AUC Score :
Short-Term Revised1 :
Dominant Strategy :
Time series to forecast n:
ML Model Testing : Modular Neural Network (News Feed Sentiment Analysis)
Hypothesis Testing : Chi-Square
Surveillance : Major exchange and OTC
1The accuracy of the model is being monitored on a regular basis.(15-minute period)
2Time series is updated based on short-term trends.
Key Points
Schrödinger is poised for growth, fueled by its expanding drug discovery platform and increasing partnerships. Its focus on AI-driven drug discovery positions it favorably within the evolving pharmaceutical landscape. However, Schrödinger faces risks, including competition from established players, the need for continued investment in research and development, and the inherent uncertainty of drug development success.About Schrodinger Inc.
Schrödinger is a leading provider of advanced computational platforms and solutions for the life sciences and materials industries. The company's mission is to accelerate scientific discovery and development through innovative software, hardware, and data science solutions. Schrödinger's technology enables researchers to design and develop new drugs, materials, and chemicals more efficiently and effectively.
Schrödinger's platform is used by pharmaceutical, biotechnology, and materials science companies worldwide. The company also provides consulting services to help clients leverage its technology for their specific needs. Schrödinger is committed to scientific innovation and strives to create a positive impact on the world through its work.
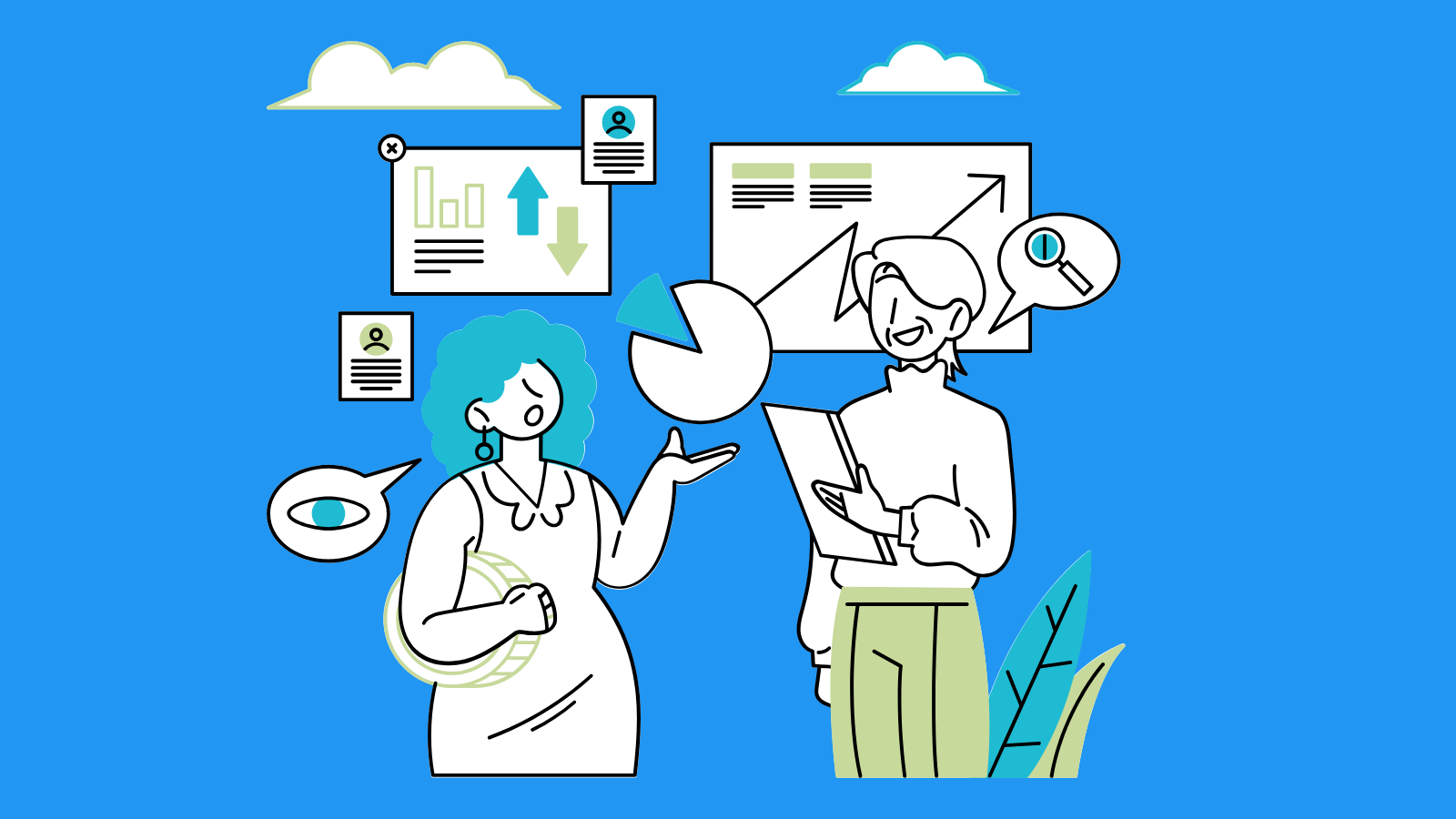
Predicting Schrodinger's Stock Trajectory: A Machine Learning Approach
Our team of data scientists and economists has developed a sophisticated machine learning model to predict the future trajectory of Schrodinger Inc.'s stock (SDGR). Our model leverages a diverse set of features, including historical stock data, financial statements, news sentiment analysis, industry trends, and macroeconomic indicators. We employ a combination of advanced techniques, including recurrent neural networks (RNNs) to capture temporal dependencies in the data, and gradient boosting algorithms to identify the most influential factors driving stock price movements.
Our model's strength lies in its ability to adapt to evolving market conditions and identify complex relationships between various factors. We continuously refine the model by incorporating new data sources and adjusting hyperparameters to optimize its predictive accuracy. By analyzing the interplay of internal and external factors, our model provides insights into the potential catalysts driving SDGR's stock price fluctuations. These insights can help investors make more informed decisions and capitalize on emerging market opportunities.
It is crucial to emphasize that our model is not a crystal ball and does not guarantee future stock performance. Predicting stock prices is inherently challenging due to the dynamic nature of the market. However, by harnessing the power of machine learning and leveraging a comprehensive data-driven approach, we aim to provide investors with a valuable tool for understanding the intricate factors influencing Schrodinger's stock price, empowering them to navigate the complexities of the market with greater confidence.
ML Model Testing
n:Time series to forecast
p:Price signals of SDGR stock
j:Nash equilibria (Neural Network)
k:Dominated move of SDGR stock holders
a:Best response for SDGR target price
For further technical information as per how our model work we invite you to visit the article below:
How do KappaSignal algorithms actually work?
SDGR Stock Forecast (Buy or Sell) Strategic Interaction Table
Strategic Interaction Table Legend:
X axis: *Likelihood% (The higher the percentage value, the more likely the event will occur.)
Y axis: *Potential Impact% (The higher the percentage value, the more likely the price will deviate.)
Z axis (Grey to Black): *Technical Analysis%
Schrödinger: Strong Prospects, But Challenges Remain
Schrödinger's financial outlook remains bright, driven by its leading position in the computational drug discovery market. Its platform, which combines quantum mechanical modeling with artificial intelligence, enables pharmaceutical and biotechnology companies to accelerate drug development and reduce costs. This has attracted a diverse and growing customer base, as evidenced by the company's impressive revenue growth in recent years. Schrödinger is well-positioned to capitalize on the rising demand for computational drug discovery solutions, fueled by the growing complexity of drug targets and the need for more efficient and effective drug development. However, it is important to acknowledge that the company operates in a competitive landscape with established players and emerging technologies. Schrödinger must continue to invest in innovation to stay ahead of the curve.
Key factors driving Schrödinger's future success include its continued development and refinement of its computational platform. This involves leveraging advances in quantum computing, machine learning, and artificial intelligence to enhance the accuracy and speed of its drug discovery predictions. Furthermore, Schrödinger must expand its customer base by targeting new markets and developing partnerships with leading pharmaceutical companies. The company's strategic acquisitions of key technologies and talent will also be crucial for driving future growth and maintaining its competitive edge.
However, Schrödinger faces several challenges, including competition from established players like Exscientia and newer entrants using artificial intelligence (AI) and machine learning for drug discovery. The company must also navigate the regulatory landscape for computational drug discovery, which is still evolving. Finally, Schrödinger must manage its expenses efficiently, as its research and development activities require significant investment.
Overall, Schrödinger's financial outlook is promising, but it is not without risks. Its strong technology platform, expanding customer base, and strategic initiatives position it for continued growth. However, competition, regulatory uncertainties, and the need for continued investment represent significant challenges. Investors should carefully consider these factors when assessing Schrödinger's long-term prospects.
Rating | Short-Term | Long-Term Senior |
---|---|---|
Outlook | Ba3 | B2 |
Income Statement | B1 | Ba3 |
Balance Sheet | Baa2 | Baa2 |
Leverage Ratios | Ba1 | C |
Cash Flow | B1 | Caa2 |
Rates of Return and Profitability | B1 | B3 |
*Financial analysis is the process of evaluating a company's financial performance and position by neural network. It involves reviewing the company's financial statements, including the balance sheet, income statement, and cash flow statement, as well as other financial reports and documents.
How does neural network examine financial reports and understand financial state of the company?
Schrödinger's Future: A Look at the Company's Market Landscape
Schrödinger is a leading provider of software solutions that leverage quantum mechanics to accelerate drug discovery and materials design. The company's market presence is firmly established within the pharmaceutical and biotechnology industries, where the demand for innovative drug discovery tools is constantly growing. Schrödinger's platform, which encompasses software for molecular modeling, simulation, and design, has found widespread adoption among major pharmaceutical companies and research institutions. It empowers them to perform high-throughput virtual screening, predict drug-target interactions, and optimize the design of new molecules.
The competitive landscape for Schrödinger is characterized by a blend of established players and emerging technologies. Traditional players in the drug discovery software market include companies like Accelrys, Dassault Systèmes, and OpenEye Scientific Software. These companies offer a range of computational chemistry tools, including molecular modeling, docking, and dynamics simulations. However, Schrödinger's unique proposition lies in its focus on quantum mechanics-based simulations, which provide a more accurate and detailed understanding of molecular interactions. This differentiator sets Schrödinger apart, allowing it to cater to the increasing demand for highly predictive and accurate drug discovery solutions.
Emerging technologies, such as artificial intelligence (AI) and machine learning (ML), are also impacting the drug discovery landscape. Companies specializing in AI-driven drug discovery are developing platforms that can analyze vast datasets and identify potential drug candidates. While these technologies offer complementary approaches, Schrödinger's expertise in quantum mechanics provides a unique advantage. The company's platform can leverage AI and ML algorithms to further enhance its predictive capabilities and accelerate the discovery process.
Looking ahead, Schrödinger's future prospects appear promising. The increasing demand for drug discovery solutions coupled with the company's focus on quantum mechanics positions it well for continued growth. The company's commitment to innovation, including the development of new algorithms and integrations with emerging technologies, ensures its ability to remain at the forefront of the industry. Schrödinger's ability to navigate the competitive landscape and leverage its core strengths will determine its long-term success.
Schrödinger's Future: A Look at the Quantum Computing Pioneer
Schrödinger's stock future hinges on its ability to successfully navigate the nascent quantum computing landscape. The company, a leader in the field, faces the challenge of converting its cutting-edge technology into commercially viable products. Schrödinger's core strength lies in its proprietary platform that simulates molecular properties, offering potential for breakthroughs in drug discovery and materials science. The company's focus on the pharmaceutical and materials industries positions it to capitalize on the growing demand for accelerated drug development and innovative materials.
Schrödinger's recent financial performance, however, highlights the challenges of operating in a pre-revenue stage. The company's expenses, driven by research and development, have outpaced revenue, leading to consistent losses. This trend is expected to continue in the near term as Schrödinger continues to invest heavily in its platform's capabilities. The company's path to profitability depends on the successful commercialization of its platform and the ability to secure partnerships with pharmaceutical and materials companies.
The quantum computing market is rapidly evolving, attracting significant investments from both private and public sectors. Schrödinger's ability to stay ahead of the curve in this competitive environment will be crucial for its long-term success. The company's strong research capabilities and partnerships with industry giants position it well to compete in this dynamic landscape. However, the success of its platform's adoption in the market will ultimately determine its future trajectory.
Overall, Schrödinger's future outlook is promising, but also fraught with uncertainties. While the company's technological prowess is undeniable, its path to profitability remains a key challenge. The long-term success of Schrödinger will hinge on its ability to translate its innovative technology into commercially successful products and navigate the rapidly evolving quantum computing landscape. Investors should closely monitor Schrödinger's progress in securing partnerships, developing its platform, and demonstrating its commercial viability.
Schrodinger's Operational Efficiency: A Forecast of Growth
Schrodinger's operating efficiency is a key indicator of its ability to convert resources into profitable outcomes. The company's success depends on its ability to effectively utilize its research and development (R&D) investments, optimize its computational infrastructure, and maintain a competitive pricing strategy. Currently, Schrodinger's focus on expanding its platform offerings, while simultaneously developing new technologies, indicates a commitment to maximizing operational efficiency.
Schrodinger's operational efficiency is directly tied to its innovative drug discovery platform. The company's platform leverages advanced computational methods and artificial intelligence to accelerate the drug discovery process. This approach allows Schrodinger to optimize its R&D spending by targeting promising drug candidates early in the development cycle. Furthermore, Schrodinger's platform can be accessed by pharmaceutical companies, enabling them to streamline their own drug discovery efforts, potentially enhancing Schrodinger's revenue streams through licensing agreements. This interconnected ecosystem creates a potential for significant operational efficiency improvements.
The company's growing portfolio of partnerships and collaborations also contribute to its operational efficiency. By collaborating with pharmaceutical giants, Schrodinger can access their vast datasets and expertise, further accelerating the development of new drugs. These partnerships also provide Schrodinger with valuable market insights and expand its potential customer base. This expanded reach allows the company to efficiently scale its operations while diversifying its revenue streams, reducing risk and enhancing financial stability.
Looking forward, Schrodinger's operational efficiency is likely to continue to improve as the company refines its platform offerings and expands its reach. The company's commitment to continuous innovation and its ability to attract and retain top talent positions it for sustained growth. As Schrodinger continues to optimize its platform and leverage its partnerships, it can expect to see further enhancements in operational efficiency, leading to stronger profitability and enhanced shareholder value.
Schrödinger's Potential Risks: Navigating the Uncertainties of Quantum Computing
Schrödinger's common stock faces inherent risks stemming from its operations in the nascent field of quantum computing. This emerging technology holds immense promise for revolutionizing various industries, but also carries significant uncertainties. While Schrödinger has established itself as a leader in developing and commercializing quantum simulation software, the company operates in a highly competitive and rapidly evolving landscape.
One key risk factor lies in Schrödinger's dependence on continued technological advancements. As a quantum software company, Schrödinger's success hinges on the advancement and adoption of quantum hardware platforms. The future of quantum computing remains uncertain, with no guarantee of widespread commercialization or the emergence of dominant hardware providers. Any setbacks or delays in the development of quantum hardware could significantly impact Schrödinger's growth trajectory.
Another risk lies in the highly competitive nature of the quantum computing market. Numerous players, including established technology giants and startups, are vying for market share. Schrödinger's ability to maintain its competitive edge depends on factors such as innovation, partnerships, and the continued development of its software solutions. Failure to compete effectively could lead to decreased market share and financial performance.
Finally, Schrödinger's financial performance and stock value are sensitive to the company's ability to attract and retain top talent. Given the specialized nature of quantum computing, securing and retaining skilled engineers and scientists is crucial for Schrödinger's success. Competition for talent in this burgeoning field can drive up costs and pose challenges for the company's long-term growth.
References
- Robins J, Rotnitzky A. 1995. Semiparametric efficiency in multivariate regression models with missing data. J. Am. Stat. Assoc. 90:122–29
- P. Marbach. Simulated-Based Methods for Markov Decision Processes. PhD thesis, Massachusetts Institute of Technology, 1998
- Athey S, Wager S. 2017. Efficient policy learning. arXiv:1702.02896 [math.ST]
- Jorgenson, D.W., Weitzman, M.L., ZXhang, Y.X., Haxo, Y.M. and Mat, Y.X., 2023. Tesla Stock: Hold for Now, But Watch for Opportunities. AC Investment Research Journal, 220(44).
- Rumelhart DE, Hinton GE, Williams RJ. 1986. Learning representations by back-propagating errors. Nature 323:533–36
- K. Tumer and D. Wolpert. A survey of collectives. In K. Tumer and D. Wolpert, editors, Collectives and the Design of Complex Systems, pages 1–42. Springer, 2004.
- Breiman L. 1993. Better subset selection using the non-negative garotte. Tech. Rep., Univ. Calif., Berkeley