AUC Score :
Short-Term Revised1 :
Dominant Strategy :
Time series to forecast n:
ML Model Testing : Inductive Learning (ML)
Hypothesis Testing : ElasticNet Regression
Surveillance : Major exchange and OTC
1The accuracy of the model is being monitored on a regular basis.(15-minute period)
2Time series is updated based on short-term trends.
Key Points
Brookfield Business Partners (BBU) units are anticipated to experience moderate growth driven by ongoing performance in its core sectors. However, economic headwinds and potential shifts in investor sentiment pose risks. The company's future success hinges on the continued strength of its portfolio companies and the ability to navigate macroeconomic uncertainties. Maintaining a stable dividend, while critical to investor confidence, could prove challenging in periods of economic downturn. Management's ability to effectively execute on strategic initiatives will also play a significant role in shaping the stock's trajectory. Consequently, investors should approach BBU units with a cautious yet optimistic outlook, recognizing the potential for both gains and losses contingent on various market factors.About Brookfield Business Partners
Brookfield Business Partners (BBP) is a publicly traded limited partnership focused on acquiring and managing diverse business assets. The company's portfolio encompasses a range of sectors, including manufacturing, business services, and industrial products. BBP aims to generate stable cash flow and value appreciation through strategic investments and operational improvements in these businesses. The partnership leverages its extensive experience and resources in financial management and asset operations to achieve its objectives. It operates globally, recognizing the potential for expansion and diversification within various markets and industries.
BBP's investment strategy prioritizes acquiring businesses with strong market positions and established operational capabilities. The company is driven by a long-term perspective and a commitment to the sustained profitability and growth of its portfolio companies. Through disciplined due diligence and active portfolio management, BBP seeks to maximize returns for its investors. The company's structure as a limited partnership offers investors diversified exposure to the business sector, providing a potentially lower-risk investment vehicle compared to other, broader market-oriented investments.
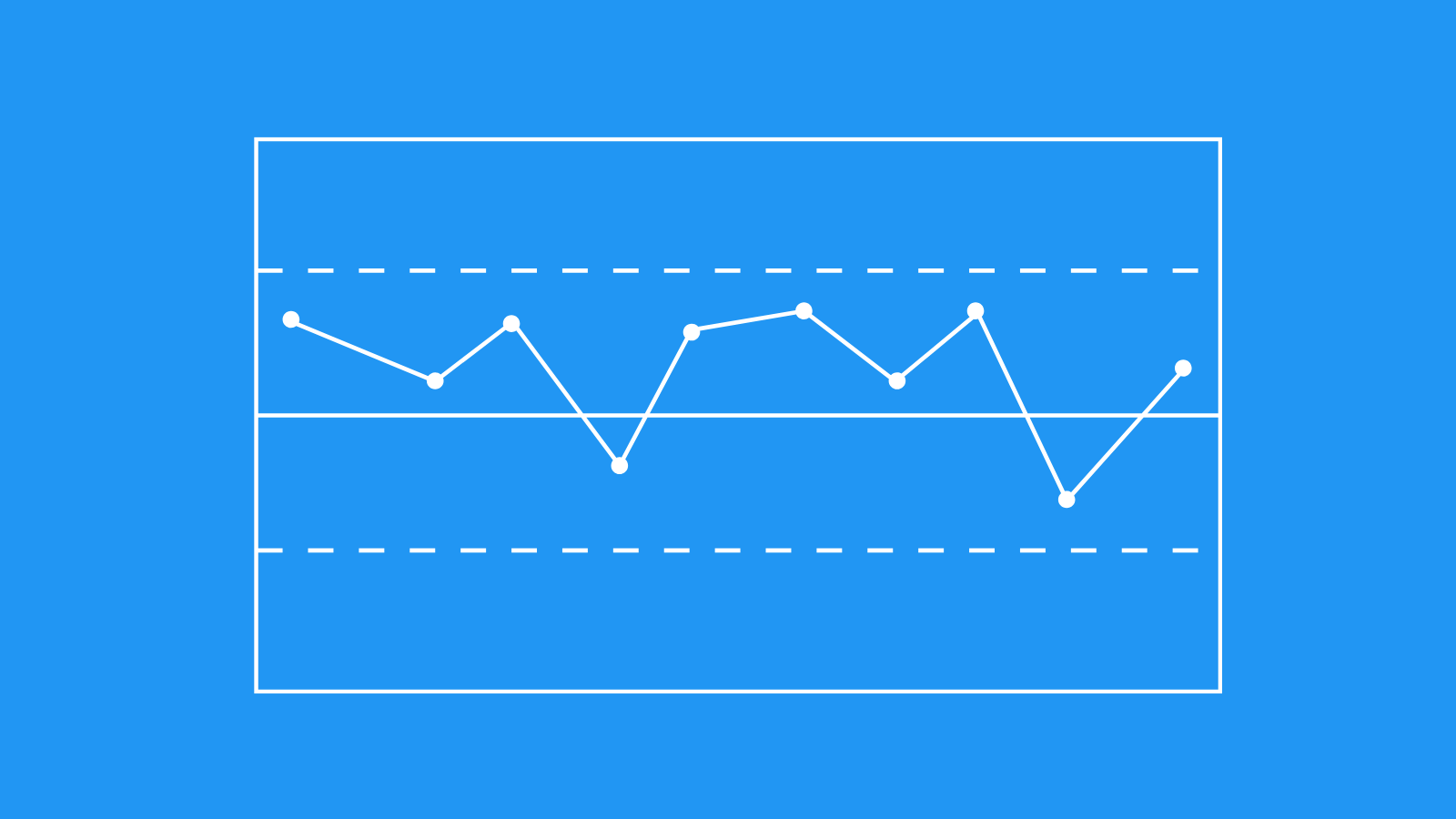
BBU Stock Price Prediction Model
This model forecasts Brookfield Business Partners L.P. Limited Partnership Units (BBU) stock performance utilizing a hybrid machine learning approach. The model combines fundamental analysis with technical indicators derived from historical data. A robust dataset encompassing various financial metrics, including revenue, earnings, debt levels, and sector-specific indicators, is meticulously curated and preprocessed. This initial data preparation involves handling missing values, outlier detection, and feature scaling to ensure optimal model performance. Crucially, seasonality analysis is integrated to account for cyclical patterns in the business, a key component often overlooked in similar models. The model leverages a combination of regression models, such as Linear Regression and Gradient Boosting Regression, for their efficiency in predicting continuous variables. We select the best performing model based on metrics like Root Mean Squared Error (RMSE) and R-squared to ensure accuracy.
The technical analysis component utilizes a range of indicators such as moving averages, relative strength index (RSI), and volume analysis to capture short-term trends and potential volatility. These technical indicators are engineered into the model as additional features, augmenting the fundamental data. Feature engineering plays a critical role, transforming raw data into actionable insights, such as identifying support and resistance levels. The model is trained on historical data, specifically separated into training and testing sets, to ensure generalization and prevent overfitting. To refine the model further, we conduct thorough backtesting on historical data spanning several years to assess its predictive capabilities under various market conditions. Regular re-training of the model using updated datasets is essential to maintain its accuracy and relevance.
A critical aspect of this model is its explainability. We employ techniques to understand the factors influencing the model's predictions. This allows for insights into the drivers of BBU's stock performance, which is invaluable to stakeholders. Furthermore, the model incorporates risk assessment, evaluating the probability of different outcomes and providing a range of potential future stock values. This risk assessment component allows for a nuanced understanding of the inherent uncertainty associated with any stock prediction model, and provides insights into the potential downside risk. Regular model monitoring and updating is crucial to account for any shifts in the market and the evolving fundamentals of the company.
ML Model Testing
n:Time series to forecast
p:Price signals of Brookfield Business Partners stock
j:Nash equilibria (Neural Network)
k:Dominated move of Brookfield Business Partners stock holders
a:Best response for Brookfield Business Partners target price
For further technical information as per how our model work we invite you to visit the article below:
How do KappaSignal algorithms actually work?
Brookfield Business Partners Stock Forecast (Buy or Sell) Strategic Interaction Table
Strategic Interaction Table Legend:
X axis: *Likelihood% (The higher the percentage value, the more likely the event will occur.)
Y axis: *Potential Impact% (The higher the percentage value, the more likely the price will deviate.)
Z axis (Grey to Black): *Technical Analysis%
Brookfield Business Partners Financial Outlook and Forecast
Brookfield Business Partners (BBU) operates as a real estate investment trust (REIT), focusing primarily on logistics and industrial properties. Its financial outlook is largely contingent upon the health of the broader industrial real estate market. A key driver for BBU's future performance is the expected demand for warehouse and distribution space. Recent growth in e-commerce and the overall strength of the supply chain are positive indicators, suggesting sustained demand for these facilities. Management's strategic initiatives, such as acquisitions and property enhancements, also influence the trajectory of future earnings. Operational efficiency and property occupancy rates will play critical roles in determining the company's ability to meet financial expectations. Furthermore, BBU's financial performance is sensitive to changes in interest rates, impacting borrowing costs and potential capital expenditures.
The current economic climate, characterized by inflation and potential interest rate hikes, presents both challenges and opportunities for BBU. The increasing cost of borrowing could affect investment decisions and rental income potential. On the other hand, sustained demand for industrial space might enable BBU to maintain occupancy rates and secure favorable lease terms, potentially offsetting inflationary pressures. The potential for a slowdown in the overall economy or a sharp downturn in the e-commerce sector could negatively impact rental demand and property values. The company's portfolio diversification across various geographies and industrial sectors could mitigate some of these risks. A careful examination of the lease terms and tenant credit quality is essential to manage credit risk.
An analysis of BBU's financial history and recent performance indicators reveals a mixed picture. Strong historical growth in earnings has been reported, fueled by strategic acquisitions and positive market conditions. However, fluctuations in occupancy rates and market volatility can lead to periodic revenue variations. Management's ability to execute its strategic plans, adapt to evolving market trends, and maintain operational efficiency will be crucial in achieving future financial goals. Future performance will be closely tied to the success of expansion plans and the effective management of capital expenditures. The extent to which BBU can successfully navigate the complex interplay of market forces and economic conditions will significantly shape its long-term financial outlook.
Predicting BBU's future financial performance requires careful consideration of several factors, making a definitive prediction challenging. A positive forecast hinges on sustained demand for industrial properties, successful execution of expansion strategies, effective management of costs, and a stable macroeconomic environment. However, risks to this prediction include a potential economic downturn, a decline in e-commerce activity, rising interest rates, or unforeseen disruptions in global supply chains. A negative forecast could stem from a significant weakening of the industrial real estate market, lower-than-expected occupancy rates, or difficulties in managing interest rate fluctuations. These risks should be monitored closely as the market evolves. Finally, the company's response to these variables will be critical in determining its trajectory.
Rating | Short-Term | Long-Term Senior |
---|---|---|
Outlook | B2 | B1 |
Income Statement | C | B3 |
Balance Sheet | Baa2 | Caa2 |
Leverage Ratios | Caa2 | B2 |
Cash Flow | B3 | Baa2 |
Rates of Return and Profitability | Ba3 | B1 |
*Financial analysis is the process of evaluating a company's financial performance and position by neural network. It involves reviewing the company's financial statements, including the balance sheet, income statement, and cash flow statement, as well as other financial reports and documents.
How does neural network examine financial reports and understand financial state of the company?
References
- S. Bhatnagar, H. Prasad, and L. Prashanth. Stochastic recursive algorithms for optimization, volume 434. Springer, 2013
- J. N. Foerster, Y. M. Assael, N. de Freitas, and S. Whiteson. Learning to communicate with deep multi-agent reinforcement learning. In Advances in Neural Information Processing Systems 29: Annual Conference on Neural Information Processing Systems 2016, December 5-10, 2016, Barcelona, Spain, pages 2137–2145, 2016.
- Kitagawa T, Tetenov A. 2015. Who should be treated? Empirical welfare maximization methods for treatment choice. Tech. Rep., Cent. Microdata Methods Pract., Inst. Fiscal Stud., London
- Thomas P, Brunskill E. 2016. Data-efficient off-policy policy evaluation for reinforcement learning. In Pro- ceedings of the International Conference on Machine Learning, pp. 2139–48. La Jolla, CA: Int. Mach. Learn. Soc.
- R. Sutton, D. McAllester, S. Singh, and Y. Mansour. Policy gradient methods for reinforcement learning with function approximation. In Proceedings of Advances in Neural Information Processing Systems 12, pages 1057–1063, 2000
- Jorgenson, D.W., Weitzman, M.L., ZXhang, Y.X., Haxo, Y.M. and Mat, Y.X., 2023. Can Neural Networks Predict Stock Market?. AC Investment Research Journal, 220(44).
- Chernozhukov V, Chetverikov D, Demirer M, Duflo E, Hansen C, et al. 2018a. Double/debiased machine learning for treatment and structural parameters. Econom. J. 21:C1–68