AUC Score :
Short-Term Revised :
Dominant Strategy : Buy
Time series to forecast n:
Methodology : Inductive Learning (ML)
Hypothesis Testing : Linear Regression
Surveillance : Major exchange and OTC
Summary
Tastemaker Acquisition Corp. Class A Common Stock prediction model is evaluated with Inductive Learning (ML) and Linear Regression1,2,3,4 and it is concluded that the TMKR stock is predictable in the short/long term. Inductive learning is a type of machine learning in which the model learns from a set of labeled data and makes predictions about new, unlabeled data. The model is trained on the labeled data and then used to make predictions on new data. Inductive learning is a supervised learning algorithm, which means that it requires labeled data to train. The labeled data is used to train the model to make predictions about new data. There are many different types of inductive learning algorithms, including decision trees, support vector machines, and neural networks. Each type of algorithm has its own strengths and weaknesses. According to price forecasts for 6 Month period, the dominant strategy among neural network is: Buy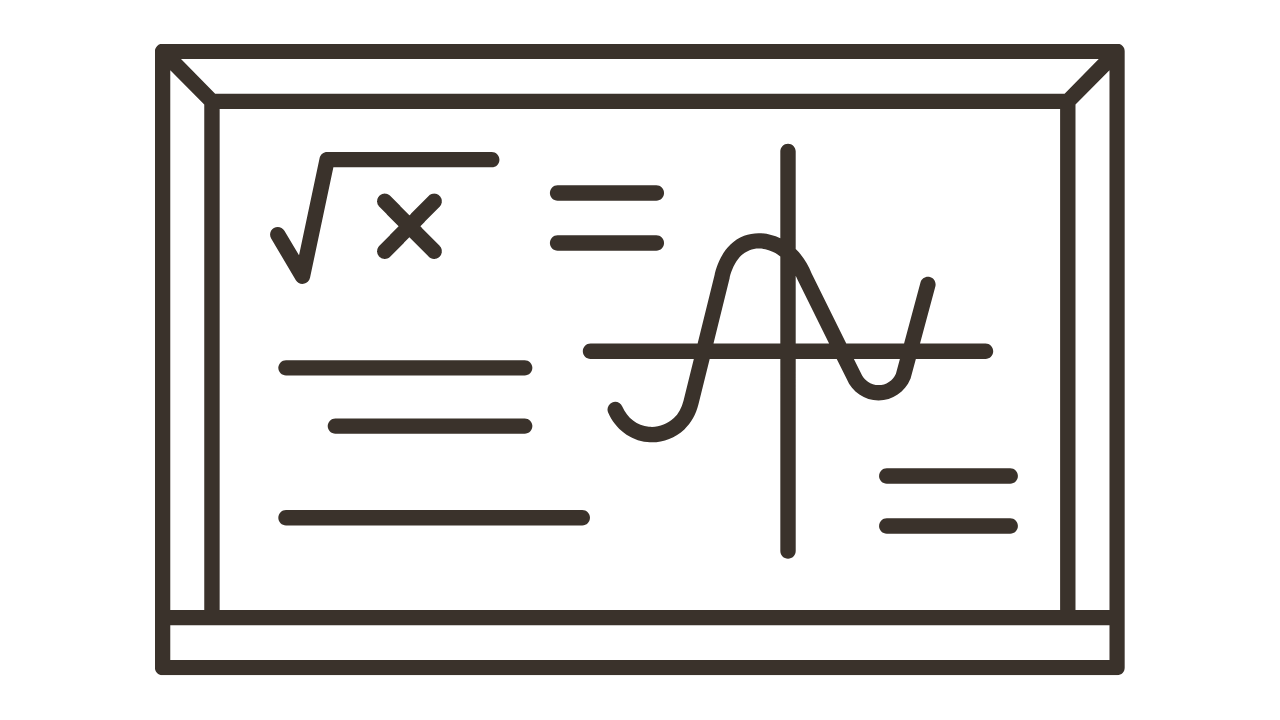
Key Points
- Short/Long Term Stocks
- Why do we need predictive models?
- Understanding Buy, Sell, and Hold Ratings
TMKR Target Price Prediction Modeling Methodology
We consider Tastemaker Acquisition Corp. Class A Common Stock Decision Process with Inductive Learning (ML) where A is the set of discrete actions of TMKR stock holders, F is the set of discrete states, P : S × F × S → R is the transition probability distribution, R : S × F → R is the reaction function, and γ ∈ [0, 1] is a move factor for expectation.1,2,3,4
F(Linear Regression)5,6,7= X R(Inductive Learning (ML)) X S(n):→ 6 Month
n:Time series to forecast
p:Price signals of TMKR stock
j:Nash equilibria (Neural Network)
k:Dominated move
a:Best response for target price
Inductive Learning (ML)
Inductive learning is a type of machine learning in which the model learns from a set of labeled data and makes predictions about new, unlabeled data. The model is trained on the labeled data and then used to make predictions on new data. Inductive learning is a supervised learning algorithm, which means that it requires labeled data to train. The labeled data is used to train the model to make predictions about new data. There are many different types of inductive learning algorithms, including decision trees, support vector machines, and neural networks. Each type of algorithm has its own strengths and weaknesses.Linear Regression
In statistics, linear regression is a method for estimating the relationship between a dependent variable and one or more independent variables. The dependent variable is the variable that is being predicted, and the independent variables are the variables that are used to predict the dependent variable. Linear regression assumes that the relationship between the dependent variable and the independent variables is linear. This means that the dependent variable can be represented as a straight line function of the independent variables.
For further technical information as per how our model work we invite you to visit the article below:
How do AC Investment Research machine learning (predictive) algorithms actually work?
TMKR Stock Forecast (Buy or Sell) for 6 Month
Sample Set: Neural NetworkStock/Index: TMKR Tastemaker Acquisition Corp. Class A Common Stock
Time series to forecast: 6 Month
According to price forecasts for 6 Month period, the dominant strategy among neural network is: Buy
Strategic Interaction Table Legend:
X axis: *Likelihood% (The higher the percentage value, the more likely the event will occur.)
Y axis: *Potential Impact% (The higher the percentage value, the more likely the price will deviate.)
Z axis (Grey to Black): *Technical Analysis%
Financial Data Adjustments for Inductive Learning (ML) based TMKR Stock Prediction Model
- In applying the effective interest method, an entity identifies fees that are an integral part of the effective interest rate of a financial instrument. The description of fees for financial services may not be indicative of the nature and substance of the services provided. Fees that are an integral part of the effective interest rate of a financial instrument are treated as an adjustment to the effective interest rate, unless the financial instrument is measured at fair value, with the change in fair value being recognised in profit or loss. In those cases, the fees are recognised as revenue or expense when the instrument is initially recognised.
- An example of a fair value hedge is a hedge of exposure to changes in the fair value of a fixed-rate debt instrument arising from changes in interest rates. Such a hedge could be entered into by the issuer or by the holder.
- Paragraph 4.1.1(a) requires an entity to classify financial assets on the basis of the entity's business model for managing the financial assets, unless paragraph 4.1.5 applies. An entity assesses whether its financial assets meet the condition in paragraph 4.1.2(a) or the condition in paragraph 4.1.2A(a) on the basis of the business model as determined by the entity's key management personnel (as defined in IAS 24 Related Party Disclosures).
- When defining default for the purposes of determining the risk of a default occurring, an entity shall apply a default definition that is consistent with the definition used for internal credit risk management purposes for the relevant financial instrument and consider qualitative indicators (for example, financial covenants) when appropriate. However, there is a rebuttable presumption that default does not occur later than when a financial asset is 90 days past due unless an entity has reasonable and supportable information to demonstrate that a more lagging default criterion is more appropriate. The definition of default used for these purposes shall be applied consistently to all financial instruments unless information becomes available that demonstrates that another default definition is more appropriate for a particular financial instrument.
*International Financial Reporting Standards (IFRS) adjustment process involves reviewing the company's financial statements and identifying any differences between the company's current accounting practices and the requirements of the IFRS. If there are any such differences, neural network makes adjustments to financial statements to bring them into compliance with the IFRS.
TMKR Tastemaker Acquisition Corp. Class A Common Stock Financial Analysis*
Rating | Short-Term | Long-Term Senior |
---|---|---|
Outlook* | B1 | B2 |
Income Statement | Baa2 | C |
Balance Sheet | Caa2 | B1 |
Leverage Ratios | C | B3 |
Cash Flow | Baa2 | Baa2 |
Rates of Return and Profitability | Ba2 | C |
*Financial analysis is the process of evaluating a company's financial performance and position by neural network. It involves reviewing the company's financial statements, including the balance sheet, income statement, and cash flow statement, as well as other financial reports and documents.
How does neural network examine financial reports and understand financial state of the company?
Conclusions
Tastemaker Acquisition Corp. Class A Common Stock is assigned short-term B1 & long-term B2 estimated rating. Tastemaker Acquisition Corp. Class A Common Stock prediction model is evaluated with Inductive Learning (ML) and Linear Regression1,2,3,4 and it is concluded that the TMKR stock is predictable in the short/long term. According to price forecasts for 6 Month period, the dominant strategy among neural network is: Buy
Prediction Confidence Score
References
- Thomas P, Brunskill E. 2016. Data-efficient off-policy policy evaluation for reinforcement learning. In Pro- ceedings of the International Conference on Machine Learning, pp. 2139–48. La Jolla, CA: Int. Mach. Learn. Soc.
- Bastani H, Bayati M. 2015. Online decision-making with high-dimensional covariates. Work. Pap., Univ. Penn./ Stanford Grad. School Bus., Philadelphia/Stanford, CA
- Abadie A, Diamond A, Hainmueller J. 2010. Synthetic control methods for comparative case studies: estimat- ing the effect of California's tobacco control program. J. Am. Stat. Assoc. 105:493–505
- Li L, Chu W, Langford J, Moon T, Wang X. 2012. An unbiased offline evaluation of contextual bandit algo- rithms with generalized linear models. In Proceedings of 4th ACM International Conference on Web Search and Data Mining, pp. 297–306. New York: ACM
- D. Bertsekas. Dynamic programming and optimal control. Athena Scientific, 1995.
- ZXhang, Y.X., Haxo, Y.M. and Mat, Y.X., 2023. The Dow Jones Industrial Average (No. Stock Analysis). AC Investment Research.
- ZXhang, Y.X., Haxo, Y.M. and Mat, Y.X., 2023. Can Neural Networks Predict Stock Market? (No. Stock Analysis). AC Investment Research.
Frequently Asked Questions
Q: What is the prediction methodology for TMKR stock?A: TMKR stock prediction methodology: We evaluate the prediction models Inductive Learning (ML) and Linear Regression
Q: Is TMKR stock a buy or sell?
A: The dominant strategy among neural network is to Buy TMKR Stock.
Q: Is Tastemaker Acquisition Corp. Class A Common Stock stock a good investment?
A: The consensus rating for Tastemaker Acquisition Corp. Class A Common Stock is Buy and is assigned short-term B1 & long-term B2 estimated rating.
Q: What is the consensus rating of TMKR stock?
A: The consensus rating for TMKR is Buy.
Q: What is the prediction period for TMKR stock?
A: The prediction period for TMKR is 6 Month