Dominant Strategy : Sell
Time series to forecast n: 11 Jun 2023 for 4 Weeks
Methodology : Multi-Task Learning (ML)
Abstract
Perseus Mining Limited prediction model is evaluated with Multi-Task Learning (ML) and Multiple Regression1,2,3,4 and it is concluded that the PRU:TSX stock is predictable in the short/long term. Multi-task learning (MTL) is a machine learning (ML) method in which multiple related tasks are learned simultaneously. This can be done by sharing features and weights between the tasks. MTL has been shown to improve the performance of each task, compared to learning each task independently. According to price forecasts for 4 Weeks period, the dominant strategy among neural network is: Sell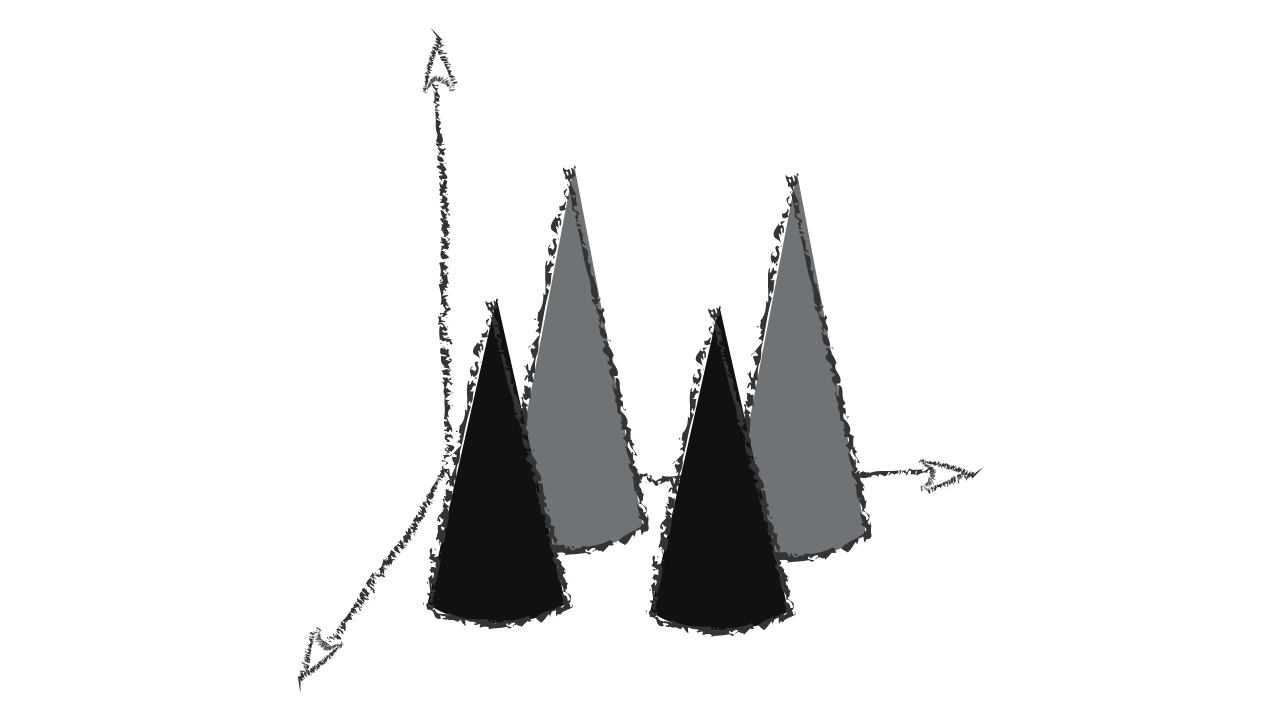
Key Points
- What are the most successful trading algorithms?
- Market Outlook
- Dominated Move
PRU:TSX Target Price Prediction Modeling Methodology
We consider Perseus Mining Limited Decision Process with Multi-Task Learning (ML) where A is the set of discrete actions of PRU:TSX stock holders, F is the set of discrete states, P : S × F × S → R is the transition probability distribution, R : S × F → R is the reaction function, and γ ∈ [0, 1] is a move factor for expectation.1,2,3,4
F(Multiple Regression)5,6,7= X R(Multi-Task Learning (ML)) X S(n):→ 4 Weeks
n:Time series to forecast
p:Price signals of PRU:TSX stock
j:Nash equilibria (Neural Network)
k:Dominated move
a:Best response for target price
Multi-Task Learning (ML)
Multi-task learning (MTL) is a machine learning (ML) method in which multiple related tasks are learned simultaneously. This can be done by sharing features and weights between the tasks. MTL has been shown to improve the performance of each task, compared to learning each task independently.Multiple Regression
Multiple regression is a statistical method that analyzes the relationship between a dependent variable and multiple independent variables. The dependent variable is the variable that is being predicted, and the independent variables are the variables that are used to predict the dependent variable. Multiple regression is a more complex statistical method than simple linear regression, which only analyzes the relationship between a dependent variable and one independent variable. Multiple regression can be used to analyze more complex relationships between variables, and it can also be used to control for confounding variables. A confounding variable is a variable that is correlated with both the dependent variable and one or more of the independent variables. Confounding variables can distort the relationship between the dependent variable and the independent variables. Multiple regression can be used to control for confounding variables by including them in the model.
For further technical information as per how our model work we invite you to visit the article below:
How do AC Investment Research machine learning (predictive) algorithms actually work?
PRU:TSX Stock Forecast (Buy or Sell) for 4 Weeks
Sample Set: Neural NetworkStock/Index: PRU:TSX Perseus Mining Limited
Time series to forecast n: 11 Jun 2023 for 4 Weeks
According to price forecasts for 4 Weeks period, the dominant strategy among neural network is: Sell
X axis: *Likelihood% (The higher the percentage value, the more likely the event will occur.)
Y axis: *Potential Impact% (The higher the percentage value, the more likely the price will deviate.)
Z axis (Grey to Black): *Technical Analysis%
IFRS Reconciliation Adjustments for Perseus Mining Limited
- A similar example of a non-financial item is a specific type of crude oil from a particular oil field that is priced off the relevant benchmark crude oil. If an entity sells that crude oil under a contract using a contractual pricing formula that sets the price per barrel at the benchmark crude oil price minus CU10 with a floor of CU15, the entity can designate as the hedged item the entire cash flow variability under the sales contract that is attributable to the change in the benchmark crude oil price. However, the entity cannot designate a component that is equal to the full change in the benchmark crude oil price. Hence, as long as the forward price (for each delivery) does not fall below CU25, the hedged item has the same cash flow variability as a crude oil sale at the benchmark crude oil price (or with a positive spread). However, if the forward price for any delivery falls below CU25, the hedged item has a lower cash flow variability than a crude oil sale at the benchmark crude oil price (or with a positive spread).
- Adjusting the hedge ratio by decreasing the volume of the hedged item does not affect how the changes in the fair value of the hedging instrument are measured. The measurement of the changes in the value of the hedged item related to the volume that continues to be designated also remains unaffected. However, from the date of rebalancing, the volume by which the hedged item was decreased is no longer part of the hedging relationship. For example, if an entity originally hedged a volume of 100 tonnes of a commodity at a forward price of CU80 and reduces that volume by 10 tonnes on rebalancing, the hedged item after rebalancing would be 90 tonnes hedged at CU80. The 10 tonnes of the hedged item that are no longer part of the hedging relationship would be accounted for in accordance with the requirements for the discontinuation of hedge accounting (see paragraphs 6.5.6–6.5.7 and B6.5.22–B6.5.28).
- An entity's documentation of the hedging relationship includes how it will assess the hedge effectiveness requirements, including the method or methods used. The documentation of the hedging relationship shall be updated for any changes to the methods (see paragraph B6.4.17).
- To the extent that a transfer of a financial asset does not qualify for derecognition, the transferee does not recognise the transferred asset as its asset. The transferee derecognises the cash or other consideration paid and recognises a receivable from the transferor. If the transferor has both a right and an obligation to reacquire control of the entire transferred asset for a fixed amount (such as under a repurchase agreement), the transferee may measure its receivable at amortised cost if it meets the criteria in paragraph 4.1.2.
*International Financial Reporting Standards (IFRS) adjustment process involves reviewing the company's financial statements and identifying any differences between the company's current accounting practices and the requirements of the IFRS. If there are any such differences, neural network makes adjustments to financial statements to bring them into compliance with the IFRS.
Conclusions
Perseus Mining Limited is assigned short-term Ba1 & long-term Ba1 estimated rating. Perseus Mining Limited prediction model is evaluated with Multi-Task Learning (ML) and Multiple Regression1,2,3,4 and it is concluded that the PRU:TSX stock is predictable in the short/long term. According to price forecasts for 4 Weeks period, the dominant strategy among neural network is: Sell
PRU:TSX Perseus Mining Limited Financial Analysis*
Rating | Short-Term | Long-Term Senior |
---|---|---|
Outlook* | Ba1 | Ba1 |
Income Statement | C | Baa2 |
Balance Sheet | Caa2 | Caa2 |
Leverage Ratios | Baa2 | Baa2 |
Cash Flow | Caa2 | Baa2 |
Rates of Return and Profitability | Ba3 | Baa2 |
*Financial analysis is the process of evaluating a company's financial performance and position by neural network. It involves reviewing the company's financial statements, including the balance sheet, income statement, and cash flow statement, as well as other financial reports and documents.
How does neural network examine financial reports and understand financial state of the company?
Prediction Confidence Score
References
- Chen X. 2007. Large sample sieve estimation of semi-nonparametric models. In Handbook of Econometrics, Vol. 6B, ed. JJ Heckman, EE Learner, pp. 5549–632. Amsterdam: Elsevier
- Alexander, J. C. Jr. (1995), "Refining the degree of earnings surprise: A comparison of statistical and analysts' forecasts," Financial Review, 30, 469–506.
- Dudik M, Langford J, Li L. 2011. Doubly robust policy evaluation and learning. In Proceedings of the 28th International Conference on Machine Learning, pp. 1097–104. La Jolla, CA: Int. Mach. Learn. Soc.
- M. J. Hausknecht. Cooperation and Communication in Multiagent Deep Reinforcement Learning. PhD thesis, The University of Texas at Austin, 2016
- Zubizarreta JR. 2015. Stable weights that balance covariates for estimation with incomplete outcome data. J. Am. Stat. Assoc. 110:910–22
- R. Rockafellar and S. Uryasev. Conditional value-at-risk for general loss distributions. Journal of Banking and Finance, 26(7):1443 – 1471, 2002
- Athey S, Mobius MM, Pál J. 2017c. The impact of aggregators on internet news consumption. Unpublished manuscript, Grad. School Bus., Stanford Univ., Stanford, CA
Frequently Asked Questions
Q: What is the prediction methodology for PRU:TSX stock?A: PRU:TSX stock prediction methodology: We evaluate the prediction models Multi-Task Learning (ML) and Multiple Regression
Q: Is PRU:TSX stock a buy or sell?
A: The dominant strategy among neural network is to Sell PRU:TSX Stock.
Q: Is Perseus Mining Limited stock a good investment?
A: The consensus rating for Perseus Mining Limited is Sell and is assigned short-term Ba1 & long-term Ba1 estimated rating.
Q: What is the consensus rating of PRU:TSX stock?
A: The consensus rating for PRU:TSX is Sell.
Q: What is the prediction period for PRU:TSX stock?
A: The prediction period for PRU:TSX is 4 Weeks