AUC Score :
Short-Term Revised1 :
Dominant Strategy : Buy
Time series to forecast n:
Methodology : Multi-Instance Learning (ML)
Hypothesis Testing : ElasticNet Regression
Surveillance : Major exchange and OTC
1The accuracy of the model is being monitored on a regular basis.(15-minute period)
2Time series is updated based on short-term trends.
Abstract
NEWMARK PROPERTY REIT prediction model is evaluated with Multi-Instance Learning (ML) and ElasticNet Regression1,2,3,4 and it is concluded that the NPR stock is predictable in the short/long term. Multi-instance learning (MIL) is a machine learning (ML) problem where a dataset consists of multiple instances, and each instance is associated with a single label. The goal of MIL is to learn a model that can predict the label of a new instance based on the labels of the instances that it is similar to. MIL is a challenging problem because the instances in a dataset are not labeled individually. This means that the model cannot simply learn a mapping from the features of an instance to its label. Instead, the model must learn a way to combine the features of multiple instances to predict the label of a new instance. According to price forecasts for 16 Weeks period, the dominant strategy among neural network is: Buy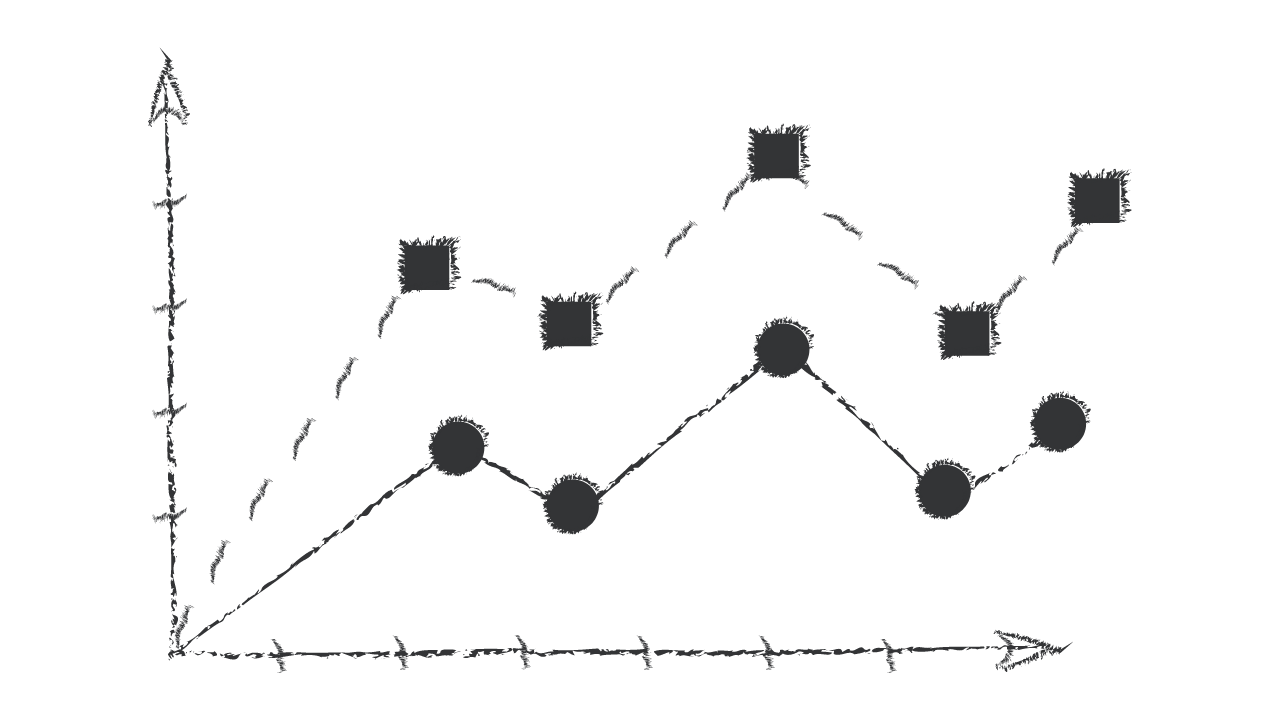
Key Points
- What are the most successful trading algorithms?
- Nash Equilibria
- What is statistical models in machine learning?
NPR Target Price Prediction Modeling Methodology
We consider NEWMARK PROPERTY REIT Decision Process with Multi-Instance Learning (ML) where A is the set of discrete actions of NPR stock holders, F is the set of discrete states, P : S × F × S → R is the transition probability distribution, R : S × F → R is the reaction function, and γ ∈ [0, 1] is a move factor for expectation.1,2,3,4
F(ElasticNet Regression)5,6,7= X R(Multi-Instance Learning (ML)) X S(n):→ 16 Weeks
n:Time series to forecast
p:Price signals of NPR stock
j:Nash equilibria (Neural Network)
k:Dominated move
a:Best response for target price
Multi-Instance Learning (ML)
Multi-instance learning (MIL) is a machine learning (ML) problem where a dataset consists of multiple instances, and each instance is associated with a single label. The goal of MIL is to learn a model that can predict the label of a new instance based on the labels of the instances that it is similar to. MIL is a challenging problem because the instances in a dataset are not labeled individually. This means that the model cannot simply learn a mapping from the features of an instance to its label. Instead, the model must learn a way to combine the features of multiple instances to predict the label of a new instance.ElasticNet Regression
Elastic net regression is a type of regression analysis that combines the benefits of ridge regression and lasso regression. It is a regularized regression method that adds a penalty to the least squares objective function in order to reduce the variance of the estimates, induce sparsity in the model, and reduce overfitting. This is done by adding a term to the objective function that is proportional to the sum of the squares of the coefficients and the sum of the absolute values of the coefficients. The penalty terms are controlled by two parameters, called the ridge constant and the lasso constant. Elastic net regression can be used to address the problems of multicollinearity, overfitting, and sensitivity to outliers. It is a more flexible method than ridge regression or lasso regression, and it can often achieve better results.
For further technical information as per how our model work we invite you to visit the article below:
How do AC Investment Research machine learning (predictive) algorithms actually work?
NPR Stock Forecast (Buy or Sell)
Sample Set: Neural NetworkStock/Index: NPR NEWMARK PROPERTY REIT
Time series to forecast: 16 Weeks
According to price forecasts, the dominant strategy among neural network is: Buy
Strategic Interaction Table Legend:
X axis: *Likelihood% (The higher the percentage value, the more likely the event will occur.)
Y axis: *Potential Impact% (The higher the percentage value, the more likely the price will deviate.)
Z axis (Grey to Black): *Technical Analysis%
Financial Data Adjustments for Multi-Instance Learning (ML) based NPR Stock Prediction Model
- Amounts presented in other comprehensive income shall not be subsequently transferred to profit or loss. However, the entity may transfer the cumulative gain or loss within equity.
- An entity can also designate only changes in the cash flows or fair value of a hedged item above or below a specified price or other variable (a 'one-sided risk'). The intrinsic value of a purchased option hedging instrument (assuming that it has the same principal terms as the designated risk), but not its time value, reflects a one-sided risk in a hedged item. For example, an entity can designate the variability of future cash flow outcomes resulting from a price increase of a forecast commodity purchase. In such a situation, the entity designates only cash flow losses that result from an increase in the price above the specified level. The hedged risk does not include the time value of a purchased option, because the time value is not a component of the forecast transaction that affects profit or loss.
- In addition to those hedging relationships specified in paragraph 6.9.1, an entity shall apply the requirements in paragraphs 6.9.11 and 6.9.12 to new hedging relationships in which an alternative benchmark rate is designated as a non-contractually specified risk component (see paragraphs 6.3.7(a) and B6.3.8) when, because of interest rate benchmark reform, that risk component is not separately identifiable at the date it is designated.
- An entity's documentation of the hedging relationship includes how it will assess the hedge effectiveness requirements, including the method or methods used. The documentation of the hedging relationship shall be updated for any changes to the methods (see paragraph B6.4.17).
*International Financial Reporting Standards (IFRS) adjustment process involves reviewing the company's financial statements and identifying any differences between the company's current accounting practices and the requirements of the IFRS. If there are any such differences, neural network makes adjustments to financial statements to bring them into compliance with the IFRS.
NPR NEWMARK PROPERTY REIT Financial Analysis*
Rating | Short-Term | Long-Term Senior |
---|---|---|
Outlook* | B3 | B3 |
Income Statement | Caa2 | B2 |
Balance Sheet | B3 | Caa2 |
Leverage Ratios | Ba1 | C |
Cash Flow | C | C |
Rates of Return and Profitability | Caa2 | Ba2 |
*Financial analysis is the process of evaluating a company's financial performance and position by neural network. It involves reviewing the company's financial statements, including the balance sheet, income statement, and cash flow statement, as well as other financial reports and documents.
How does neural network examine financial reports and understand financial state of the company?
Conclusions
NEWMARK PROPERTY REIT is assigned short-term B3 & long-term B3 estimated rating. NEWMARK PROPERTY REIT prediction model is evaluated with Multi-Instance Learning (ML) and ElasticNet Regression1,2,3,4 and it is concluded that the NPR stock is predictable in the short/long term. According to price forecasts for 16 Weeks period, the dominant strategy among neural network is: Buy
Prediction Confidence Score
References
- Brailsford, T.J. R.W. Faff (1996), "An evaluation of volatility forecasting techniques," Journal of Banking Finance, 20, 419–438.
- Dudik M, Langford J, Li L. 2011. Doubly robust policy evaluation and learning. In Proceedings of the 28th International Conference on Machine Learning, pp. 1097–104. La Jolla, CA: Int. Mach. Learn. Soc.
- Matzkin RL. 2007. Nonparametric identification. In Handbook of Econometrics, Vol. 6B, ed. J Heckman, E Learner, pp. 5307–68. Amsterdam: Elsevier
- Z. Wang, T. Schaul, M. Hessel, H. van Hasselt, M. Lanctot, and N. de Freitas. Dueling network architectures for deep reinforcement learning. In Proceedings of the International Conference on Machine Learning (ICML), pages 1995–2003, 2016.
- Jiang N, Li L. 2016. Doubly robust off-policy value evaluation for reinforcement learning. In Proceedings of the 33rd International Conference on Machine Learning, pp. 652–61. La Jolla, CA: Int. Mach. Learn. Soc.
- Barrett, C. B. (1997), "Heteroscedastic price forecasting for food security management in developing countries," Oxford Development Studies, 25, 225–236.
- D. Bertsekas and J. Tsitsiklis. Neuro-dynamic programming. Athena Scientific, 1996.
Frequently Asked Questions
Q: What is the prediction methodology for NPR stock?A: NPR stock prediction methodology: We evaluate the prediction models Multi-Instance Learning (ML) and ElasticNet Regression
Q: Is NPR stock a buy or sell?
A: The dominant strategy among neural network is to Buy NPR Stock.
Q: Is NEWMARK PROPERTY REIT stock a good investment?
A: The consensus rating for NEWMARK PROPERTY REIT is Buy and is assigned short-term B3 & long-term B3 estimated rating.
Q: What is the consensus rating of NPR stock?
A: The consensus rating for NPR is Buy.
Q: What is the prediction period for NPR stock?
A: The prediction period for NPR is 16 Weeks