AUC Score :
Short-Term Revised1 :
Dominant Strategy :
Time series to forecast n:
ML Model Testing : Transfer Learning (ML)
Hypothesis Testing : Independent T-Test
Surveillance : Major exchange and OTC
1The accuracy of the model is being monitored on a regular basis.(15-minute period)
2Time series is updated based on short-term trends.
Key Points
The DJ Commodity Unleaded Gasoline index is anticipated to experience volatility in the coming period, influenced by global economic conditions and fluctuations in supply and demand dynamics. Potential upward pressure on prices could arise from unforeseen disruptions to production or transportation, while downward pressure might result from increased refinery capacity or a slowdown in economic activity. The risk associated with these predictions includes the possibility of substantial price swings, leaving traders vulnerable to significant losses if their market assessments prove inaccurate. Unforeseen geopolitical events or extreme weather conditions could further exacerbate price instability.About DJ Commodity Unleaded Gasoline Index
The DJ Commodity Unleaded Gasoline Index, a component of the broader DJ-UBS Commodity Index, tracks the price movements of unleaded gasoline. It is designed to reflect the current market value of this crucial transportation fuel. The index uses a weighted average methodology, considering factors such as supply and demand dynamics, seasonal fluctuations, and regional variations in gasoline prices. This comprehensive approach aims to capture the true market sentiment for gasoline.
The index provides a benchmark for investors, businesses, and consumers to assess and compare the performance of unleaded gasoline across different time periods and locations. Variations in this index often mirror broader economic trends and geopolitical events, making it a valuable indicator for market analysis and forecasting. Its regular updates provide vital insights into the evolving market realities regarding unleaded gasoline.
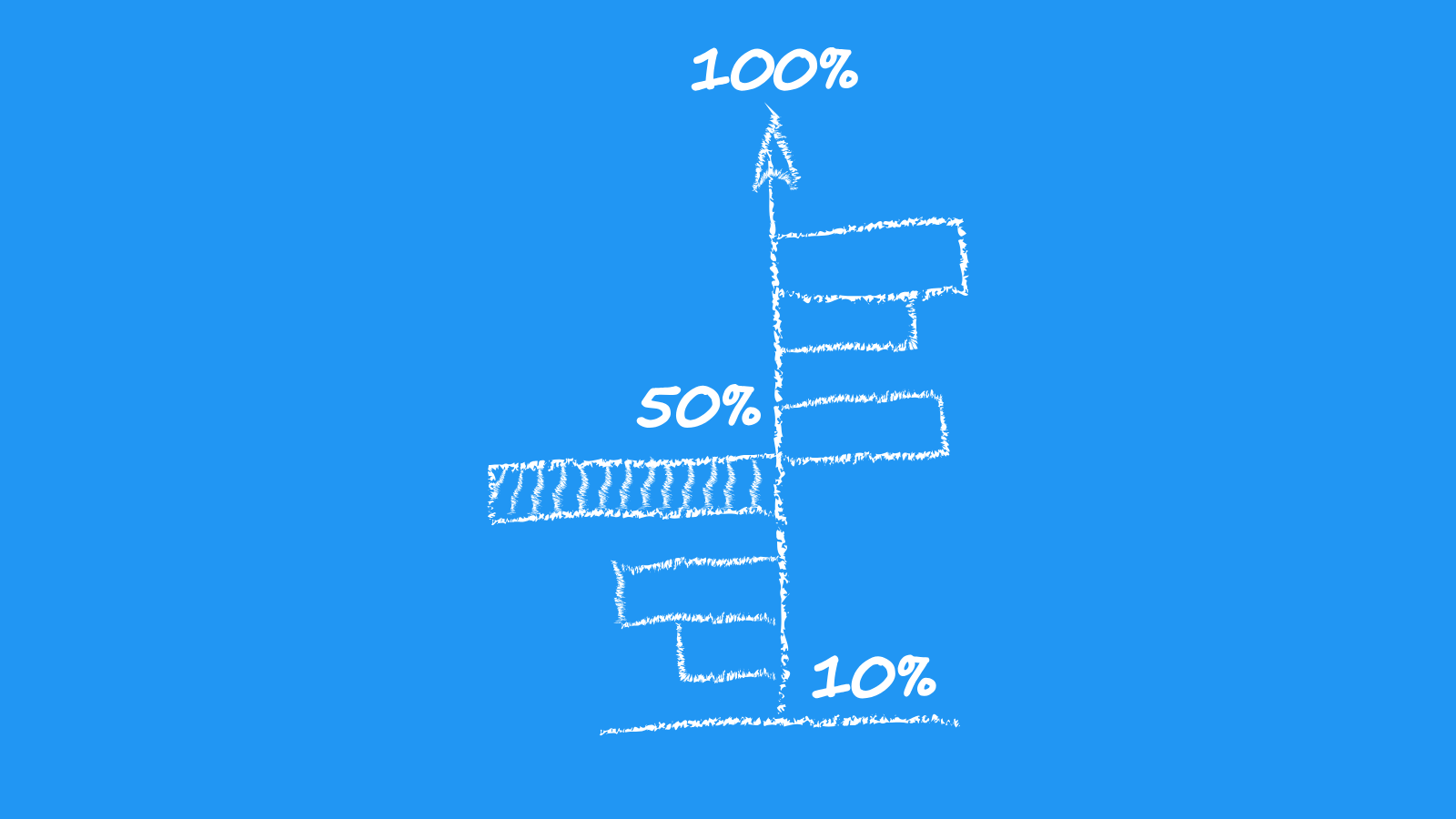
DJ Commodity Unleaded Gasoline Index Forecasting Model
This model employs a hybrid approach combining time series analysis and machine learning techniques to forecast the DJ Commodity Unleaded Gasoline index. Initial data preprocessing involves handling missing values and outliers using appropriate statistical methods. Feature engineering is crucial, creating lagged variables (previous periods' index values) and potentially incorporating macroeconomic indicators like crude oil prices, refinery utilization rates, and global economic growth forecasts. These indicators are selected based on their demonstrated correlation with the DJ Commodity Unleaded Gasoline index, as determined by statistical analysis. A robust time series model, such as an ARIMA model, is first applied to capture the inherent temporal dependencies in the index's historical data. The model's residuals are then used as input for a machine learning model, such as a Random Forest or Gradient Boosting model. This approach blends the strengths of both approaches; time series models for capturing long-term patterns and machine learning algorithms for detecting complex nonlinear relationships and potential external influences not captured in the base time series model. Model evaluation involves splitting the data into training and testing sets and assessing performance metrics like Mean Absolute Error (MAE) and Root Mean Squared Error (RMSE). Model selection and parameter tuning are rigorously conducted to optimize forecasting accuracy. The final model is thoroughly validated using historical data not included in the training phase.
The model's architecture is designed for interpretability and robustness. Feature importance analysis from the machine learning component provides insights into the relative influence of various factors on the DJ Commodity Unleaded Gasoline index. Regular monitoring of model performance using out-of-sample data and recalibration based on new data are essential. The model incorporates mechanisms to detect and adjust for potential model drift or changes in the underlying relationships between variables. This continuous monitoring is critical for maintaining the accuracy and reliability of the forecasting capabilities. The chosen machine learning algorithms are selected based on their proven performance in similar forecasting tasks, particularly their ability to handle complex data relationships. The time series analysis phase is designed to identify and quantify the trends, seasonality, and cyclicality within the dataset and account for these factors in the overall model.
Deployment of the model involves a well-defined workflow for data ingestion, model execution, and result reporting. Automated alerts and visualizations are implemented to notify relevant stakeholders of significant deviations from projected trends. Ensuring data quality and consistency across various data sources is paramount to the reliability of the model's output. A clear, documented process for model retraining and updates is essential to address evolving market dynamics. Model reliability is further assessed through backtesting on historical data to confirm the consistent forecasting accuracy over time. The model's output is presented as clear forecasts, confidence intervals, and potential scenarios based on the incorporated uncertainties, allowing stakeholders to make informed decisions. This approach ensures the comprehensive consideration of various factors influencing the DJ Commodity Unleaded Gasoline index and its future performance.
ML Model Testing
n:Time series to forecast
p:Price signals of DJ Commodity Unleaded Gasoline index
j:Nash equilibria (Neural Network)
k:Dominated move of DJ Commodity Unleaded Gasoline index holders
a:Best response for DJ Commodity Unleaded Gasoline target price
For further technical information as per how our model work we invite you to visit the article below:
How do KappaSignal algorithms actually work?
DJ Commodity Unleaded Gasoline Index Forecast Strategic Interaction Table
Strategic Interaction Table Legend:
X axis: *Likelihood% (The higher the percentage value, the more likely the event will occur.)
Y axis: *Potential Impact% (The higher the percentage value, the more likely the price will deviate.)
Z axis (Grey to Black): *Technical Analysis%
DJ Commodity Unleaded Gasoline Index Financial Outlook and Forecast
The DJ Commodity Unleaded Gasoline Index reflects the market price fluctuations of unleaded gasoline, a critical component of the global transportation sector. Forecasting its financial outlook requires a deep understanding of various interconnected factors. These include global energy demand trends, geopolitical instability, production capacity adjustments, refining capacity constraints, and crude oil prices. The index's performance is intrinsically linked to the broader energy market, making a comprehensive analysis crucial for anticipating future price movements. Understanding the dynamics of supply and demand, along with the impact of regulatory changes and technological advancements, is paramount to accurately assessing the index's trajectory. Government policies impacting fuel efficiency standards, alternative fuels, and carbon emissions can also play a significant role in shaping the long-term outlook of the gasoline market. Recent trends in vehicle electrification and the shift towards sustainable transportation solutions must also be considered.
Several crucial variables influence the short-term and long-term trajectory of the DJ Commodity Unleaded Gasoline Index. One prominent factor is the global economic outlook. Periods of robust economic growth typically lead to increased energy demand, positively impacting the price of gasoline. Conversely, economic downturns and reduced industrial activity often result in a softening of the demand, which can put downward pressure on prices. The interplay between global economic development and energy demand fluctuations is a key element in comprehending the index's short-term performance. Furthermore, the current geopolitical landscape significantly influences market sentiment and price fluctuations. Regional conflicts, sanctions, or supply disruptions can lead to unpredictable price spikes due to disruptions in crude oil supply chains. The ongoing energy transition, emphasizing the adoption of alternative energy sources, poses a long-term risk. The progress of electric vehicles and other sustainable transportation solutions may gradually diminish the demand for gasoline, exerting a potential downward pressure on the index in the years to come.
The refining capacity of various regions is also a critical aspect. Capacity constraints and maintenance schedules can impact gasoline supply, resulting in price volatility. The efficiency of refining processes and the development of new refining technologies affect the production output and cost, which in turn can influence the index. Crude oil prices act as a fundamental driver of the gasoline index, as they represent a significant component of the gasoline manufacturing cost. Fluctuations in crude oil markets, driven by factors like OPEC output decisions, geopolitical events, and speculation, directly influence gasoline prices. Factors such as seasonal demand fluctuations, particularly during peak driving seasons, and the inventory levels of refined products also contribute to short-term price variations. This intricate web of interconnected factors makes predicting precise price movements challenging but understanding these influences is crucial.
Predicting the future direction of the DJ Commodity Unleaded Gasoline Index presents both challenges and opportunities. While a positive outlook could anticipate continued growth in the short term if economic activity strengthens globally and supply chain disruptions ease, the long-term outlook is potentially negative due to the increasing adoption of alternative fuels. The transition to electric vehicles could lead to a sustained decline in demand for gasoline in the coming decades. The risks associated with this prediction include unforeseen geopolitical events, unexpected technological advancements in alternative energy sectors, and a more drastic-than-expected economic slowdown. Potential unforeseen supply chain disruptions or unexpected adjustments in crude oil prices could create volatility and challenge any forecast. The success of government policies aimed at promoting sustainable transportation and the speed of innovation in alternative fuels will have a major impact on the long-term future of the index.
Rating | Short-Term | Long-Term Senior |
---|---|---|
Outlook | Ba3 | Ba1 |
Income Statement | Ba3 | Ba3 |
Balance Sheet | Baa2 | Baa2 |
Leverage Ratios | Ba3 | Baa2 |
Cash Flow | Caa2 | Baa2 |
Rates of Return and Profitability | Baa2 | C |
*An aggregate rating for an index summarizes the overall sentiment towards the companies it includes. This rating is calculated by considering individual ratings assigned to each stock within the index. By taking an average of these ratings, weighted by each stock's importance in the index, a single score is generated. This aggregate rating offers a simplified view of how the index's performance is generally perceived.
How does neural network examine financial reports and understand financial state of the company?
References
- L. Panait and S. Luke. Cooperative multi-agent learning: The state of the art. Autonomous Agents and Multi-Agent Systems, 11(3):387–434, 2005.
- Z. Wang, T. Schaul, M. Hessel, H. van Hasselt, M. Lanctot, and N. de Freitas. Dueling network architectures for deep reinforcement learning. In Proceedings of the International Conference on Machine Learning (ICML), pages 1995–2003, 2016.
- T. Morimura, M. Sugiyama, M. Kashima, H. Hachiya, and T. Tanaka. Nonparametric return distribution ap- proximation for reinforcement learning. In Proceedings of the 27th International Conference on Machine Learning, pages 799–806, 2010
- Y. Le Tallec. Robust, risk-sensitive, and data-driven control of Markov decision processes. PhD thesis, Massachusetts Institute of Technology, 2007.
- Jorgenson, D.W., Weitzman, M.L., ZXhang, Y.X., Haxo, Y.M. and Mat, Y.X., 2023. Can Neural Networks Predict Stock Market?. AC Investment Research Journal, 220(44).
- Chow, G. C. (1960), "Tests of equality between sets of coefficients in two linear regressions," Econometrica, 28, 591–605.
- Nie X, Wager S. 2019. Quasi-oracle estimation of heterogeneous treatment effects. arXiv:1712.04912 [stat.ML]