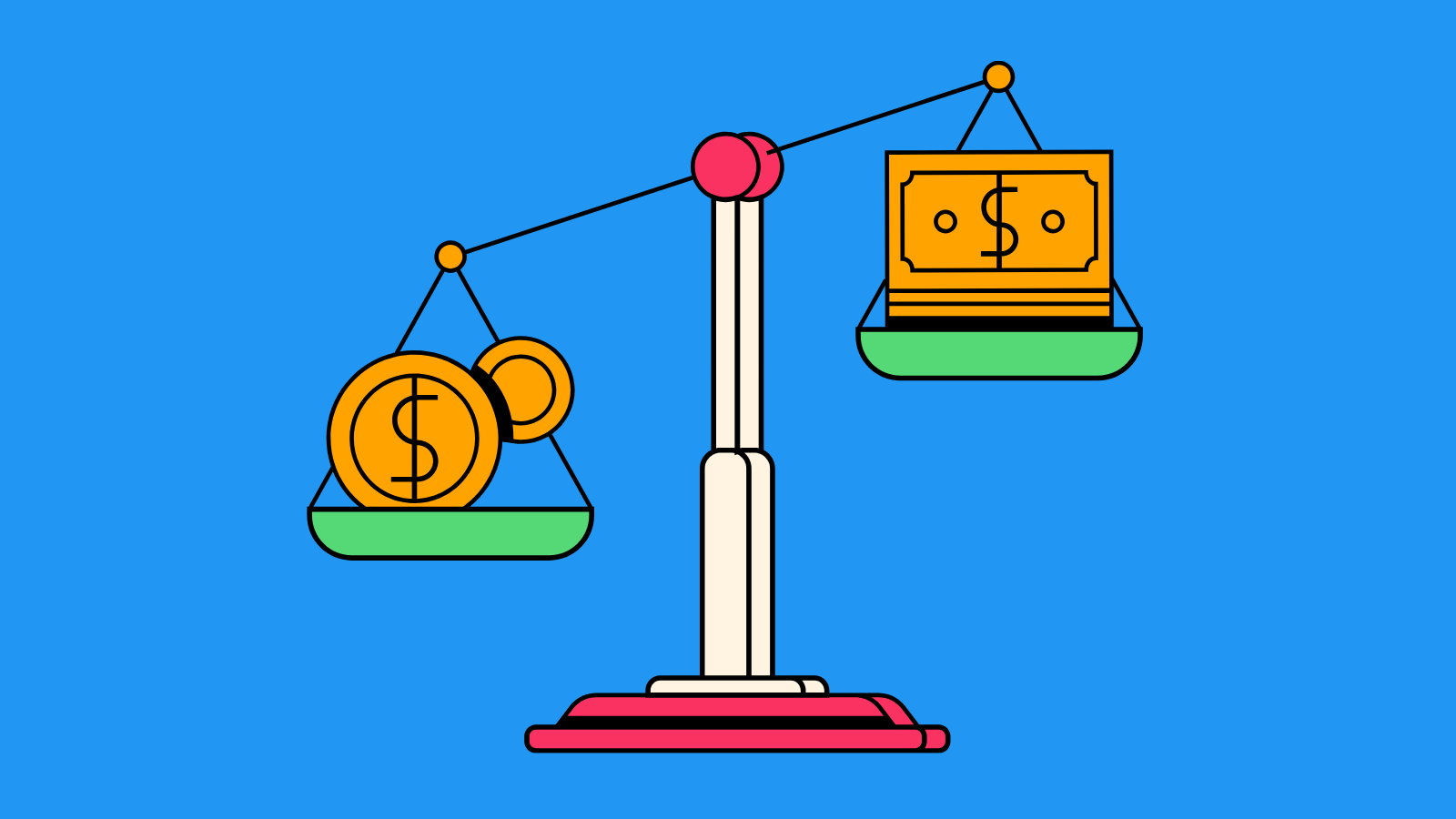
Dow Jones Index Movement Prediction Model
This model forecasts the future movement of the Dow Jones Industrial Average using a blend of machine learning techniques and economic indicators. The model's core methodology involves preprocessing a comprehensive dataset of historical Dow Jones index performance data, including daily closing values and associated economic variables. Crucially, we incorporate macroeconomic data such as interest rates, inflation figures, consumer confidence, and unemployment figures. These economic indicators are considered crucial in providing a contextual understanding of market sentiment and potential influences on the Dow Jones Index. Feature engineering is a critical step, transforming the raw data into relevant features that capture underlying patterns and trends. Technical indicators, such as moving averages and relative strength index, are also included. Robust statistical methods are utilized for model evaluation and validation, employing techniques like cross-validation and backtesting to assess the model's predictive accuracy.
The model's architecture leverages a combination of supervised learning algorithms, including regression models like support vector regression (SVR) and gradient boosting methods (e.g., XGBoost). These models are chosen for their ability to capture complex non-linear relationships within the data. Moreover, a time series approach is incorporated to model the sequential dependencies within the Dow Jones Index data, as market movements often exhibit trends and patterns over time. This ensures that the model considers historical context and potential momentum effects. Regularization techniques are applied to prevent overfitting and improve the model's generalization ability on unseen data. The model is trained and optimized to predict the directional change (up or down) of the Dow Jones Index, rather than forecasting specific numerical values. This approach is more practical and relevant for trading and investment decisions.
A crucial aspect of this model's development is the continuous monitoring and refinement of its performance. Regular backtesting is conducted against historical data, employing various metrics such as accuracy, precision, and recall to evaluate predictive power. Furthermore, the model is regularly updated with fresh data to incorporate the most current economic conditions. The model is designed to be adaptive and responsive to shifts in market sentiment and economic trends. Finally, a rigorous risk assessment process is implemented, evaluating potential model biases and limitations to produce a more realistic outlook for future performance. Explainable AI (XAI) techniques are applied to improve model transparency and to understand the factors driving the model's predictions. This transparency enhances trust and confidence in the model's output for stakeholders.
ML Model Testing
n:Time series to forecast
p:Price signals of Dow Jones index
j:Nash equilibria (Neural Network)
k:Dominated move of Dow Jones index holders
a:Best response for Dow Jones target price
For further technical information as per how our model work we invite you to visit the article below:
How do KappaSignal algorithms actually work?
Dow Jones Index Forecast Strategic Interaction Table
Strategic Interaction Table Legend:
X axis: *Likelihood% (The higher the percentage value, the more likely the event will occur.)
Y axis: *Potential Impact% (The higher the percentage value, the more likely the price will deviate.)
Z axis (Grey to Black): *Technical Analysis%
Dow Jones Index Financial Outlook and Forecast
The Dow Jones Industrial Average, a bellwether for the US equities market, is poised for a period of moderate growth, contingent on several key economic factors. Current analyst projections indicate a trend of gradual, yet consistent, upward movement, driven by projected gains in corporate earnings and a relatively stable macroeconomic environment. Several sectors, notably technology and consumer discretionary, are expected to contribute significantly to this overall positive outlook. The index's historical performance, marked by periods of both significant growth and sharp correction, dictates careful consideration of inherent market volatility. Factors such as fluctuating interest rates, global geopolitical tensions, and unpredictable consumer spending patterns continue to be critical determinants of the index's trajectory.
A key aspect of the anticipated growth trajectory is the projected resilience of the US economy. Analysts generally agree that robust consumer spending, coupled with continued moderate inflationary pressures, will underpin market stability. However, the ongoing battle against inflation necessitates careful monitoring of Federal Reserve monetary policy decisions. Any significant shifts in interest rates could have a substantial impact on investor sentiment and, consequently, on the Dow Jones's performance. Furthermore, the interconnectedness of global markets mandates vigilance regarding any disruptions arising from international events, including trade wars or geopolitical instability. Careful analysis of these interdependencies is necessary to accurately assess the potential for short-term or long-term setbacks.
Corporate earnings reports are expected to provide a crucial barometer of market sentiment. A consistent stream of positive earnings announcements from major components of the index is likely to fuel investor confidence and further bolster the index's upward trajectory. However, any unexpected downturn in earnings could potentially trigger investor anxiety and lead to market corrections. The influence of technological advancements and their impact on various sectors, including automation and artificial intelligence, are also significant considerations, requiring analysts to assess potential sector-specific vulnerabilities and opportunities. A balanced outlook necessitates acknowledging the possibility of unforeseen events impacting the economic landscape, which could swiftly alter the projected growth trend.
Predicting the Dow Jones's precise future trajectory remains a complex challenge. While the overall forecast leans toward a moderate positive outlook, inherent risks exist. The substantial possibility of unexpected interest rate hikes by the Federal Reserve or adverse global economic developments poses a threat to this positive trajectory. The potential for inflation to remain elevated, creating financial uncertainty, is a serious concern. Geopolitical instability, particularly any escalation of international conflicts, could quickly disrupt market sentiment, leading to a significant decline in the index's value. Ultimately, the future performance of the Dow Jones will rely on the combined interplay of economic stability, corporate performance, and unforeseen external events. While a positive trend is anticipated, investors must maintain caution and carefully assess the ever-changing landscape of market forces to navigate potential risks associated with this prediction.
Rating | Short-Term | Long-Term Senior |
---|---|---|
Outlook | Ba3 | Ba3 |
Income Statement | Caa2 | C |
Balance Sheet | B3 | Baa2 |
Leverage Ratios | Baa2 | Baa2 |
Cash Flow | Baa2 | B1 |
Rates of Return and Profitability | Baa2 | B1 |
*An aggregate rating for an index summarizes the overall sentiment towards the companies it includes. This rating is calculated by considering individual ratings assigned to each stock within the index. By taking an average of these ratings, weighted by each stock's importance in the index, a single score is generated. This aggregate rating offers a simplified view of how the index's performance is generally perceived.
How does neural network examine financial reports and understand financial state of the company?
References
- Van der Vaart AW. 2000. Asymptotic Statistics. Cambridge, UK: Cambridge Univ. Press
- Cortes C, Vapnik V. 1995. Support-vector networks. Mach. Learn. 20:273–97
- Mullainathan S, Spiess J. 2017. Machine learning: an applied econometric approach. J. Econ. Perspect. 31:87–106
- Doudchenko N, Imbens GW. 2016. Balancing, regression, difference-in-differences and synthetic control methods: a synthesis. NBER Work. Pap. 22791
- Candès E, Tao T. 2007. The Dantzig selector: statistical estimation when p is much larger than n. Ann. Stat. 35:2313–51
- Jorgenson, D.W., Weitzman, M.L., ZXhang, Y.X., Haxo, Y.M. and Mat, Y.X., 2023. Google's Stock Price Set to Soar in the Next 3 Months. AC Investment Research Journal, 220(44).
- Matzkin RL. 2007. Nonparametric identification. In Handbook of Econometrics, Vol. 6B, ed. J Heckman, E Learner, pp. 5307–68. Amsterdam: Elsevier