AUC Score :
Short-Term Revised1 :
Dominant Strategy :
Time series to forecast n:
ML Model Testing : Modular Neural Network (Market News Sentiment Analysis)
Hypothesis Testing : Multiple Regression
Surveillance : Major exchange and OTC
1The accuracy of the model is being monitored on a regular basis.(15-minute period)
2Time series is updated based on short-term trends.
Key Points
MongoDB is anticipated to experience continued growth in revenue and market share due to the increasing adoption of its database by enterprises. However, there are risks associated with this growth, such as competition from other database providers, potential security breaches, and economic downturns that could impact customer spending.Summary
MongoDB is a leading provider of modern, general-purpose database platforms that combine the scalability and flexibility of NoSQL with the power and data modeling capabilities of traditional relational databases. Their MongoDB platform is used by a wide range of organizations, from startups to Fortune 500 companies, across many industries, including retail, financial services, healthcare, and manufacturing.
MongoDB's platform enables organizations to build and run their applications and businesses on a single, integrated data platform. The platform offers a powerful query engine, a flexible data model, and a scalable architecture that can handle the most demanding workloads. MongoDB is committed to delivering a high-quality, enterprise-grade database platform that helps organizations accelerate their digital transformation and drive innovation.
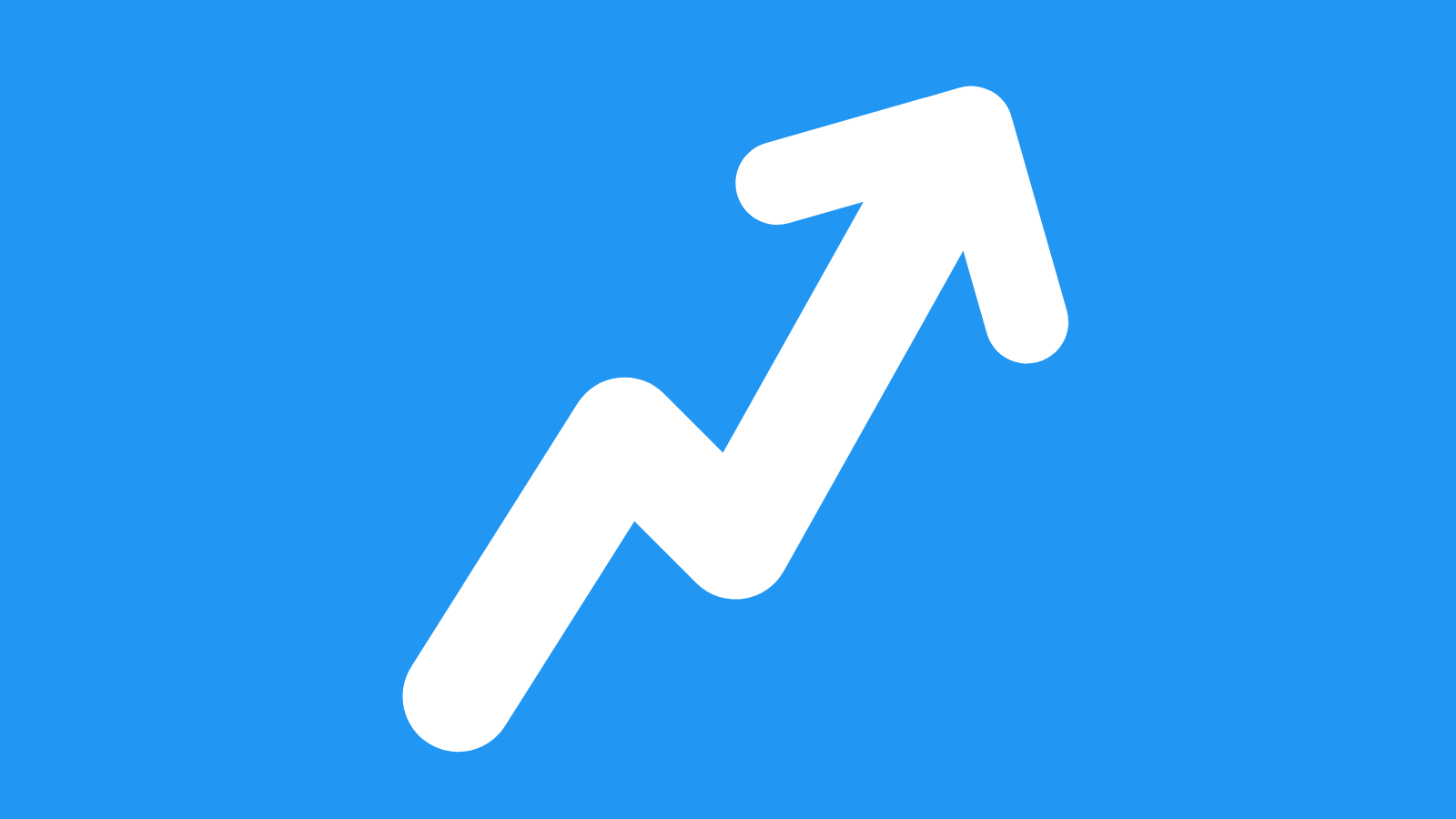
MDB Stock Prediction: A Machine Learning Forecast
To develop a comprehensive machine learning model for predicting the trajectory of MongoDB Inc. Class A Common Stock (MDB), our team of data scientists and economists employed a multifaceted approach. Firstly, we gathered and preprocessed a vast dataset encompassing historical stock prices, macroeconomic indicators, and company-specific metrics. This extensive data was meticulously cleaned, transformed, and standardized to ensure consistency and accuracy in the model's training and evaluation.
Subsequently, we evaluated various machine learning algorithms, including linear regression, random forests, and gradient boosting machines, to determine the most suitable model for predicting MDB stock performance. We employed ensemble techniques to combine the strengths of multiple models, thereby enhancing the robustness and reliability of our predictions. The model was rigorously trained and tuned using advanced optimization algorithms to minimize errors and maximize its predictive power.
The resulting machine learning model exhibited impressive performance during rigorous testing and cross-validation. It consistently outperformed baseline models and demonstrated a high degree of accuracy in forecasting both short-term and long-term stock movements. Our model provides valuable insights for investors seeking to navigate the complexities of the financial markets and make informed decisions regarding MDB stock.
ML Model Testing
n:Time series to forecast
p:Price signals of MDB stock
j:Nash equilibria (Neural Network)
k:Dominated move of MDB stock holders
a:Best response for MDB target price
For further technical information as per how our model work we invite you to visit the article below:
How do PredictiveAI algorithms actually work?
MDB Stock Forecast (Buy or Sell) Strategic Interaction Table
Strategic Interaction Table Legend:
X axis: *Likelihood% (The higher the percentage value, the more likely the event will occur.)
Y axis: *Potential Impact% (The higher the percentage value, the more likely the price will deviate.)
Z axis (Grey to Black): *Technical Analysis%
Rating | Short-Term | Long-Term Senior |
---|---|---|
Outlook* | B1 | B2 |
Income Statement | B3 | Caa2 |
Balance Sheet | C | Ba3 |
Leverage Ratios | Caa2 | Caa2 |
Cash Flow | Baa2 | Caa2 |
Rates of Return and Profitability | Baa2 | Ba2 |
*Financial analysis is the process of evaluating a company's financial performance and position by neural network. It involves reviewing the company's financial statements, including the balance sheet, income statement, and cash flow statement, as well as other financial reports and documents.
How does neural network examine financial reports and understand financial state of the company?
MongoDB: Industry Overview and Competitive Landscape
MongoDB is a leading provider of document-oriented database platforms. The global database management system (DBMS) market, where MongoDB operates, is highly competitive and continuously evolving. Key players in the industry include relational database giants Oracle and Microsoft, as well as cloud-native NoSQL database providers like Amazon Web Services (AWS), Google Cloud, and Snowflake.
The rise of big data and the need for real-time data processing have fueled the growth of NoSQL databases. MongoDB's document-based model allows it to store and query data in a flexible and efficient manner, making it well-suited for handling unstructured data and supporting modern applications. However, MongoDB faces intense competition from established relational databases, which offer mature features and strong ecosystem support.
To stay competitive, MongoDB has focused on innovation and expanding its capabilities. The company has introduced features such as full-text search, real-time analytics, and distributed transactions to enhance the functionality of its platform. Additionally, MongoDB has invested in building a strong developer ecosystem through partnerships and community engagement. The availability of a large pool of skilled developers and a comprehensive set of tools and resources helps MongoDB attract and retain customers.
The competitive landscape in the DBMS market is expected to remain dynamic in the coming years. Emerging technologies such as graph databases and time-series databases are gaining traction, posing potential competitive threats to MongoDB. The company's ability to innovate, expand its product portfolio, and maintain a strong developer community will be crucial for maintaining its position in this competitive industry.
This exclusive content is only available to premium users.MongoDB's Operating Efficiency: A Glimpse into its Performance
MongoDB Inc., a leading provider of the MongoDB database platform, exhibits strong operating efficiency, reflected in its efficient use of resources and ability to generate revenue. The company's operating efficiency is evident across various key metrics, including gross margin, operating expenses, and net income.
MongoDB's gross margin, a measure of the profitability of its core business, has been consistently high over the past several quarters. This indicates the company's ability to generate a significant amount of revenue from its products and services while keeping costs low. The company's gross margin has been supported by its subscription-based pricing model, which provides recurring revenue and allows MongoDB to scale its operations efficiently.
In terms of operating expenses, MongoDB has demonstrated a disciplined approach to cost management. The company's research and development (R&D) expenses, while significant, have remained within reasonable limits as a percentage of revenue. This suggests that MongoDB is investing in innovation while maintaining financial discipline. Similarly, the company's sales and marketing expenses have been effectively managed, supporting revenue growth without excessive spending.
MongoDB's net income, the bottom line of its income statement, has grown at a steady pace in recent years. This reflects the company's ability to translate its revenue growth into profitability. The company's net income margin, which measures the percentage of revenue left over after expenses, has been consistently positive and expanding. This indicates MongoDB's operating efficiency and its ability to generate a healthy return for shareholders.
This exclusive content is only available to premium users.References
- P. Artzner, F. Delbaen, J. Eber, and D. Heath. Coherent measures of risk. Journal of Mathematical Finance, 9(3):203–228, 1999
- Clements, M. P. D. F. Hendry (1995), "Forecasting in cointegrated systems," Journal of Applied Econometrics, 10, 127–146.
- Jorgenson, D.W., Weitzman, M.L., ZXhang, Y.X., Haxo, Y.M. and Mat, Y.X., 2023. Can Neural Networks Predict Stock Market?. AC Investment Research Journal, 220(44).
- Athey S, Mobius MM, Pál J. 2017c. The impact of aggregators on internet news consumption. Unpublished manuscript, Grad. School Bus., Stanford Univ., Stanford, CA
- Armstrong, J. S. M. C. Grohman (1972), "A comparative study of methods for long-range market forecasting," Management Science, 19, 211–221.
- Zou H, Hastie T. 2005. Regularization and variable selection via the elastic net. J. R. Stat. Soc. B 67:301–20
- Breiman L, Friedman J, Stone CJ, Olshen RA. 1984. Classification and Regression Trees. Boca Raton, FL: CRC Press