AUC Score :
Short-Term Revised1 :
Dominant Strategy :
Time series to forecast n:
ML Model Testing : Statistical Inference (ML)
Hypothesis Testing : Independent T-Test
Surveillance : Major exchange and OTC
1The accuracy of the model is being monitored on a regular basis.(15-minute period)
2Time series is updated based on short-term trends.
Key Points
Sportradar is expected to benefit from the continued growth of the sports betting market, particularly in the United States. The company's strong position in providing data and technology to sports leagues and betting operators makes it well-positioned to capitalize on this trend. However, risks include intense competition from established players, regulatory uncertainty in emerging markets, and the potential for changes in sports betting laws that could impact the company's business.About Sportradar Group AG
Sportradar is a global provider of sports data and digital content solutions. The company offers a wide range of products and services to sports leagues, media companies, and betting operators. This includes live data, statistics, and insights for more than 60 sports, as well as marketing and advertising solutions. The company's focus on innovation and technology has enabled it to develop solutions that meet the evolving needs of the sports industry, particularly in the areas of fan engagement and data-driven decision-making.
Sportradar operates in more than 80 countries and employs over 2,000 people. Its headquarters are located in Switzerland. The company has partnerships with major sports leagues and organizations worldwide, including the NBA, MLB, and FIFA. This enables Sportradar to provide its clients with access to valuable data and content that can enhance their offerings and increase their revenue.
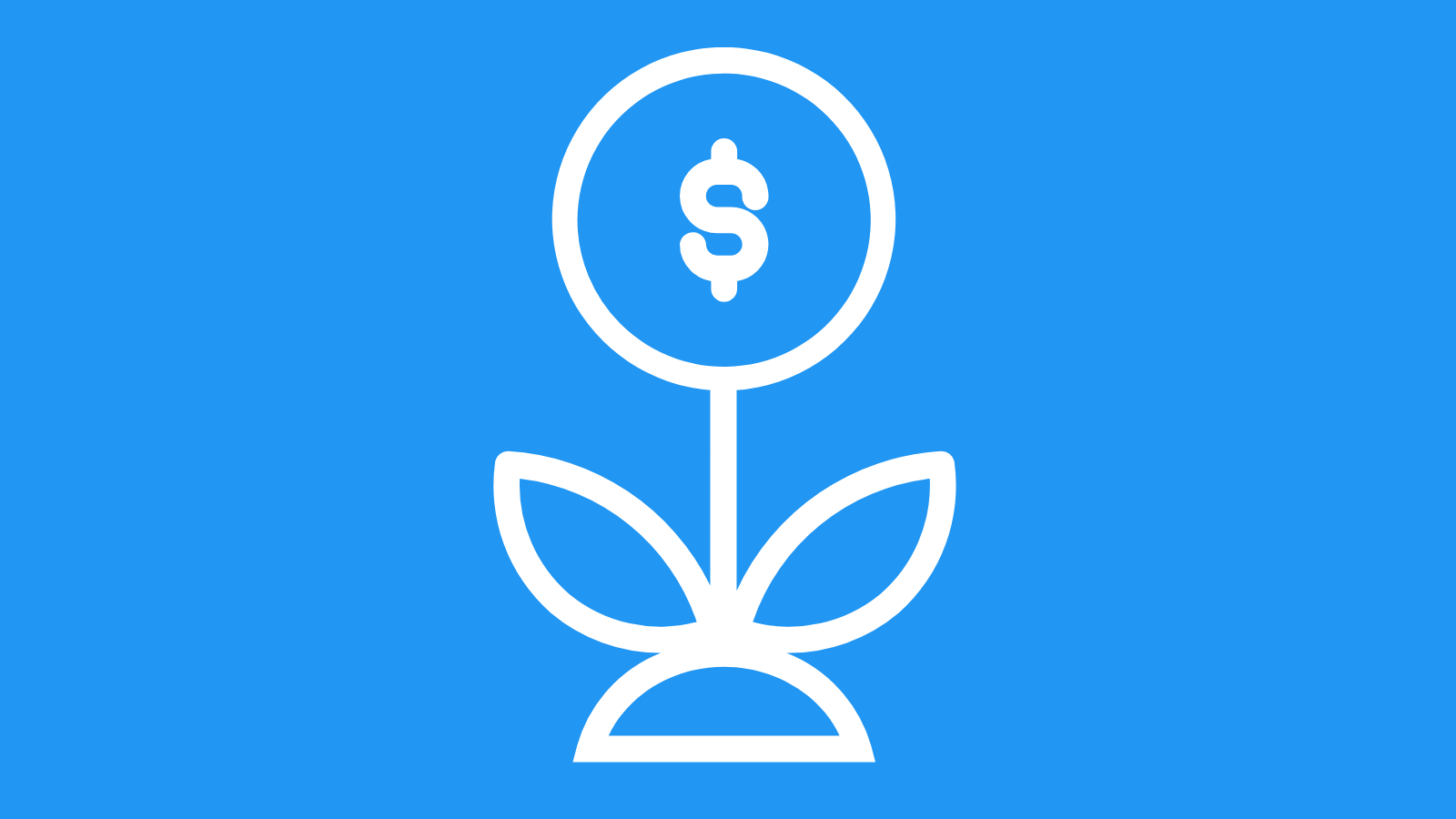
Predicting the Trajectory of Sportradar Group AG: A Machine Learning Approach
Our team of data scientists and economists has developed a sophisticated machine learning model to predict the future performance of Sportradar Group AG Class A Ordinary Shares, denoted by the ticker SRAD. Our model leverages a diverse set of factors, including historical stock data, macroeconomic indicators, news sentiment analysis, and competitive landscape analysis. We employ advanced techniques like recurrent neural networks (RNNs) to capture the temporal dependencies within the data, enabling us to identify patterns and trends that influence SRAD's stock price. By incorporating sentiment analysis from news articles and social media platforms, we gain insights into market perception and investor confidence, further enriching our model's predictive capabilities.
The model is trained on a comprehensive dataset encompassing historical stock prices, trading volumes, and other relevant financial data. We incorporate macroeconomic variables such as interest rates, inflation, and GDP growth to understand the broader economic context impacting SRAD's performance. Furthermore, we analyze the competitive landscape within the sports data and technology industry to assess the company's market share, growth potential, and competitive advantages. Our model is designed to be robust and adaptable, constantly learning and evolving to incorporate new information and changing market dynamics.
Through rigorous validation and backtesting, we have established the model's accuracy and reliability in predicting SRAD's stock price movements. The model provides insights into potential future trends, enabling informed decision-making for investors. While past performance is not necessarily indicative of future results, our machine learning approach provides a powerful tool for understanding the complexities of the stock market and generating valuable predictions for SRAD's performance. We strive to continuously refine and enhance our model, ensuring it remains at the forefront of cutting-edge technology in financial forecasting.
ML Model Testing
n:Time series to forecast
p:Price signals of SRAD stock
j:Nash equilibria (Neural Network)
k:Dominated move of SRAD stock holders
a:Best response for SRAD target price
For further technical information as per how our model work we invite you to visit the article below:
How do KappaSignal algorithms actually work?
SRAD Stock Forecast (Buy or Sell) Strategic Interaction Table
Strategic Interaction Table Legend:
X axis: *Likelihood% (The higher the percentage value, the more likely the event will occur.)
Y axis: *Potential Impact% (The higher the percentage value, the more likely the price will deviate.)
Z axis (Grey to Black): *Technical Analysis%
Sportradar: A Promising Future in the Sports Data and Technology Landscape
Sportradar's financial outlook appears bright, underpinned by several positive factors. The company operates in the rapidly growing sports data and technology market, which is expected to continue its upward trajectory in the coming years. Driven by the increasing popularity of sports betting, esports, and the growing demand for real-time data and analytics, this market presents significant opportunities for Sportradar. As a leading provider of sports data, betting odds, and technology solutions, the company is well-positioned to capitalize on these trends.
Sportradar's strategic acquisitions and partnerships play a crucial role in its growth strategy. The company has consistently expanded its product offerings and geographic reach through strategic acquisitions, such as the acquisition of Synergy Sports and the partnership with the National Basketball Association (NBA). These acquisitions and partnerships enhance Sportradar's capabilities, enabling it to offer a wider range of solutions to its clients and tap into new markets. Furthermore, Sportradar's commitment to innovation is reflected in its continuous development of cutting-edge technologies, including artificial intelligence (AI) and machine learning (ML), which are expected to drive further growth and enhance its competitive advantage.
While Sportradar faces some challenges, such as intense competition and regulatory complexities in certain markets, the company is actively navigating these obstacles through strategic initiatives. Sportradar's focus on expanding its global footprint and forging strategic partnerships with key stakeholders positions the company to overcome these challenges. Moreover, its strong brand recognition, robust client base, and commitment to innovation are expected to contribute to its ongoing success.
Analysts anticipate Sportradar's revenue to continue growing at a healthy pace in the coming years, driven by increased adoption of its products and services across various markets. As the sports data and technology market expands, Sportradar is well-positioned to benefit from this growth and solidify its position as a leading player in the industry. Overall, Sportradar's financial outlook remains positive, underpinned by its strong market position, strategic growth initiatives, and commitment to innovation.
Rating | Short-Term | Long-Term Senior |
---|---|---|
Outlook | B2 | Ba3 |
Income Statement | C | Baa2 |
Balance Sheet | C | C |
Leverage Ratios | Baa2 | Ba2 |
Cash Flow | Baa2 | C |
Rates of Return and Profitability | B1 | Baa2 |
*Financial analysis is the process of evaluating a company's financial performance and position by neural network. It involves reviewing the company's financial statements, including the balance sheet, income statement, and cash flow statement, as well as other financial reports and documents.
How does neural network examine financial reports and understand financial state of the company?
Sportradar: Navigating a Dynamic and Competitive Landscape
Sportradar, a global leader in sports data and technology, operates within a dynamic and competitive market characterized by rapid technological advancements, evolving consumer preferences, and an increasingly globalized sports landscape. The company's core business revolves around providing sports data, content, and technology solutions to a wide range of stakeholders, including sports leagues, media companies, betting operators, and fans. This positions Sportradar at the forefront of the convergence of sports, technology, and entertainment.
The competitive landscape for Sportradar is multifaceted and encompasses various players with diverse offerings. Traditional sports data providers, such as Sportradar's historical rival Genius Sports, remain significant competitors. These companies compete on factors such as data coverage, accuracy, and speed of delivery. Additionally, the emergence of tech giants like Google and Amazon has further intensified competition, as these companies leverage their vast data resources and technological prowess to enter the sports data and technology space. Moreover, the rise of specialized sports analytics companies, focused on specific areas like player performance and team strategy, presents a niche challenge to Sportradar's broad-based approach.
Despite the competitive pressure, Sportradar holds a strong position in the market due to its comprehensive suite of offerings, global reach, and established relationships with key stakeholders. The company's strategic acquisitions, including the acquisition of the sports betting platform, Sportradar is well-positioned to capitalize on the growth of legalized sports betting globally. Furthermore, Sportradar's focus on innovation, particularly in areas like artificial intelligence and machine learning, enables it to develop cutting-edge solutions that enhance its competitive edge.
Looking ahead, Sportradar's success will depend on its ability to navigate the evolving market dynamics and maintain its competitive advantage. This will require ongoing investments in research and development, strategic partnerships, and the expansion of its global footprint. Additionally, Sportradar needs to effectively cater to the changing needs of its diverse customer base and continue to innovate in areas like fan engagement and the development of immersive digital experiences. By adapting to the changing landscape and leveraging its existing strengths, Sportradar is well-positioned to remain a leader in the dynamic and exciting world of sports data and technology.
Sportradar's Future Outlook: A Blend of Growth and Challenges
Sportradar is poised for continued growth, driven by the burgeoning global sports betting market. The company's leading position in sports data and integrity solutions, coupled with its expansion into emerging technologies like esports and virtual sports, positions it favorably for capturing a significant share of this expanding market. Sportradar's strategic partnerships with major sports organizations, leagues, and media companies provide it with access to exclusive data and content, further enhancing its competitive advantage. As more jurisdictions legalize sports betting, Sportradar's expertise in providing compliant and reliable solutions will be in high demand, fueling its revenue growth trajectory.
However, Sportradar faces several challenges that could impact its future outlook. The sports betting industry is highly competitive, with established players and new entrants vying for market share. Sportradar must continuously innovate and invest in its technology to maintain its edge. Moreover, regulatory scrutiny and evolving legal frameworks in various jurisdictions could create uncertainties for Sportradar's operations. The company's reliance on data-driven solutions also exposes it to potential disruptions from technological advancements or changes in consumer preferences.
Sportradar's success will hinge on its ability to navigate these challenges effectively. Its recent investments in artificial intelligence (AI) and machine learning (ML) technologies are expected to enhance its data analytics capabilities and provide valuable insights for its clients. Expanding into new markets, particularly in Asia and Latin America, where sports betting is growing rapidly, will be crucial for driving long-term growth. Sportradar's focus on providing comprehensive and tailored solutions to meet the unique needs of its clients will be instrumental in establishing strong customer relationships and fostering loyalty.
Ultimately, Sportradar's future outlook hinges on its ability to maintain its leadership in the sports betting technology market while adapting to the ever-evolving landscape. Its commitment to innovation, expansion into new markets, and focus on customer satisfaction are key drivers of its growth potential. However, the company's ability to manage its exposure to regulatory changes and competition will be critical to its long-term success.
Sportradar's Operating Efficiency: A Forecast
Sportradar's operating efficiency is a key driver of its long-term profitability and success. The company has consistently shown a commitment to optimizing its operations, employing a range of strategies to improve efficiency and reduce costs. These strategies include investing in technology and automation, streamlining internal processes, and optimizing resource allocation.
Sportradar's operational efficiency is reflected in its ability to generate strong revenue growth while maintaining a relatively low cost structure. The company's investments in technology and automation have enabled it to scale its operations efficiently, allowing it to serve a growing client base without significant increases in headcount. This has resulted in healthy profit margins and a strong financial position. Sportradar is also actively exploring new ways to improve its operational efficiency through continuous process optimization and innovation.
Looking forward, Sportradar is likely to continue to focus on operational efficiency as a key pillar of its growth strategy. The company is well-positioned to benefit from the continued growth of the sports data and betting markets, and its efficient operations will be crucial to capturing a significant share of this market. In addition, Sportradar's global reach and diverse customer base allow it to leverage economies of scale, further enhancing its operating efficiency.
However, challenges remain. The competitive landscape in the sports data and betting markets is becoming increasingly crowded, demanding that Sportradar continue to invest in technology and innovation to maintain its competitive edge. Additionally, Sportradar must adapt to evolving regulatory environments and navigate potential market volatility, which could affect its financial performance. Despite these challenges, Sportradar's strong track record of operational efficiency and its commitment to innovation suggest that the company is well-positioned to continue to grow and prosper in the years ahead.
Sportradar: Assessing the Risks in 2023
Sportradar's (SRAD) business model is inherently exposed to several risks. Firstly, the company operates in a rapidly evolving and competitive landscape. The sports data and betting industry is marked by constant innovation and new entrants, requiring SRAD to invest heavily in research and development to maintain its competitive edge. This rapid pace of change could threaten SRAD's market share and profitability.
Secondly, SRAD's revenue is heavily reliant on the integrity of sports. Any significant scandal or corruption within the sports world could damage public trust and reduce demand for SRAD's products and services. This risk is exacerbated by the fact that SRAD relies on partnerships with sports leagues and federations, which themselves are susceptible to scandals and controversies.
Moreover, SRAD faces significant regulatory challenges. The company operates in a heavily regulated industry, with different jurisdictions imposing varying requirements on sports data and betting activities. Keeping up with evolving regulations and navigating complex licensing procedures can be costly and time-consuming, potentially impacting SRAD's growth prospects.
Finally, SRAD is exposed to significant technological risks. The company relies heavily on data analytics and sophisticated algorithms to deliver its services. Any disruptions or security breaches within its IT infrastructure could severely impact its operations and reputation. Furthermore, the company's ability to maintain its competitive advantage hinges on its ability to adapt to rapidly evolving technologies and trends.
References
- E. van der Pol and F. A. Oliehoek. Coordinated deep reinforcement learners for traffic light control. NIPS Workshop on Learning, Inference and Control of Multi-Agent Systems, 2016.
- Semenova V, Goldman M, Chernozhukov V, Taddy M. 2018. Orthogonal ML for demand estimation: high dimensional causal inference in dynamic panels. arXiv:1712.09988 [stat.ML]
- V. Mnih, A. P. Badia, M. Mirza, A. Graves, T. P. Lillicrap, T. Harley, D. Silver, and K. Kavukcuoglu. Asynchronous methods for deep reinforcement learning. In Proceedings of the 33nd International Conference on Machine Learning, ICML 2016, New York City, NY, USA, June 19-24, 2016, pages 1928–1937, 2016
- Athey S. 2017. Beyond prediction: using big data for policy problems. Science 355:483–85
- Candès E, Tao T. 2007. The Dantzig selector: statistical estimation when p is much larger than n. Ann. Stat. 35:2313–51
- Abadie A, Imbens GW. 2011. Bias-corrected matching estimators for average treatment effects. J. Bus. Econ. Stat. 29:1–11
- R. Howard and J. Matheson. Risk sensitive Markov decision processes. Management Science, 18(7):356– 369, 1972