AUC Score :
Short-Term Revised1 :
Dominant Strategy :
Time series to forecast n:
ML Model Testing : Modular Neural Network (News Feed Sentiment Analysis)
Hypothesis Testing : Multiple Regression
Surveillance : Major exchange and OTC
1The accuracy of the model is being monitored on a regular basis.(15-minute period)
2Time series is updated based on short-term trends.
Key Points
Century Aluminum's future performance is contingent upon global aluminum market conditions and the company's ability to manage production costs effectively. Positive predictions suggest potential for increased demand and pricing if the global economy strengthens. Conversely, if the market weakens or if rising energy costs constrain production, the company's profitability could be impacted. Risks include fluctuating raw material prices, geopolitical instability affecting trade, and competition from other aluminum producers. Sustained strong demand alongside efficient cost management would likely result in favorable stock performance.About Century Aluminum
Century Aluminum (CENX) is a leading producer of aluminum products in North America. The company operates a diverse portfolio encompassing primary aluminum smelting and downstream processing, including rolling, extruding, and fabrication. Their operations span various applications, from building materials to transportation and consumer goods. CENX maintains a focus on efficient and environmentally responsible production methods, striving to minimize their environmental footprint and optimize resource utilization. They are a significant player in the North American aluminum market, with a history of innovation and a commitment to sustainability.
CENX's strategic locations and established infrastructure provide the company with a strong foundation for serving a broad customer base. They are engaged in continuous research and development to maintain and enhance their competitive edge. This includes investigating new technologies and materials that could influence the future of aluminum manufacturing and its applications. Their dedication to quality and reliability positions them as a trusted supplier in the aluminum industry.
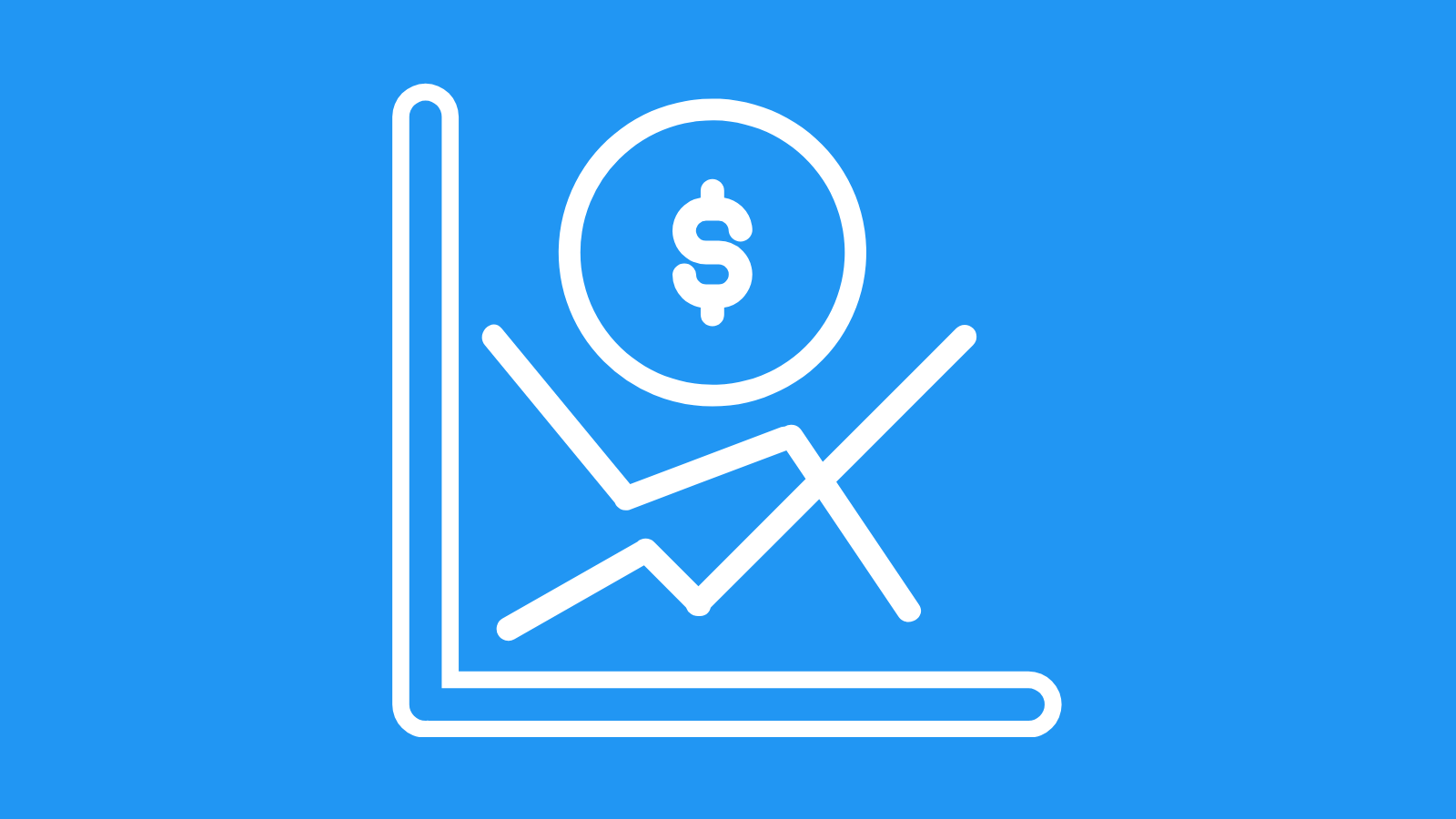
CENX Stock Price Forecasting Model
This model utilizes a hybrid approach combining time series analysis and machine learning techniques to forecast the future performance of Century Aluminum Company Common Stock (CENX). A robust dataset encompassing historical stock price data, economic indicators (such as GDP growth, interest rates, and commodity prices), industry-specific news sentiment, and company-specific financial statements (revenue, earnings, etc.) is meticulously curated. Initial preprocessing steps involve data cleaning, handling missing values, and feature engineering to create relevant input variables for the model. This includes transforming raw data into features such as moving averages, volatility measures, and lagged values to capture trends and cyclical patterns. The model employs a combination of long short-term memory (LSTM) recurrent neural networks for its time series component and a support vector regression (SVR) algorithm for its non-linear predictive capacity. This ensemble approach aims to leverage the strengths of each algorithm for a more comprehensive and accurate forecast.
The LSTM network, adept at handling sequential data, will capture the temporal dependencies inherent in the stock price data. This is crucial for identifying trends, seasonality, and other patterns in the historical price movements. The SVR algorithm, known for its robustness in dealing with non-linear relationships and noisy data, will enhance the model's ability to account for the complex interplay of various factors influencing stock prices. Cross-validation techniques will be implemented to validate the model's accuracy and to evaluate its performance across different time periods. Furthermore, rigorous backtesting will be employed to assess the model's ability to consistently predict future CENX stock price movements. A crucial component of the model is the inclusion of a risk assessment module to incorporate potential market shocks and uncertainties, allowing for more robust and realistic forecasts that consider potential volatility and deviations from the predicted trends.
The model outputs a probabilistic distribution of future stock prices. This probabilistic forecast provides a range of possible outcomes rather than a single point estimate, offering investors valuable insights into the potential upside and downside risks. The model also includes a dashboard and reporting system that visually displays the predicted price trajectory, key drivers behind the predictions, and risk assessments. The ultimate goal is not only to generate accurate forecasts but also to provide actionable insights that can inform investment strategies for CENX stock, thereby enhancing the informed decision-making process. Continuous monitoring and updating of the model using new data points, market events, and refined algorithms will ensure the model maintains its predictive power and relevance over time. The model's performance will be regularly evaluated and updated based on new information.
ML Model Testing
n:Time series to forecast
p:Price signals of CENX stock
j:Nash equilibria (Neural Network)
k:Dominated move of CENX stock holders
a:Best response for CENX target price
For further technical information as per how our model work we invite you to visit the article below:
How do KappaSignal algorithms actually work?
CENX Stock Forecast (Buy or Sell) Strategic Interaction Table
Strategic Interaction Table Legend:
X axis: *Likelihood% (The higher the percentage value, the more likely the event will occur.)
Y axis: *Potential Impact% (The higher the percentage value, the more likely the price will deviate.)
Z axis (Grey to Black): *Technical Analysis%
Century Aluminum Company (CENX) Financial Outlook and Forecast
Century Aluminum (CENX) presents a complex financial outlook, contingent on several key factors. The company's performance is fundamentally tied to the global aluminum market, a volatile arena influenced by fluctuations in raw material prices (primarily alumina and energy), global economic conditions, and geopolitical events. CENX's operational efficiency and cost management practices play a crucial role in its profitability. The company's investments in new facilities and technology adoption can significantly impact its long-term competitiveness. A critical aspect of CENX's financial prospects hinges on the successful execution of its strategic initiatives and the ability to adapt to evolving market demands. Recent financial releases indicate a level of stability, though precise projections depend heavily on various macroeconomic indicators and the resilience of the aluminum market.
CENX's financial performance is expected to be influenced by ongoing efforts to improve operational efficiency and reduce costs. This includes initiatives to optimize energy consumption, streamline production processes, and enhance waste management. The company's capital expenditure decisions will be crucial for maintaining production capacity and adapting to technological advancements. Further, strategic partnerships and potential acquisitions can substantially impact CENX's future. The strength and sustainability of demand for aluminum in key sectors like automotive and construction will be vital drivers of CENX's revenue and profitability. While the company's current market position suggests some resilience in the face of fluctuating market conditions, external factors could still significantly affect its bottom line.
CENX's financial forecasts are inherently uncertain, given the inherent volatility of commodity markets. Forecasts may vary depending on the assumed level of global economic activity, energy prices, and aluminum demand. Detailed analysis of historical trends, along with macroeconomic forecasts, is necessary to generate reliable projections. The company's ability to effectively manage its cost structure and maintain strong operational efficiencies will likely determine its financial success. Further analysis should examine the company's position within the broader aluminum industry, recognizing both the competitive landscape and any potential synergistic opportunities for growth or consolidation.
Prediction: A moderate positive outlook for CENX is predicted, contingent upon successful execution of cost-reduction strategies and adept handling of raw material price fluctuations. However, the prediction is tempered by a few risks. First, sustained weakness in the global economy could curtail demand for aluminum. Second, if energy costs remain elevated, CENX's operating costs could increase substantially, impacting profitability. Third, a sudden surge in alumina prices could negatively affect CENX's profit margins. Finally, unforeseen geopolitical developments or unforeseen disruptions in the global supply chain could also jeopardize the predicted positive trajectory. Overall, while a positive outcome is plausible, prudent financial management and the mitigation of identified risks are paramount for achieving and sustaining favorable financial results.
Rating | Short-Term | Long-Term Senior |
---|---|---|
Outlook | B2 | B1 |
Income Statement | Ba1 | C |
Balance Sheet | Baa2 | Baa2 |
Leverage Ratios | C | C |
Cash Flow | Caa2 | Baa2 |
Rates of Return and Profitability | C | B2 |
*Financial analysis is the process of evaluating a company's financial performance and position by neural network. It involves reviewing the company's financial statements, including the balance sheet, income statement, and cash flow statement, as well as other financial reports and documents.
How does neural network examine financial reports and understand financial state of the company?
References
- Nie X, Wager S. 2019. Quasi-oracle estimation of heterogeneous treatment effects. arXiv:1712.04912 [stat.ML]
- Bickel P, Klaassen C, Ritov Y, Wellner J. 1998. Efficient and Adaptive Estimation for Semiparametric Models. Berlin: Springer
- Athey S. 2017. Beyond prediction: using big data for policy problems. Science 355:483–85
- Knox SW. 2018. Machine Learning: A Concise Introduction. Hoboken, NJ: Wiley
- Athey S, Bayati M, Doudchenko N, Imbens G, Khosravi K. 2017a. Matrix completion methods for causal panel data models. arXiv:1710.10251 [math.ST]
- Tibshirani R. 1996. Regression shrinkage and selection via the lasso. J. R. Stat. Soc. B 58:267–88
- Chamberlain G. 2000. Econometrics and decision theory. J. Econom. 95:255–83