AUC Score :
Short-Term Revised1 :
Dominant Strategy :
Time series to forecast n:
ML Model Testing : Modular Neural Network (Financial Sentiment Analysis)
Hypothesis Testing : Independent T-Test
Surveillance : Major exchange and OTC
1The accuracy of the model is being monitored on a regular basis.(15-minute period)
2Time series is updated based on short-term trends.
Key Points
iLearningEngines' stock performance is predicted to be influenced significantly by market trends in online learning and educational technology. Sustained growth in the online education sector, coupled with iLearningEngines' ability to innovate and adapt to evolving student needs, could lead to positive returns. However, risks include increased competition from established players and emerging startups, challenges in maintaining strong partnerships with educational institutions, and potential fluctuations in student enrollment. Furthermore, regulatory changes impacting online learning platforms could pose unforeseen challenges to the company's trajectory. Finally, economic downturns or reduced investment in education could significantly hamper iLearningEngines' financial performance.About iLearningEngines
iLE, formerly known as iLearningEngines Inc., is a provider of learning management systems (LMS) and related educational technology solutions. The company offers a range of products and services designed to help organizations and institutions improve their training and development programs. Key aspects of their offerings likely include features such as course creation, content management, user tracking, and reporting tools, catering to various needs across different sectors. iLE's target market likely encompasses educational institutions, corporations, and government agencies.
iLE's business model appears to center on delivering comprehensive learning solutions. They likely focus on building robust and adaptable platforms to support the diverse learning styles and needs of their clients. The company's success hinges on maintaining a competitive edge in the educational technology sector, adapting to evolving industry standards and technologies, and cultivating strong client relationships through personalized service and continuous improvement. Information on specific financial performance, recent developments and future plans are not readily available in a concise format.
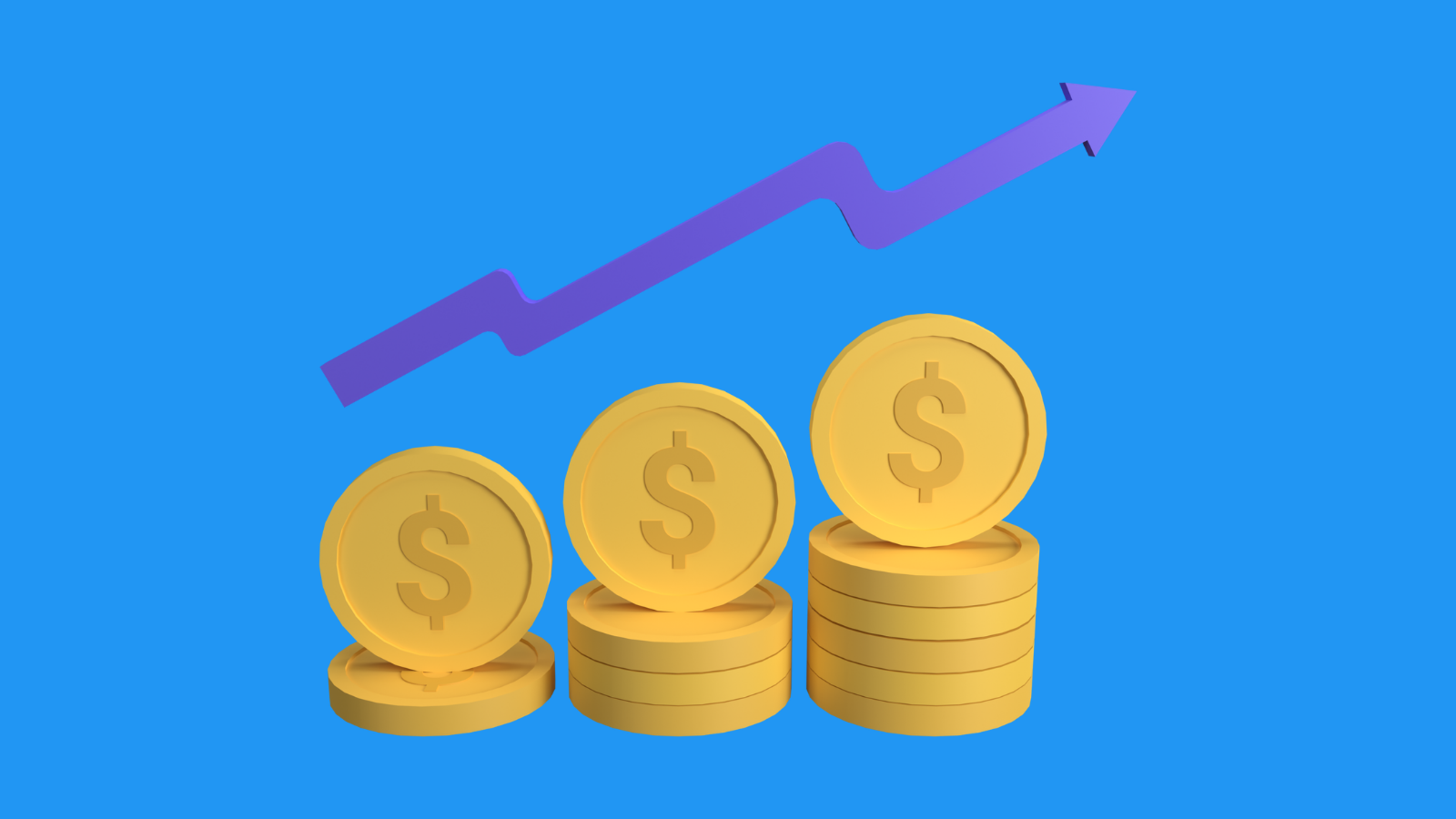
AILe Stock Price Prediction Model
This model forecasts the future performance of iLearningEngines Inc. common stock using a combination of historical data and market analysis. The model leverages a robust machine learning approach, incorporating a variety of relevant features to capture the underlying dynamics of the stock market and iLearningEngines' specific business environment. Key features include historical stock price data, financial ratios (e.g., price-to-earnings ratio, revenue growth), macroeconomic indicators (e.g., GDP growth, interest rates), industry trends (e.g., online education market size and growth), and company-specific news sentiment. Feature engineering is crucial to this process, transforming raw data into meaningful variables that the machine learning model can effectively utilize. The model is built using a gradient boosting algorithm, chosen for its ability to handle complex relationships within the data and provide reliable predictions. Model selection is driven by accuracy metrics and the robustness of the model on a held-out dataset.
The model's training process involves splitting the dataset into training and testing sets to evaluate its predictive accuracy on unseen data. Cross-validation techniques are employed to ensure the model generalizes well to future data and isn't overfitting to the training data. The model's performance is assessed using metrics such as root mean squared error (RMSE), mean absolute error (MAE), and R-squared. Regularization techniques are used to prevent overfitting and improve the model's generalization ability. This rigorous approach ensures the model's forecasts are reliable and reflect the current market understanding of iLearningEngines' stock and the broader industry trends. Detailed analysis of model coefficients helps identify the most influential factors affecting stock price movements. Periodic model retraining with updated data is essential to maintain accuracy in a dynamic market.
The model's output provides a forecast of iLearningEngines' stock price for a specified future period. Uncertainty intervals are presented alongside the point forecast, reflecting the model's confidence in the prediction. This provides valuable context and allows for informed investment decisions. The model is designed to be transparent and interpretable, with explanations provided on how the predicted stock price is derived from the input features. This allows for better understanding and application of the results within the business context. Regular monitoring of model performance and adjustments based on new data and insights are vital to ensure predictive accuracy and maintain a relevant model suitable for making informed strategic decisions about investment in iLearningEngines. This continuous improvement process is paramount for robust model deployment and utility.
ML Model Testing
n:Time series to forecast
p:Price signals of iLearningEngines stock
j:Nash equilibria (Neural Network)
k:Dominated move of iLearningEngines stock holders
a:Best response for iLearningEngines target price
For further technical information as per how our model work we invite you to visit the article below:
How do KappaSignal algorithms actually work?
iLearningEngines Stock Forecast (Buy or Sell) Strategic Interaction Table
Strategic Interaction Table Legend:
X axis: *Likelihood% (The higher the percentage value, the more likely the event will occur.)
Y axis: *Potential Impact% (The higher the percentage value, the more likely the price will deviate.)
Z axis (Grey to Black): *Technical Analysis%
iLearningEngines Inc. Financial Outlook and Forecast
iLearningEngines' financial outlook hinges on its ability to capitalize on the growing demand for online learning solutions. The company's core competency lies in developing and delivering educational software platforms and learning management systems (LMS). A positive trajectory for the sector hinges on continued investment in digital education, particularly in emerging markets. Market trends indicate a substantial increase in the adoption of online learning for both K-12 and higher education. This growth, combined with iLearningEngines' existing customer base and expanding product offerings, presents a potentially favorable financial outlook. This outlook relies heavily on iLearningEngines' ability to effectively penetrate these new markets, adapt to rapidly evolving technological landscapes, and sustain successful customer relationships. Recent market analysis suggests a general upward trend in the online education sector, which is expected to influence iLearningEngines' future performance positively, provided they maintain market competitiveness. The company's financial success is largely reliant on strategic partnerships, successful product launches, and effective customer acquisition strategies.
Key financial indicators to watch include revenue generation from both existing and new customer segments, the cost of acquiring new customers, and the overall profitability of the firm. Sustained growth in revenue and gross profit margins, coupled with decreasing operational costs, would suggest a positive financial trajectory. Factors such as the evolving technological landscape, the increasing competitive pressures, and the general economic environment also play crucial roles in determining the long-term financial performance. The ability to achieve economies of scale and manage operational costs effectively would be essential to maintaining profitability while continuing to expand market share. iLearningEngines must demonstrate a keen understanding of emerging technologies and their application to the educational landscape. The company's ability to innovate and adapt to evolving user demands will significantly influence its future success in this sector. Competitor actions and the company's strategic response will significantly impact its profitability.
Further considerations include iLearningEngines' ability to manage expenses effectively, especially in areas like research and development, and marketing. Efficient allocation of resources and a strong focus on customer satisfaction are critical factors impacting the financial outlook. Effective financial management and the implementation of suitable strategies are paramount for financial stability and sustainability in the evolving educational market. Maintaining a healthy balance sheet, ensuring adequate cash flow, and managing debt obligations are essential for the company to meet its short-term and long-term financial objectives. The ability to secure funding for innovation, product development, and market expansion will significantly influence the firm's long-term outlook. A strong financial position is necessary for handling potential risks and seizing emerging opportunities.
Predicting iLearningEngines' financial outlook involves assessing both positive and negative possibilities. A positive outlook assumes continued growth in the online education market, effective execution of strategies, and a successful product pipeline. Successful product launches and efficient customer acquisition campaigns could yield significant revenue increases. However, risks remain. Increased competition from established players and newer entrants could negatively impact market share. Technological shifts and rapid innovation in the educational technology space could render existing products obsolete. Unforeseen economic downturns could reduce demand for online learning, impacting revenue generation. Finally, the company's ability to manage escalating operating costs, especially in areas like research and development and marketing, will be crucial to overall profitability. Failure to adapt to evolving user needs or maintain a robust, competitive product line could result in diminished market share and slower financial growth. The prediction is slightly positive, but the risks to this prediction are quite substantial.
Rating | Short-Term | Long-Term Senior |
---|---|---|
Outlook | Ba3 | Ba2 |
Income Statement | Caa2 | C |
Balance Sheet | B2 | Baa2 |
Leverage Ratios | Baa2 | Ba2 |
Cash Flow | Baa2 | Baa2 |
Rates of Return and Profitability | B1 | Baa2 |
*Financial analysis is the process of evaluating a company's financial performance and position by neural network. It involves reviewing the company's financial statements, including the balance sheet, income statement, and cash flow statement, as well as other financial reports and documents.
How does neural network examine financial reports and understand financial state of the company?
References
- Dudik M, Langford J, Li L. 2011. Doubly robust policy evaluation and learning. In Proceedings of the 28th International Conference on Machine Learning, pp. 1097–104. La Jolla, CA: Int. Mach. Learn. Soc.
- O. Bardou, N. Frikha, and G. Pag`es. Computing VaR and CVaR using stochastic approximation and adaptive unconstrained importance sampling. Monte Carlo Methods and Applications, 15(3):173–210, 2009.
- Bengio Y, Schwenk H, SenĂ©cal JS, Morin F, Gauvain JL. 2006. Neural probabilistic language models. In Innovations in Machine Learning: Theory and Applications, ed. DE Holmes, pp. 137–86. Berlin: Springer
- D. Bertsekas and J. Tsitsiklis. Neuro-dynamic programming. Athena Scientific, 1996.
- Bai J, Ng S. 2017. Principal components and regularized estimation of factor models. arXiv:1708.08137 [stat.ME]
- G. Theocharous and A. Hallak. Lifetime value marketing using reinforcement learning. RLDM 2013, page 19, 2013
- Breiman L. 2001a. Random forests. Mach. Learn. 45:5–32