Dominant Strategy : Hold
Time series to forecast n: 10 Jun 2023 for 4 Weeks
Methodology : Modular Neural Network (CNN Layer)
Abstract
Anghami Inc. Warrants prediction model is evaluated with Modular Neural Network (CNN Layer) and Polynomial Regression1,2,3,4 and it is concluded that the ANGHW stock is predictable in the short/long term. CNN layers are a powerful tool for extracting features from images. They are able to learn to detect patterns in images that are not easily detected by humans. This makes them well-suited for a variety of MNN applications. According to price forecasts for 4 Weeks period, the dominant strategy among neural network is: Hold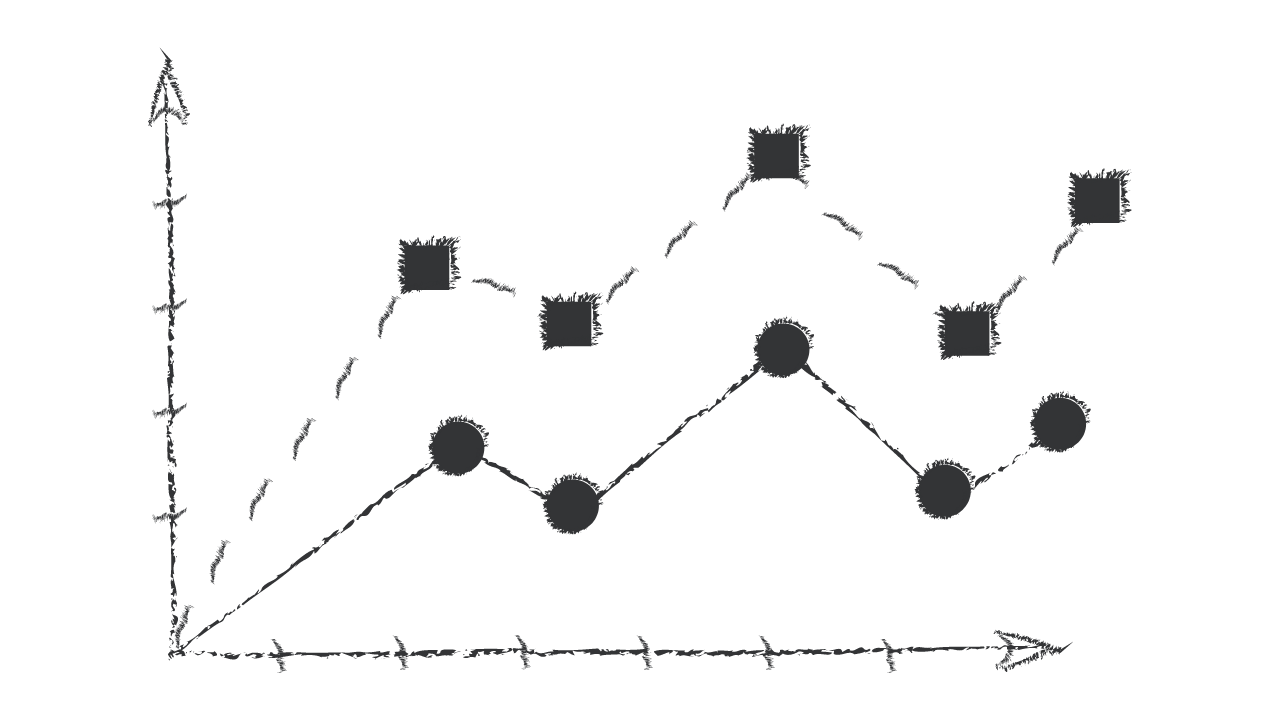
Key Points
- Stock Forecast Based On a Predictive Algorithm
- Trading Interaction
- What is statistical models in machine learning?
ANGHW Target Price Prediction Modeling Methodology
We consider Anghami Inc. Warrants Decision Process with Modular Neural Network (CNN Layer) where A is the set of discrete actions of ANGHW stock holders, F is the set of discrete states, P : S × F × S → R is the transition probability distribution, R : S × F → R is the reaction function, and γ ∈ [0, 1] is a move factor for expectation.1,2,3,4
F(Polynomial Regression)5,6,7= X R(Modular Neural Network (CNN Layer)) X S(n):→ 4 Weeks
n:Time series to forecast
p:Price signals of ANGHW stock
j:Nash equilibria (Neural Network)
k:Dominated move
a:Best response for target price
Modular Neural Network (CNN Layer)
CNN layers are a powerful tool for extracting features from images. They are able to learn to detect patterns in images that are not easily detected by humans. This makes them well-suited for a variety of MNN applications.Polynomial Regression
Polynomial regression is a type of regression analysis that uses a polynomial function to model the relationship between a dependent variable and one or more independent variables. Polynomial functions are mathematical functions that have a polynomial term, which is a term that is raised to a power greater than 1. In polynomial regression, the dependent variable is modeled as a polynomial function of the independent variables. The degree of the polynomial function is determined by the researcher. The higher the degree of the polynomial function, the more complex the model will be.
For further technical information as per how our model work we invite you to visit the article below:
How do AC Investment Research machine learning (predictive) algorithms actually work?
ANGHW Stock Forecast (Buy or Sell) for 4 Weeks
Sample Set: Neural NetworkStock/Index: ANGHW Anghami Inc. Warrants
Time series to forecast n: 10 Jun 2023 for 4 Weeks
According to price forecasts for 4 Weeks period, the dominant strategy among neural network is: Hold
X axis: *Likelihood% (The higher the percentage value, the more likely the event will occur.)
Y axis: *Potential Impact% (The higher the percentage value, the more likely the price will deviate.)
Z axis (Grey to Black): *Technical Analysis%
IFRS Reconciliation Adjustments for Anghami Inc. Warrants
- Sales that occur for other reasons, such as sales made to manage credit concentration risk (without an increase in the assets' credit risk), may also be consistent with a business model whose objective is to hold financial assets in order to collect contractual cash flows. In particular, such sales may be consistent with a business model whose objective is to hold financial assets in order to collect contractual cash flows if those sales are infrequent (even if significant in value) or insignificant in value both individually and in aggregate (even if frequent). If more than an infrequent number of such sales are made out of a portfolio and those sales are more than insignificant in value (either individually or in aggregate), the entity needs to assess whether and how such sales are consistent with an objective of collecting contractual cash flows. Whether a third party imposes the requirement to sell the financial assets, or that activity is at the entity's discretion, is not relevant to this assessment. An increase in the frequency or value of sales in a particular period is not necessarily inconsistent with an objective to hold financial assets in order to collect contractual cash flows, if an entity can explain the reasons for those sales and demonstrate why those sales do not reflect a change in the entity's business model. In addition, sales may be consistent with the objective of holding financial assets in order to collect contractual cash flows if the sales are made close to the maturity of the financial assets and the proceeds from the sales approximate the collection of the remaining contractual cash flows.
- For the purpose of applying paragraphs B4.1.11(b) and B4.1.12(b), irrespective of the event or circumstance that causes the early termination of the contract, a party may pay or receive reasonable compensation for that early termination. For example, a party may pay or receive reasonable compensation when it chooses to terminate the contract early (or otherwise causes the early termination to occur).
- If changes are made in addition to those changes required by interest rate benchmark reform to the financial asset or financial liability designated in a hedging relationship (as described in paragraphs 5.4.6–5.4.8) or to the designation of the hedging relationship (as required by paragraph 6.9.1), an entity shall first apply the applicable requirements in this Standard to determine if those additional changes result in the discontinuation of hedge accounting. If the additional changes do not result in the discontinuation of hedge accounting, an entity shall amend the formal designation of the hedging relationship as specified in paragraph 6.9.1.
- At the date of initial application, an entity shall assess whether a financial asset meets the condition in paragraphs 4.1.2(a) or 4.1.2A(a) on the basis of the facts and circumstances that exist at that date. The resulting classification shall be applied retrospectively irrespective of the entity's business model in prior reporting periods.
*International Financial Reporting Standards (IFRS) adjustment process involves reviewing the company's financial statements and identifying any differences between the company's current accounting practices and the requirements of the IFRS. If there are any such differences, neural network makes adjustments to financial statements to bring them into compliance with the IFRS.
Conclusions
Anghami Inc. Warrants is assigned short-term Ba1 & long-term Ba1 estimated rating. Anghami Inc. Warrants prediction model is evaluated with Modular Neural Network (CNN Layer) and Polynomial Regression1,2,3,4 and it is concluded that the ANGHW stock is predictable in the short/long term. According to price forecasts for 4 Weeks period, the dominant strategy among neural network is: Hold
ANGHW Anghami Inc. Warrants Financial Analysis*
Rating | Short-Term | Long-Term Senior |
---|---|---|
Outlook* | Ba1 | Ba1 |
Income Statement | C | C |
Balance Sheet | B2 | Baa2 |
Leverage Ratios | Baa2 | Ba2 |
Cash Flow | Baa2 | Caa2 |
Rates of Return and Profitability | Ba1 | C |
*Financial analysis is the process of evaluating a company's financial performance and position by neural network. It involves reviewing the company's financial statements, including the balance sheet, income statement, and cash flow statement, as well as other financial reports and documents.
How does neural network examine financial reports and understand financial state of the company?
Prediction Confidence Score
References
- uyer, S. Whiteson, B. Bakker, and N. A. Vlassis. Multiagent reinforcement learning for urban traffic control using coordination graphs. In Machine Learning and Knowledge Discovery in Databases, European Conference, ECML/PKDD 2008, Antwerp, Belgium, September 15-19, 2008, Proceedings, Part I, pages 656–671, 2008.
- J. Baxter and P. Bartlett. Infinite-horizon policy-gradient estimation. Journal of Artificial Intelligence Re- search, 15:319–350, 2001.
- Belloni A, Chernozhukov V, Hansen C. 2014. High-dimensional methods and inference on structural and treatment effects. J. Econ. Perspect. 28:29–50
- Hastie T, Tibshirani R, Friedman J. 2009. The Elements of Statistical Learning. Berlin: Springer
- Bickel P, Klaassen C, Ritov Y, Wellner J. 1998. Efficient and Adaptive Estimation for Semiparametric Models. Berlin: Springer
- Bell RM, Koren Y. 2007. Lessons from the Netflix prize challenge. ACM SIGKDD Explor. Newsl. 9:75–79
- Swaminathan A, Joachims T. 2015. Batch learning from logged bandit feedback through counterfactual risk minimization. J. Mach. Learn. Res. 16:1731–55
Frequently Asked Questions
Q: What is the prediction methodology for ANGHW stock?A: ANGHW stock prediction methodology: We evaluate the prediction models Modular Neural Network (CNN Layer) and Polynomial Regression
Q: Is ANGHW stock a buy or sell?
A: The dominant strategy among neural network is to Hold ANGHW Stock.
Q: Is Anghami Inc. Warrants stock a good investment?
A: The consensus rating for Anghami Inc. Warrants is Hold and is assigned short-term Ba1 & long-term Ba1 estimated rating.
Q: What is the consensus rating of ANGHW stock?
A: The consensus rating for ANGHW is Hold.
Q: What is the prediction period for ANGHW stock?
A: The prediction period for ANGHW is 4 Weeks