AUC Score :
Short-Term Revised :
Dominant Strategy : Speculative Trend
Time series to forecast n:
Methodology : Multi-Task Learning (ML)
Hypothesis Testing : Multiple Regression
Surveillance : Major exchange and OTC
Summary
MPLX LP Common Units Representing Limited Partner Interests prediction model is evaluated with Multi-Task Learning (ML) and Multiple Regression1,2,3,4 and it is concluded that the MPLX stock is predictable in the short/long term. Multi-task learning (MTL) is a machine learning (ML) method in which multiple related tasks are learned simultaneously. This can be done by sharing features and weights between the tasks. MTL has been shown to improve the performance of each task, compared to learning each task independently. According to price forecasts for 16 Weeks period, the dominant strategy among neural network is: Speculative Trend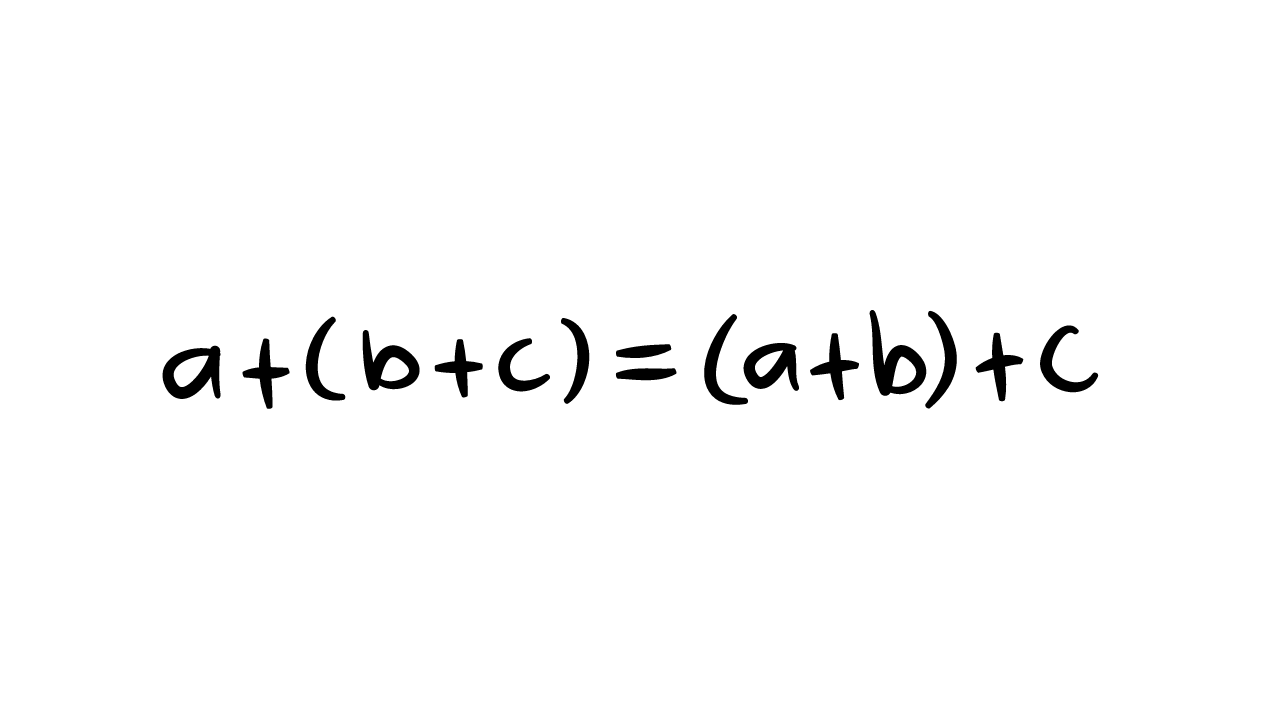
Key Points
- Can statistics predict the future?
- Operational Risk
- Is it better to buy and sell or hold?
MPLX Target Price Prediction Modeling Methodology
We consider MPLX LP Common Units Representing Limited Partner Interests Decision Process with Multi-Task Learning (ML) where A is the set of discrete actions of MPLX stock holders, F is the set of discrete states, P : S × F × S → R is the transition probability distribution, R : S × F → R is the reaction function, and γ ∈ [0, 1] is a move factor for expectation.1,2,3,4
F(Multiple Regression)5,6,7= X R(Multi-Task Learning (ML)) X S(n):→ 16 Weeks
n:Time series to forecast
p:Price signals of MPLX stock
j:Nash equilibria (Neural Network)
k:Dominated move
a:Best response for target price
Multi-Task Learning (ML)
Multi-task learning (MTL) is a machine learning (ML) method in which multiple related tasks are learned simultaneously. This can be done by sharing features and weights between the tasks. MTL has been shown to improve the performance of each task, compared to learning each task independently.Multiple Regression
Multiple regression is a statistical method that analyzes the relationship between a dependent variable and multiple independent variables. The dependent variable is the variable that is being predicted, and the independent variables are the variables that are used to predict the dependent variable. Multiple regression is a more complex statistical method than simple linear regression, which only analyzes the relationship between a dependent variable and one independent variable. Multiple regression can be used to analyze more complex relationships between variables, and it can also be used to control for confounding variables. A confounding variable is a variable that is correlated with both the dependent variable and one or more of the independent variables. Confounding variables can distort the relationship between the dependent variable and the independent variables. Multiple regression can be used to control for confounding variables by including them in the model.
For further technical information as per how our model work we invite you to visit the article below:
How do AC Investment Research machine learning (predictive) algorithms actually work?
MPLX Stock Forecast (Buy or Sell) for 16 Weeks
Sample Set: Neural NetworkStock/Index: MPLX MPLX LP Common Units Representing Limited Partner Interests
Time series to forecast: 16 Weeks
According to price forecasts for 16 Weeks period, the dominant strategy among neural network is: Speculative Trend
Strategic Interaction Table Legend:
X axis: *Likelihood% (The higher the percentage value, the more likely the event will occur.)
Y axis: *Potential Impact% (The higher the percentage value, the more likely the price will deviate.)
Z axis (Grey to Black): *Technical Analysis%
Financial Data Adjustments for Multi-Task Learning (ML) based MPLX Stock Prediction Model
- If a put option written by an entity prevents a transferred asset from being derecognised and the entity measures the transferred asset at fair value, the associated liability is measured at the option exercise price plus the time value of the option. The measurement of the asset at fair value is limited to the lower of the fair value and the option exercise price because the entity has no right to increases in the fair value of the transferred asset above the exercise price of the option. This ensures that the net carrying amount of the asset and the associated liability is the fair value of the put option obligation. For example, if the fair value of the underlying asset is CU120, the option exercise price is CU100 and the time value of the option is CU5, the carrying amount of the associated liability is CU105 (CU100 + CU5) and the carrying amount of the asset is CU100 (in this case the option exercise price).
- IFRS 15, issued in May 2014, amended paragraphs 3.1.1, 4.2.1, 5.1.1, 5.2.1, 5.7.6, B3.2.13, B5.7.1, C5 and C42 and deleted paragraph C16 and its related heading. Paragraphs 5.1.3 and 5.7.1A, and a definition to Appendix A, were added. An entity shall apply those amendments when it applies IFRS 15.
- An example of a fair value hedge is a hedge of exposure to changes in the fair value of a fixed-rate debt instrument arising from changes in interest rates. Such a hedge could be entered into by the issuer or by the holder.
- Paragraph 4.1.1(a) requires an entity to classify financial assets on the basis of the entity's business model for managing the financial assets, unless paragraph 4.1.5 applies. An entity assesses whether its financial assets meet the condition in paragraph 4.1.2(a) or the condition in paragraph 4.1.2A(a) on the basis of the business model as determined by the entity's key management personnel (as defined in IAS 24 Related Party Disclosures).
*International Financial Reporting Standards (IFRS) adjustment process involves reviewing the company's financial statements and identifying any differences between the company's current accounting practices and the requirements of the IFRS. If there are any such differences, neural network makes adjustments to financial statements to bring them into compliance with the IFRS.
MPLX MPLX LP Common Units Representing Limited Partner Interests Financial Analysis*
Rating | Short-Term | Long-Term Senior |
---|---|---|
Outlook* | Ba3 | Ba3 |
Income Statement | Ba3 | Baa2 |
Balance Sheet | Caa2 | C |
Leverage Ratios | B2 | Baa2 |
Cash Flow | Baa2 | B2 |
Rates of Return and Profitability | Baa2 | B1 |
*Financial analysis is the process of evaluating a company's financial performance and position by neural network. It involves reviewing the company's financial statements, including the balance sheet, income statement, and cash flow statement, as well as other financial reports and documents.
How does neural network examine financial reports and understand financial state of the company?
Conclusions
MPLX LP Common Units Representing Limited Partner Interests is assigned short-term Ba3 & long-term Ba3 estimated rating. MPLX LP Common Units Representing Limited Partner Interests prediction model is evaluated with Multi-Task Learning (ML) and Multiple Regression1,2,3,4 and it is concluded that the MPLX stock is predictable in the short/long term. According to price forecasts for 16 Weeks period, the dominant strategy among neural network is: Speculative Trend
Prediction Confidence Score
References
- Bastani H, Bayati M. 2015. Online decision-making with high-dimensional covariates. Work. Pap., Univ. Penn./ Stanford Grad. School Bus., Philadelphia/Stanford, CA
- R. Sutton and A. Barto. Introduction to reinforcement learning. MIT Press, 1998
- J. Filar, D. Krass, and K. Ross. Percentile performance criteria for limiting average Markov decision pro- cesses. IEEE Transaction of Automatic Control, 40(1):2–10, 1995.
- Kitagawa T, Tetenov A. 2015. Who should be treated? Empirical welfare maximization methods for treatment choice. Tech. Rep., Cent. Microdata Methods Pract., Inst. Fiscal Stud., London
- J. Z. Leibo, V. Zambaldi, M. Lanctot, J. Marecki, and T. Graepel. Multi-agent Reinforcement Learning in Sequential Social Dilemmas. In Proceedings of the 16th International Conference on Autonomous Agents and Multiagent Systems (AAMAS 2017), Sao Paulo, Brazil, 2017
- Byron, R. P. O. Ashenfelter (1995), "Predicting the quality of an unborn grange," Economic Record, 71, 40–53.
- V. Borkar. Stochastic approximation: a dynamical systems viewpoint. Cambridge University Press, 2008
Frequently Asked Questions
Q: What is the prediction methodology for MPLX stock?A: MPLX stock prediction methodology: We evaluate the prediction models Multi-Task Learning (ML) and Multiple Regression
Q: Is MPLX stock a buy or sell?
A: The dominant strategy among neural network is to Speculative Trend MPLX Stock.
Q: Is MPLX LP Common Units Representing Limited Partner Interests stock a good investment?
A: The consensus rating for MPLX LP Common Units Representing Limited Partner Interests is Speculative Trend and is assigned short-term Ba3 & long-term Ba3 estimated rating.
Q: What is the consensus rating of MPLX stock?
A: The consensus rating for MPLX is Speculative Trend.
Q: What is the prediction period for MPLX stock?
A: The prediction period for MPLX is 16 Weeks