Dominant Strategy : Sell
Time series to forecast n: 10 Jun 2023 for 1 Year
Methodology : Transductive Learning (ML)
Abstract
PetroTal Corp. prediction model is evaluated with Transductive Learning (ML) and Linear Regression1,2,3,4 and it is concluded that the TAL:TSXV stock is predictable in the short/long term. Transductive learning is a supervised machine learning (ML) method in which the model is trained on both labeled and unlabeled data. The goal of transductive learning is to predict the labels of the unlabeled data. Transductive learning is a hybrid of inductive and semi-supervised learning. Inductive learning algorithms are trained on labeled data only, while semi-supervised learning algorithms are trained on a combination of labeled and unlabeled data. Transductive learning algorithms can achieve better performance than inductive learning algorithms on tasks where there is a small amount of labeled data. This is because transductive learning algorithms can use the unlabeled data to help them learn the relationships between the features and the labels. According to price forecasts for 1 Year period, the dominant strategy among neural network is: Sell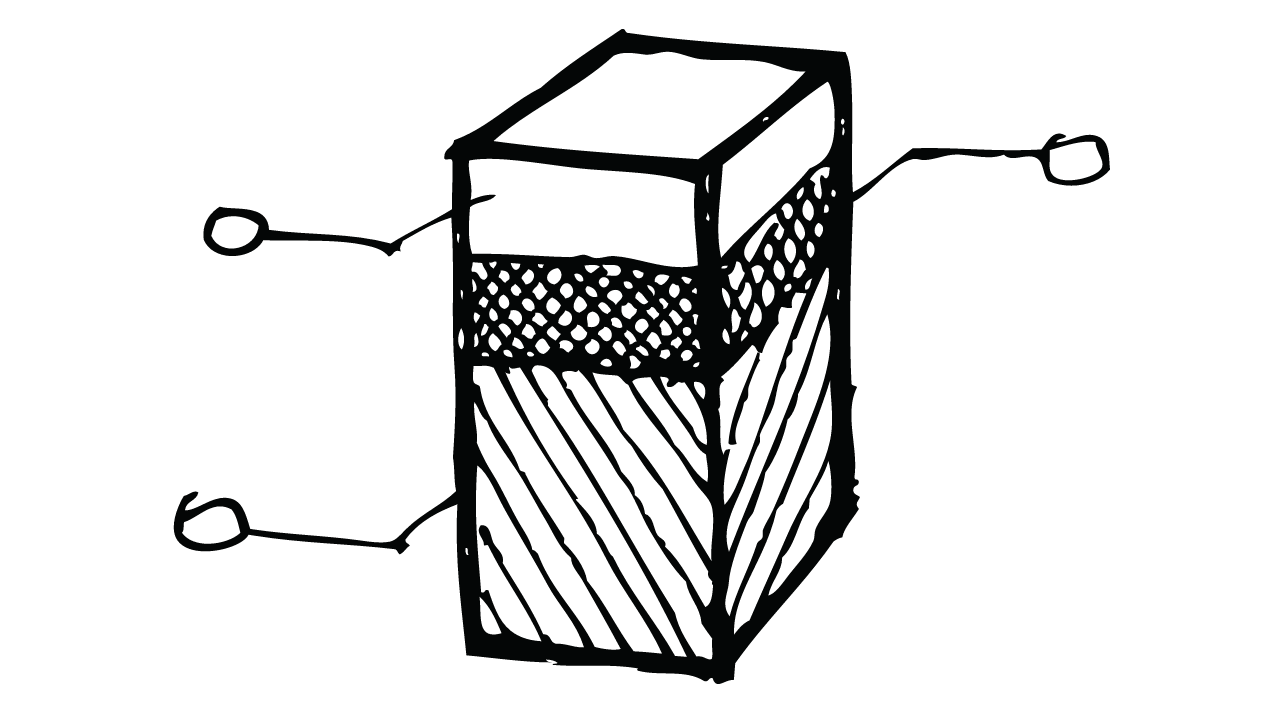
Key Points
- Short/Long Term Stocks
- Short/Long Term Stocks
- How do you know when a stock will go up or down?
TAL:TSXV Target Price Prediction Modeling Methodology
We consider PetroTal Corp. Decision Process with Transductive Learning (ML) where A is the set of discrete actions of TAL:TSXV stock holders, F is the set of discrete states, P : S × F × S → R is the transition probability distribution, R : S × F → R is the reaction function, and γ ∈ [0, 1] is a move factor for expectation.1,2,3,4
F(Linear Regression)5,6,7= X R(Transductive Learning (ML)) X S(n):→ 1 Year
n:Time series to forecast
p:Price signals of TAL:TSXV stock
j:Nash equilibria (Neural Network)
k:Dominated move
a:Best response for target price
Transductive Learning (ML)
Transductive learning is a supervised machine learning (ML) method in which the model is trained on both labeled and unlabeled data. The goal of transductive learning is to predict the labels of the unlabeled data. Transductive learning is a hybrid of inductive and semi-supervised learning. Inductive learning algorithms are trained on labeled data only, while semi-supervised learning algorithms are trained on a combination of labeled and unlabeled data. Transductive learning algorithms can achieve better performance than inductive learning algorithms on tasks where there is a small amount of labeled data. This is because transductive learning algorithms can use the unlabeled data to help them learn the relationships between the features and the labels.Linear Regression
In statistics, linear regression is a method for estimating the relationship between a dependent variable and one or more independent variables. The dependent variable is the variable that is being predicted, and the independent variables are the variables that are used to predict the dependent variable. Linear regression assumes that the relationship between the dependent variable and the independent variables is linear. This means that the dependent variable can be represented as a straight line function of the independent variables.
For further technical information as per how our model work we invite you to visit the article below:
How do AC Investment Research machine learning (predictive) algorithms actually work?
TAL:TSXV Stock Forecast (Buy or Sell) for 1 Year
Sample Set: Neural NetworkStock/Index: TAL:TSXV PetroTal Corp.
Time series to forecast n: 10 Jun 2023 for 1 Year
According to price forecasts for 1 Year period, the dominant strategy among neural network is: Sell
X axis: *Likelihood% (The higher the percentage value, the more likely the event will occur.)
Y axis: *Potential Impact% (The higher the percentage value, the more likely the price will deviate.)
Z axis (Grey to Black): *Technical Analysis%
IFRS Reconciliation Adjustments for PetroTal Corp.
- At the date of initial application, an entity shall determine whether the treatment in paragraph 5.7.7 would create or enlarge an accounting mismatch in profit or loss on the basis of the facts and circumstances that exist at the date of initial application. This Standard shall be applied retrospectively on the basis of that determination.
- For example, when the critical terms (such as the nominal amount, maturity and underlying) of the hedging instrument and the hedged item match or are closely aligned, it might be possible for an entity to conclude on the basis of a qualitative assessment of those critical terms that the hedging instrument and the hedged item have values that will generally move in the opposite direction because of the same risk and hence that an economic relationship exists between the hedged item and the hedging instrument (see paragraphs B6.4.4–B6.4.6).
- An entity can also designate only changes in the cash flows or fair value of a hedged item above or below a specified price or other variable (a 'one-sided risk'). The intrinsic value of a purchased option hedging instrument (assuming that it has the same principal terms as the designated risk), but not its time value, reflects a one-sided risk in a hedged item. For example, an entity can designate the variability of future cash flow outcomes resulting from a price increase of a forecast commodity purchase. In such a situation, the entity designates only cash flow losses that result from an increase in the price above the specified level. The hedged risk does not include the time value of a purchased option, because the time value is not a component of the forecast transaction that affects profit or loss.
- If an entity has applied paragraph 7.2.6 then at the date of initial application the entity shall recognise any difference between the fair value of the entire hybrid contract at the date of initial application and the sum of the fair values of the components of the hybrid contract at the date of initial application in the opening retained earnings (or other component of equity, as appropriate) of the reporting period that includes the date of initial application.
*International Financial Reporting Standards (IFRS) adjustment process involves reviewing the company's financial statements and identifying any differences between the company's current accounting practices and the requirements of the IFRS. If there are any such differences, neural network makes adjustments to financial statements to bring them into compliance with the IFRS.
Conclusions
PetroTal Corp. is assigned short-term Ba1 & long-term Ba1 estimated rating. PetroTal Corp. prediction model is evaluated with Transductive Learning (ML) and Linear Regression1,2,3,4 and it is concluded that the TAL:TSXV stock is predictable in the short/long term. According to price forecasts for 1 Year period, the dominant strategy among neural network is: Sell
TAL:TSXV PetroTal Corp. Financial Analysis*
Rating | Short-Term | Long-Term Senior |
---|---|---|
Outlook* | Ba1 | Ba1 |
Income Statement | B2 | Baa2 |
Balance Sheet | Baa2 | B2 |
Leverage Ratios | B2 | Baa2 |
Cash Flow | Baa2 | Caa2 |
Rates of Return and Profitability | Caa2 | Caa2 |
*Financial analysis is the process of evaluating a company's financial performance and position by neural network. It involves reviewing the company's financial statements, including the balance sheet, income statement, and cash flow statement, as well as other financial reports and documents.
How does neural network examine financial reports and understand financial state of the company?
Prediction Confidence Score
References
- Akgiray, V. (1989), "Conditional heteroscedasticity in time series of stock returns: Evidence and forecasts," Journal of Business, 62, 55–80.
- Bengio Y, Schwenk H, Senécal JS, Morin F, Gauvain JL. 2006. Neural probabilistic language models. In Innovations in Machine Learning: Theory and Applications, ed. DE Holmes, pp. 137–86. Berlin: Springer
- Angrist JD, Pischke JS. 2008. Mostly Harmless Econometrics: An Empiricist's Companion. Princeton, NJ: Princeton Univ. Press
- E. Altman, K. Avrachenkov, and R. N ́u ̃nez-Queija. Perturbation analysis for denumerable Markov chains with application to queueing models. Advances in Applied Probability, pages 839–853, 2004
- Abadir, K. M., K. Hadri E. Tzavalis (1999), "The influence of VAR dimensions on estimator biases," Econometrica, 67, 163–181.
- Bessler, D. A. R. A. Babula, (1987), "Forecasting wheat exports: Do exchange rates matter?" Journal of Business and Economic Statistics, 5, 397–406.
- Bengio Y, Schwenk H, Senécal JS, Morin F, Gauvain JL. 2006. Neural probabilistic language models. In Innovations in Machine Learning: Theory and Applications, ed. DE Holmes, pp. 137–86. Berlin: Springer
Frequently Asked Questions
Q: What is the prediction methodology for TAL:TSXV stock?A: TAL:TSXV stock prediction methodology: We evaluate the prediction models Transductive Learning (ML) and Linear Regression
Q: Is TAL:TSXV stock a buy or sell?
A: The dominant strategy among neural network is to Sell TAL:TSXV Stock.
Q: Is PetroTal Corp. stock a good investment?
A: The consensus rating for PetroTal Corp. is Sell and is assigned short-term Ba1 & long-term Ba1 estimated rating.
Q: What is the consensus rating of TAL:TSXV stock?
A: The consensus rating for TAL:TSXV is Sell.
Q: What is the prediction period for TAL:TSXV stock?
A: The prediction period for TAL:TSXV is 1 Year