Dominant Strategy : Strong Sell
Time series to forecast n: 13 Jun 2023 for 1 Year
Methodology : Transductive Learning (ML)
Abstract
Rush Street Interactive Inc. Class A Common Stock prediction model is evaluated with Transductive Learning (ML) and Chi-Square1,2,3,4 and it is concluded that the RSI stock is predictable in the short/long term. Transductive learning is a supervised machine learning (ML) method in which the model is trained on both labeled and unlabeled data. The goal of transductive learning is to predict the labels of the unlabeled data. Transductive learning is a hybrid of inductive and semi-supervised learning. Inductive learning algorithms are trained on labeled data only, while semi-supervised learning algorithms are trained on a combination of labeled and unlabeled data. Transductive learning algorithms can achieve better performance than inductive learning algorithms on tasks where there is a small amount of labeled data. This is because transductive learning algorithms can use the unlabeled data to help them learn the relationships between the features and the labels. According to price forecasts for 1 Year period, the dominant strategy among neural network is: Strong Sell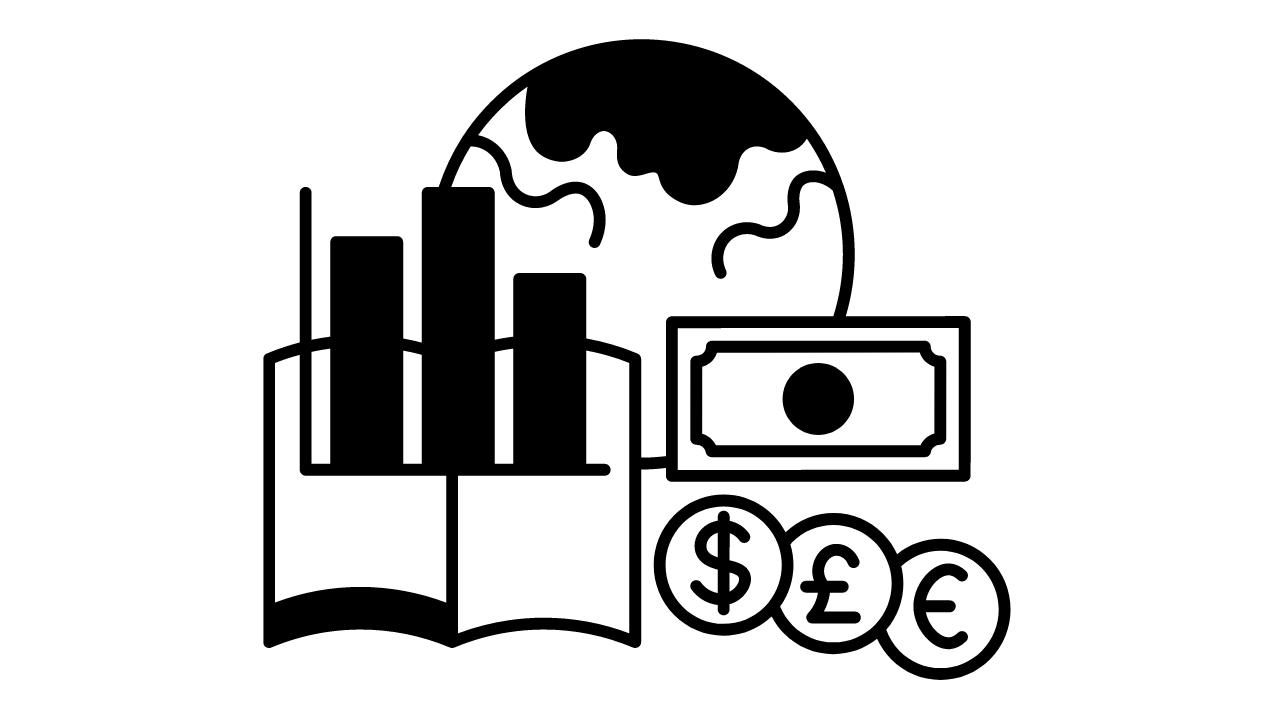
Key Points
- What are the most successful trading algorithms?
- Can statistics predict the future?
- Technical Analysis with Algorithmic Trading
RSI Target Price Prediction Modeling Methodology
We consider Rush Street Interactive Inc. Class A Common Stock Decision Process with Transductive Learning (ML) where A is the set of discrete actions of RSI stock holders, F is the set of discrete states, P : S × F × S → R is the transition probability distribution, R : S × F → R is the reaction function, and γ ∈ [0, 1] is a move factor for expectation.1,2,3,4
F(Chi-Square)5,6,7= X R(Transductive Learning (ML)) X S(n):→ 1 Year
n:Time series to forecast
p:Price signals of RSI stock
j:Nash equilibria (Neural Network)
k:Dominated move
a:Best response for target price
Transductive Learning (ML)
Transductive learning is a supervised machine learning (ML) method in which the model is trained on both labeled and unlabeled data. The goal of transductive learning is to predict the labels of the unlabeled data. Transductive learning is a hybrid of inductive and semi-supervised learning. Inductive learning algorithms are trained on labeled data only, while semi-supervised learning algorithms are trained on a combination of labeled and unlabeled data. Transductive learning algorithms can achieve better performance than inductive learning algorithms on tasks where there is a small amount of labeled data. This is because transductive learning algorithms can use the unlabeled data to help them learn the relationships between the features and the labels.Chi-Square
A chi-squared test is a statistical hypothesis test that assesses whether observed frequencies in a sample differ significantly from expected frequencies. It is one of the most widely used statistical tests in the social sciences and in many areas of observational research. The chi-squared test is a non-parametric test, meaning that it does not assume that the data is normally distributed. This makes it a versatile tool that can be used to analyze a wide variety of data. There are two main types of chi-squared tests: the chi-squared goodness of fit test and the chi-squared test of independence.
For further technical information as per how our model work we invite you to visit the article below:
How do AC Investment Research machine learning (predictive) algorithms actually work?
RSI Stock Forecast (Buy or Sell) for 1 Year
Sample Set: Neural NetworkStock/Index: RSI Rush Street Interactive Inc. Class A Common Stock
Time series to forecast n: 13 Jun 2023 for 1 Year
According to price forecasts for 1 Year period, the dominant strategy among neural network is: Strong Sell
X axis: *Likelihood% (The higher the percentage value, the more likely the event will occur.)
Y axis: *Potential Impact% (The higher the percentage value, the more likely the price will deviate.)
Z axis (Grey to Black): *Technical Analysis%
IFRS Reconciliation Adjustments for Rush Street Interactive Inc. Class A Common Stock
- An entity need not undertake an exhaustive search for information but shall consider all reasonable and supportable information that is available without undue cost or effort and that is relevant to the estimate of expected credit losses, including the effect of expected prepayments. The information used shall include factors that are specific to the borrower, general economic conditions and an assessment of both the current as well as the forecast direction of conditions at the reporting date. An entity may use various sources of data, that may be both internal (entity-specific) and external. Possible data sources include internal historical credit loss experience, internal ratings, credit loss experience of other entities and external ratings, reports and statistics. Entities that have no, or insufficient, sources of entityspecific data may use peer group experience for the comparable financial instrument (or groups of financial instruments).
- An entity shall apply Annual Improvements to IFRS Standards 2018–2020 to financial liabilities that are modified or exchanged on or after the beginning of the annual reporting period in which the entity first applies the amendment.
- For the purposes of applying the requirement in paragraph 5.7.7(a), credit risk is different from asset-specific performance risk. Asset-specific performance risk is not related to the risk that an entity will fail to discharge a particular obligation but instead it is related to the risk that a single asset or a group of assets will perform poorly (or not at all).
- Unless paragraph 6.8.8 applies, for a hedge of a non-contractually specified benchmark component of interest rate risk, an entity shall apply the requirement in paragraphs 6.3.7(a) and B6.3.8—that the risk component shall be separately identifiable—only at the inception of the hedging relationship.
*International Financial Reporting Standards (IFRS) adjustment process involves reviewing the company's financial statements and identifying any differences between the company's current accounting practices and the requirements of the IFRS. If there are any such differences, neural network makes adjustments to financial statements to bring them into compliance with the IFRS.
Conclusions
Rush Street Interactive Inc. Class A Common Stock is assigned short-term Ba1 & long-term Ba1 estimated rating. Rush Street Interactive Inc. Class A Common Stock prediction model is evaluated with Transductive Learning (ML) and Chi-Square1,2,3,4 and it is concluded that the RSI stock is predictable in the short/long term. According to price forecasts for 1 Year period, the dominant strategy among neural network is: Strong Sell
RSI Rush Street Interactive Inc. Class A Common Stock Financial Analysis*
Rating | Short-Term | Long-Term Senior |
---|---|---|
Outlook* | Ba1 | Ba1 |
Income Statement | B2 | B3 |
Balance Sheet | Ba2 | C |
Leverage Ratios | B3 | Baa2 |
Cash Flow | B2 | Caa2 |
Rates of Return and Profitability | Baa2 | C |
*Financial analysis is the process of evaluating a company's financial performance and position by neural network. It involves reviewing the company's financial statements, including the balance sheet, income statement, and cash flow statement, as well as other financial reports and documents.
How does neural network examine financial reports and understand financial state of the company?
Prediction Confidence Score
References
- K. Tuyls and G. Weiss. Multiagent learning: Basics, challenges, and prospects. AI Magazine, 33(3): 41–52, 2012
- V. Mnih, K. Kavukcuoglu, D. Silver, A. Rusu, J. Veness, M. Bellemare, A. Graves, M. Riedmiller, A. Fidjeland, G. Ostrovski, S. Petersen, C. Beattie, A. Sadik, I. Antonoglou, H. King, D. Kumaran, D. Wierstra, S. Legg, and D. Hassabis. Human-level control through deep reinforcement learning. Nature, 518(7540):529–533, 02 2015.
- Miller A. 2002. Subset Selection in Regression. New York: CRC Press
- K. Boda, J. Filar, Y. Lin, and L. Spanjers. Stochastic target hitting time and the problem of early retirement. Automatic Control, IEEE Transactions on, 49(3):409–419, 2004
- Nie X, Wager S. 2019. Quasi-oracle estimation of heterogeneous treatment effects. arXiv:1712.04912 [stat.ML]
- R. Williams. Simple statistical gradient-following algorithms for connectionist reinforcement learning. Ma- chine learning, 8(3-4):229–256, 1992
- D. S. Bernstein, S. Zilberstein, and N. Immerman. The complexity of decentralized control of Markov Decision Processes. In UAI '00: Proceedings of the 16th Conference in Uncertainty in Artificial Intelligence, Stanford University, Stanford, California, USA, June 30 - July 3, 2000, pages 32–37, 2000.
Frequently Asked Questions
Q: What is the prediction methodology for RSI stock?A: RSI stock prediction methodology: We evaluate the prediction models Transductive Learning (ML) and Chi-Square
Q: Is RSI stock a buy or sell?
A: The dominant strategy among neural network is to Strong Sell RSI Stock.
Q: Is Rush Street Interactive Inc. Class A Common Stock stock a good investment?
A: The consensus rating for Rush Street Interactive Inc. Class A Common Stock is Strong Sell and is assigned short-term Ba1 & long-term Ba1 estimated rating.
Q: What is the consensus rating of RSI stock?
A: The consensus rating for RSI is Strong Sell.
Q: What is the prediction period for RSI stock?
A: The prediction period for RSI is 1 Year