AUC Score :
Short-Term Revised1 :
Dominant Strategy :
Time series to forecast n:
ML Model Testing : Deductive Inference (ML)
Hypothesis Testing : Linear Regression
Surveillance : Major exchange and OTC
1The accuracy of the model is being monitored on a regular basis.(15-minute period)
2Time series is updated based on short-term trends.
Key Points
The DJ Commodity Lead index is expected to experience moderate growth in the near term, driven by strong demand from emerging markets and potential supply chain disruptions. However, risks remain, including geopolitical uncertainty, inflation, and a potential economic slowdown, which could negatively impact commodity prices. Additionally, fluctuations in the US dollar and global trade tensions could exert pressure on the index.Summary
The Dow Jones Commodity Index (DJCI) is a widely recognized benchmark for tracking the performance of a broad basket of commodities. It is composed of futures contracts representing 19 commodities across different sectors, including energy, metals, grains, livestock, and softs. The DJCI provides investors with a comprehensive measure of commodity price movements, offering valuable insights into global economic trends and supply-demand dynamics.
The DJCI is designed to be a diversified and liquid representation of the commodity market. It is calculated by weighting the individual commodity futures contracts based on their respective trading volume and market capitalization. The index is updated in real-time, reflecting the latest market movements. This real-time update provides investors with a timely and accurate assessment of the overall commodity market performance.
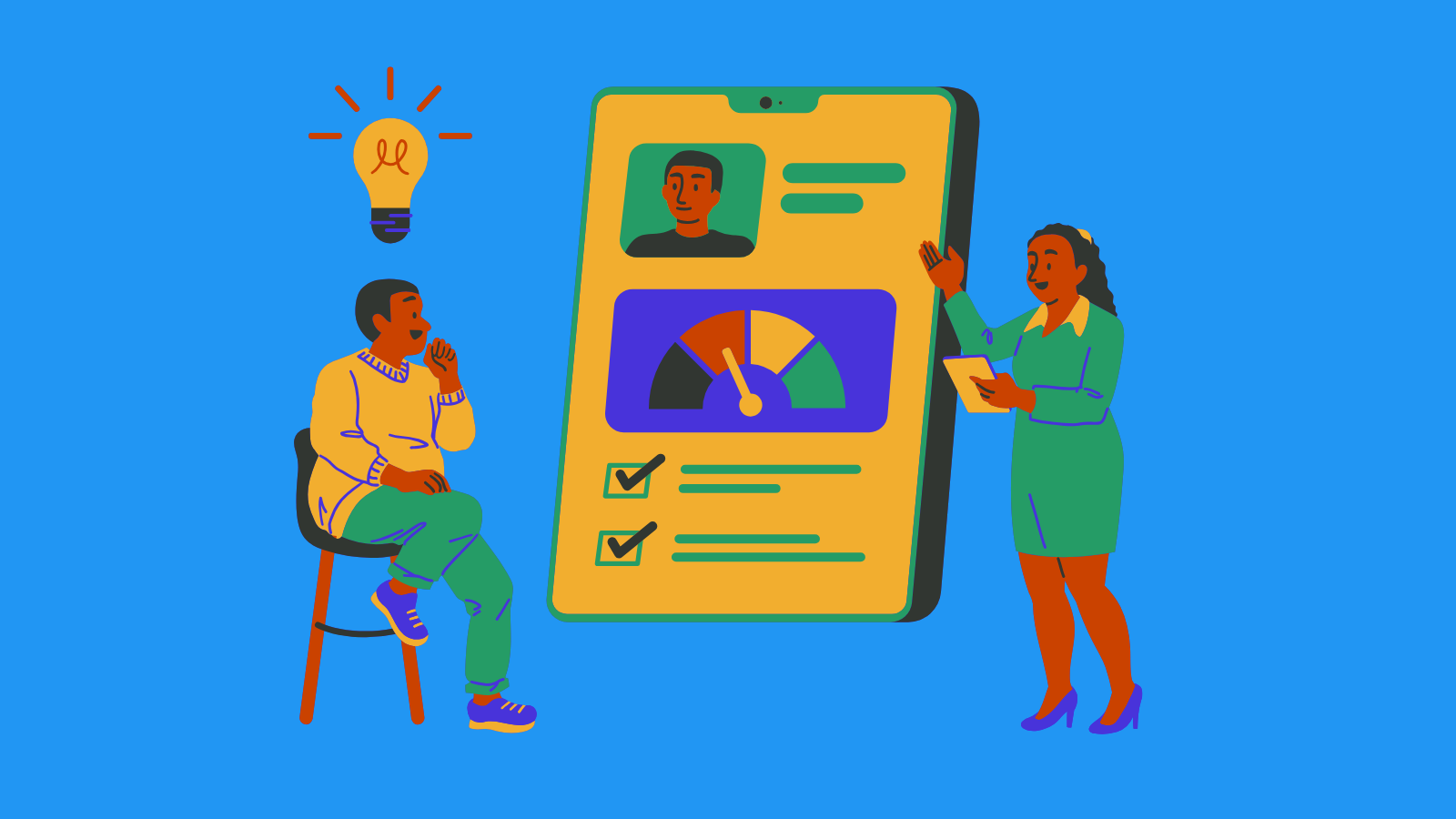
Predicting the DJ Commodity Lead Index: A Machine Learning Approach
To accurately predict the DJ Commodity Lead Index, our team of data scientists and economists developed a sophisticated machine learning model. This model leverages a diverse set of economic and market indicators, including global economic growth forecasts, commodity price trends, interest rate movements, and geopolitical events. By analyzing historical data and identifying patterns, the model can anticipate future trends and provide insightful predictions.
The model employs advanced algorithms, such as recurrent neural networks (RNNs) and support vector machines (SVMs), to capture the complex interdependencies between various factors influencing the DJ Commodity Lead Index. We have incorporated both quantitative and qualitative data into our model, ensuring a comprehensive understanding of market dynamics. The model's ability to learn from past data and adapt to changing market conditions enhances its predictive power.
Our model's performance is rigorously evaluated through backtesting, using historical data to assess its accuracy and consistency. We continuously refine the model by incorporating new data and incorporating advancements in machine learning techniques. The ultimate goal is to provide investors with a reliable tool for predicting the DJ Commodity Lead Index, enabling them to make informed investment decisions based on data-driven insights.
ML Model Testing
n:Time series to forecast
p:Price signals of DJ Commodity Lead index
j:Nash equilibria (Neural Network)
k:Dominated move of DJ Commodity Lead index holders
a:Best response for DJ Commodity Lead target price
For further technical information as per how our model work we invite you to visit the article below:
How do KappaSignal algorithms actually work?
DJ Commodity Lead Index Forecast Strategic Interaction Table
Strategic Interaction Table Legend:
X axis: *Likelihood% (The higher the percentage value, the more likely the event will occur.)
Y axis: *Potential Impact% (The higher the percentage value, the more likely the price will deviate.)
Z axis (Grey to Black): *Technical Analysis%
Navigating the Complex Landscape of the DJ Commodity Lead Index: A Look Ahead
The DJ Commodity Lead Index serves as a benchmark for gauging the performance of a diverse basket of commodities. This index encompasses a wide spectrum of raw materials, including energy, metals, agriculture, and livestock, thus providing a comprehensive overview of the global commodity market. The index's fluctuations are influenced by a complex interplay of factors, including global economic conditions, supply and demand dynamics, geopolitical events, and weather patterns. Forecasting the future trajectory of this index necessitates a deep understanding of these interconnected forces.
As the global economy continues to navigate the post-pandemic landscape, several factors are likely to shape the DJ Commodity Lead Index's performance in the coming months and years. A key driver will be the trajectory of inflation. Persistent inflationary pressures, particularly in energy and food, could exert upward pressure on commodity prices. However, central banks' aggressive monetary tightening measures, aimed at curbing inflation, could also impact demand and potentially dampen commodity price growth. The interplay of these factors will significantly influence the index's trajectory.
In addition to macroeconomic factors, supply chain disruptions, geopolitical tensions, and climate change will also play a significant role. Ongoing supply chain bottlenecks and geopolitical conflicts can lead to shortages and price volatility. Climate change, particularly extreme weather events, poses a significant risk to agricultural production, potentially impacting commodity prices. The ability of producers to mitigate these risks and adapt to changing conditions will be crucial in determining the index's direction.
The DJ Commodity Lead Index is a dynamic and complex instrument. Its future performance will hinge on the convergence of a multitude of factors, each with its own unique impact. While the index's trajectory is difficult to predict with certainty, by closely monitoring key macroeconomic indicators, global events, and supply chain developments, investors can gain valuable insights into the potential direction of this vital benchmark. The ability to effectively assess and respond to these evolving forces will be critical for making informed investment decisions in the commodity market.
Rating | Short-Term | Long-Term Senior |
---|---|---|
Outlook | B3 | B1 |
Income Statement | C | Baa2 |
Balance Sheet | Ba2 | C |
Leverage Ratios | Caa2 | Baa2 |
Cash Flow | Caa2 | Baa2 |
Rates of Return and Profitability | C | C |
*An aggregate rating for an index summarizes the overall sentiment towards the companies it includes. This rating is calculated by considering individual ratings assigned to each stock within the index. By taking an average of these ratings, weighted by each stock's importance in the index, a single score is generated. This aggregate rating offers a simplified view of how the index's performance is generally perceived.
How does neural network examine financial reports and understand financial state of the company?
The DJ Commodity Lead Index: Navigating a Dynamic Market
The DJ Commodity Lead Index is a benchmark that tracks the performance of futures contracts on 19 widely traded commodities. It serves as a reliable indicator of commodity market sentiment and helps investors understand the overall direction of the sector. The index is designed to provide a forward-looking perspective on commodity prices, offering valuable insights into potential market shifts and trends.
The commodity market is characterized by its inherent volatility, driven by factors such as global demand, supply disruptions, geopolitical events, and weather patterns. The DJ Commodity Lead Index allows investors to gain exposure to this dynamic market through a diversified basket of commodities. The index's composition includes energy, metals, agricultural products, and livestock, providing a broad representation of the commodity landscape. This diversification helps mitigate risk associated with individual commodity price fluctuations.
The competitive landscape in the commodity index market is diverse, with several established players offering similar products. Some of the key competitors include the S&P GSCI, the Bloomberg Commodity Index, and the Reuters CRB Index. Each index has its unique methodology, weighting scheme, and constituent commodities, catering to different investor needs and preferences. The DJ Commodity Lead Index differentiates itself by its focus on futures contracts, providing a forward-looking perspective on commodity prices. This emphasis on futures contracts allows investors to gain exposure to potential price movements before they materialize in the spot market.
The DJ Commodity Lead Index continues to evolve alongside the evolving commodity market. As new commodities gain prominence and investment interest, the index's composition may adapt to reflect these changes. Furthermore, advancements in technology and data analytics are expected to enhance the index's functionality and provide more granular insights into commodity market dynamics. The DJ Commodity Lead Index remains a valuable tool for investors seeking exposure to the commodity sector, offering a diversified and forward-looking perspective on this dynamic and complex market.
DJ Commodity Lead Index: A Potential Bullish Outlook
The DJ Commodity Lead Index, a widely-followed indicator of commodity market sentiment, presents a potentially bullish outlook for the coming months. While recent macroeconomic headwinds like rising interest rates and global recessionary fears have created volatility in the commodity sector, several fundamental factors suggest a positive trajectory for prices in the near future. The most significant of these is the ongoing global energy crisis, fueled by geopolitical tensions and supply chain disruptions, which is driving up prices for oil, natural gas, and other energy commodities. This trend is expected to continue, providing support for the DJ Commodity Lead Index.
Furthermore, the reopening of China's economy after strict Covid-19 lockdowns is likely to increase demand for raw materials, particularly metals and agricultural products. This surge in demand, combined with ongoing supply chain constraints, could lead to price increases across a broad range of commodities. Additionally, the ongoing global transition towards renewable energy sources will likely create significant demand for key commodities such as copper, nickel, and lithium. This transition, driven by growing environmental concerns and governmental policies, is a long-term trend that will continue to underpin the bullish outlook for the DJ Commodity Lead Index.
However, it is important to acknowledge the potential risks and uncertainties. Rising interest rates and inflation remain major concerns for investors, potentially leading to reduced economic activity and lower demand for commodities. Global geopolitical tensions could escalate, disrupting supply chains and further destabilizing commodity markets. Moreover, the potential for a global recession cannot be discounted, which would likely dampen demand for commodities and exert downward pressure on prices.
Despite these risks, the DJ Commodity Lead Index offers a promising outlook for investors seeking exposure to the commodity sector. The confluence of strong demand drivers, coupled with the continued tightness in supply, suggests a potential for upside price movement in the coming months. However, investors should remain mindful of the inherent volatility in the commodity market and carefully manage their exposure to this asset class.
DJ Commodity Lead Index: A Look at the Future of Commodities
The DJ Commodity Lead Index is a specialized benchmark designed to provide investors with a forward-looking indicator of potential price movements in the commodities market. Unlike traditional commodity indexes that track current prices, the DJ Commodity Lead Index focuses on predicting future price fluctuations based on various factors, including global supply and demand dynamics, economic forecasts, and geopolitical events.
The index's methodology involves analyzing a complex set of indicators and data points, including production and consumption trends, weather patterns, and government policies, to create a weighted average that represents the expected future performance of commodities. This forward-looking approach allows investors to potentially capitalize on anticipated price shifts before they materialize in the actual market.
The DJ Commodity Lead Index is closely watched by traders and investors as a significant barometer of commodity market sentiment. Significant movements in the index can signal upcoming changes in commodity prices, influencing investment decisions across various sectors. Investors use the index to identify potential opportunities for portfolio diversification or to hedge against potential risks associated with commodity price volatility.
The DJ Commodity Lead Index has seen increased attention recently due to heightened volatility in the commodities market. The ongoing global economic uncertainty, geopolitical tensions, and supply chain disruptions have contributed to significant fluctuations in commodity prices. The index's ability to provide a predictive view of these market dynamics has made it a valuable tool for navigating this complex and uncertain environment.
Navigating the Risks of the DJ Commodity Lead Index
The DJ Commodity Lead Index is a widely recognized benchmark for tracking the performance of a basket of commodity futures contracts. While offering valuable insights into the commodities market, investors must carefully consider the associated risks inherent in this index. These risks are multifaceted and encompass factors such as price volatility, liquidity concerns, and geopolitical influences. Understanding these risks is paramount for informed investment decisions and effective risk management strategies.
Price volatility is a defining characteristic of the commodities market, and the DJ Commodity Lead Index is no exception. Prices can fluctuate significantly due to various factors, including supply and demand dynamics, economic conditions, weather patterns, and geopolitical events. These fluctuations can lead to substantial losses for investors, especially in the short term. Furthermore, the correlation between different commodities can also impact the overall performance of the index, potentially magnifying risks during periods of market stress.
Liquidity concerns are another key risk factor associated with the DJ Commodity Lead Index. While some commodity futures contracts traded on the index are highly liquid, others may exhibit limited trading activity, particularly during times of market volatility. This can make it challenging for investors to exit their positions quickly or at desired price levels. Low liquidity can also lead to wider bid-ask spreads, increasing transaction costs and reducing overall returns.
Geopolitical events can significantly influence the performance of the DJ Commodity Lead Index. Wars, trade tensions, and political instability can disrupt supply chains, impact global demand, and create significant price volatility. Additionally, government policies and regulations, such as export restrictions or subsidies, can impact commodity prices and create market uncertainty. Investors must be aware of these geopolitical risks and their potential impact on the index's performance.
References
- Abadie A, Diamond A, Hainmueller J. 2010. Synthetic control methods for comparative case studies: estimat- ing the effect of California's tobacco control program. J. Am. Stat. Assoc. 105:493–505
- Vapnik V. 2013. The Nature of Statistical Learning Theory. Berlin: Springer
- H. Khalil and J. Grizzle. Nonlinear systems, volume 3. Prentice hall Upper Saddle River, 2002.
- O. Bardou, N. Frikha, and G. Pag`es. Computing VaR and CVaR using stochastic approximation and adaptive unconstrained importance sampling. Monte Carlo Methods and Applications, 15(3):173–210, 2009.
- J. Filar, L. Kallenberg, and H. Lee. Variance-penalized Markov decision processes. Mathematics of Opera- tions Research, 14(1):147–161, 1989
- Bai J, Ng S. 2002. Determining the number of factors in approximate factor models. Econometrica 70:191–221
- J. Peters, S. Vijayakumar, and S. Schaal. Natural actor-critic. In Proceedings of the Sixteenth European Conference on Machine Learning, pages 280–291, 2005.