Dominant Strategy : Sell
Time series to forecast n: 13 Jun 2023 for 16 Weeks
Methodology : Transductive Learning (ML)
Abstract
Coliseum Acquisition Corp. Class A Ordinary Share prediction model is evaluated with Transductive Learning (ML) and Statistical Hypothesis Testing1,2,3,4 and it is concluded that the MITA stock is predictable in the short/long term. Transductive learning is a supervised machine learning (ML) method in which the model is trained on both labeled and unlabeled data. The goal of transductive learning is to predict the labels of the unlabeled data. Transductive learning is a hybrid of inductive and semi-supervised learning. Inductive learning algorithms are trained on labeled data only, while semi-supervised learning algorithms are trained on a combination of labeled and unlabeled data. Transductive learning algorithms can achieve better performance than inductive learning algorithms on tasks where there is a small amount of labeled data. This is because transductive learning algorithms can use the unlabeled data to help them learn the relationships between the features and the labels. According to price forecasts for 16 Weeks period, the dominant strategy among neural network is: Sell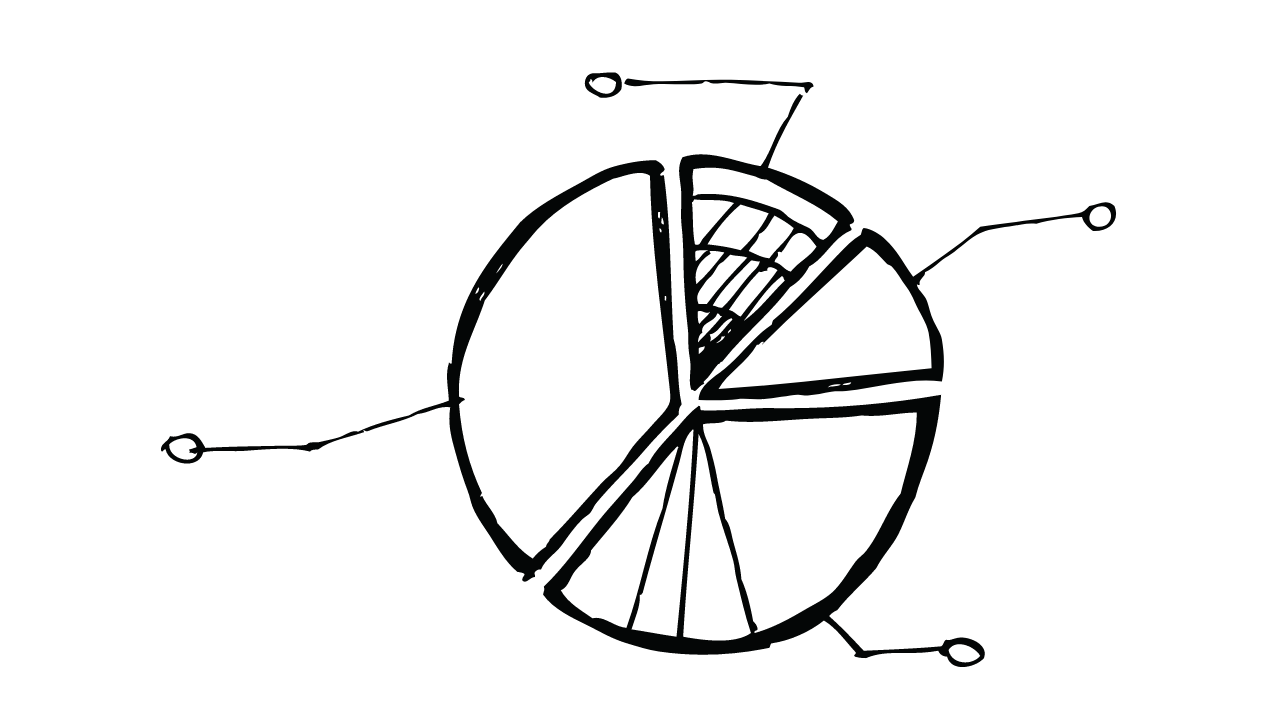
Key Points
- Probability Distribution
- What is neural prediction?
- How do you pick a stock?
MITA Target Price Prediction Modeling Methodology
We consider Coliseum Acquisition Corp. Class A Ordinary Share Decision Process with Transductive Learning (ML) where A is the set of discrete actions of MITA stock holders, F is the set of discrete states, P : S × F × S → R is the transition probability distribution, R : S × F → R is the reaction function, and γ ∈ [0, 1] is a move factor for expectation.1,2,3,4
F(Statistical Hypothesis Testing)5,6,7= X R(Transductive Learning (ML)) X S(n):→ 16 Weeks
n:Time series to forecast
p:Price signals of MITA stock
j:Nash equilibria (Neural Network)
k:Dominated move
a:Best response for target price
Transductive Learning (ML)
Transductive learning is a supervised machine learning (ML) method in which the model is trained on both labeled and unlabeled data. The goal of transductive learning is to predict the labels of the unlabeled data. Transductive learning is a hybrid of inductive and semi-supervised learning. Inductive learning algorithms are trained on labeled data only, while semi-supervised learning algorithms are trained on a combination of labeled and unlabeled data. Transductive learning algorithms can achieve better performance than inductive learning algorithms on tasks where there is a small amount of labeled data. This is because transductive learning algorithms can use the unlabeled data to help them learn the relationships between the features and the labels.Statistical Hypothesis Testing
Statistical hypothesis testing is a process used to determine whether there is enough evidence to support a claim about a population based on a sample. The process involves making two hypotheses, a null hypothesis and an alternative hypothesis, and then collecting data and using statistical tests to determine which hypothesis is more likely to be true. The null hypothesis is the statement that there is no difference between the population and the sample. The alternative hypothesis is the statement that there is a difference between the population and the sample. The statistical test is used to calculate a p-value, which is the probability of obtaining the observed data or more extreme data if the null hypothesis is true. A p-value of less than 0.05 is typically considered to be statistically significant, which means that there is less than a 5% chance of obtaining the observed data or more extreme data if the null hypothesis is true.
For further technical information as per how our model work we invite you to visit the article below:
How do AC Investment Research machine learning (predictive) algorithms actually work?
MITA Stock Forecast (Buy or Sell) for 16 Weeks
Sample Set: Neural NetworkStock/Index: MITA Coliseum Acquisition Corp. Class A Ordinary Share
Time series to forecast n: 13 Jun 2023 for 16 Weeks
According to price forecasts for 16 Weeks period, the dominant strategy among neural network is: Sell
X axis: *Likelihood% (The higher the percentage value, the more likely the event will occur.)
Y axis: *Potential Impact% (The higher the percentage value, the more likely the price will deviate.)
Z axis (Grey to Black): *Technical Analysis%
IFRS Reconciliation Adjustments for Coliseum Acquisition Corp. Class A Ordinary Share
- The accounting for the time value of options in accordance with paragraph 6.5.15 applies only to the extent that the time value relates to the hedged item (aligned time value). The time value of an option relates to the hedged item if the critical terms of the option (such as the nominal amount, life and underlying) are aligned with the hedged item. Hence, if the critical terms of the option and the hedged item are not fully aligned, an entity shall determine the aligned time value, ie how much of the time value included in the premium (actual time value) relates to the hedged item (and therefore should be treated in accordance with paragraph 6.5.15). An entity determines the aligned time value using the valuation of the option that would have critical terms that perfectly match the hedged item.
- When using historical credit loss experience in estimating expected credit losses, it is important that information about historical credit loss rates is applied to groups that are defined in a manner that is consistent with the groups for which the historical credit loss rates were observed. Consequently, the method used shall enable each group of financial assets to be associated with information about past credit loss experience in groups of financial assets with similar risk characteristics and with relevant observable data that reflects current conditions.
- Paragraphs 6.9.7–6.9.13 provide exceptions to the requirements specified in those paragraphs only. An entity shall apply all other hedge accounting requirements in this Standard, including the qualifying criteria in paragraph 6.4.1, to hedging relationships that were directly affected by interest rate benchmark reform.
- If a financial instrument that was previously recognised as a financial asset is measured at fair value through profit or loss and its fair value decreases below zero, it is a financial liability measured in accordance with paragraph 4.2.1. However, hybrid contracts with hosts that are assets within the scope of this Standard are always measured in accordance with paragraph 4.3.2.
*International Financial Reporting Standards (IFRS) adjustment process involves reviewing the company's financial statements and identifying any differences between the company's current accounting practices and the requirements of the IFRS. If there are any such differences, neural network makes adjustments to financial statements to bring them into compliance with the IFRS.
Conclusions
Coliseum Acquisition Corp. Class A Ordinary Share is assigned short-term Ba1 & long-term Ba1 estimated rating. Coliseum Acquisition Corp. Class A Ordinary Share prediction model is evaluated with Transductive Learning (ML) and Statistical Hypothesis Testing1,2,3,4 and it is concluded that the MITA stock is predictable in the short/long term. According to price forecasts for 16 Weeks period, the dominant strategy among neural network is: Sell
MITA Coliseum Acquisition Corp. Class A Ordinary Share Financial Analysis*
Rating | Short-Term | Long-Term Senior |
---|---|---|
Outlook* | Ba1 | Ba1 |
Income Statement | Caa2 | Baa2 |
Balance Sheet | Caa2 | Baa2 |
Leverage Ratios | Baa2 | B3 |
Cash Flow | C | Ba3 |
Rates of Return and Profitability | Baa2 | Caa2 |
*Financial analysis is the process of evaluating a company's financial performance and position by neural network. It involves reviewing the company's financial statements, including the balance sheet, income statement, and cash flow statement, as well as other financial reports and documents.
How does neural network examine financial reports and understand financial state of the company?
Prediction Confidence Score
References
- Athey S, Blei D, Donnelly R, Ruiz F. 2017b. Counterfactual inference for consumer choice across many prod- uct categories. AEA Pap. Proc. 108:64–67
- Athey S. 2019. The impact of machine learning on economics. In The Economics of Artificial Intelligence: An Agenda, ed. AK Agrawal, J Gans, A Goldfarb. Chicago: Univ. Chicago Press. In press
- Friedberg R, Tibshirani J, Athey S, Wager S. 2018. Local linear forests. arXiv:1807.11408 [stat.ML]
- Andrews, D. W. K. W. Ploberger (1994), "Optimal tests when a nuisance parameter is present only under the alternative," Econometrica, 62, 1383–1414.
- Abadir, K. M., K. Hadri E. Tzavalis (1999), "The influence of VAR dimensions on estimator biases," Econometrica, 67, 163–181.
- H. Khalil and J. Grizzle. Nonlinear systems, volume 3. Prentice hall Upper Saddle River, 2002.
- LeCun Y, Bengio Y, Hinton G. 2015. Deep learning. Nature 521:436–44
Frequently Asked Questions
Q: What is the prediction methodology for MITA stock?A: MITA stock prediction methodology: We evaluate the prediction models Transductive Learning (ML) and Statistical Hypothesis Testing
Q: Is MITA stock a buy or sell?
A: The dominant strategy among neural network is to Sell MITA Stock.
Q: Is Coliseum Acquisition Corp. Class A Ordinary Share stock a good investment?
A: The consensus rating for Coliseum Acquisition Corp. Class A Ordinary Share is Sell and is assigned short-term Ba1 & long-term Ba1 estimated rating.
Q: What is the consensus rating of MITA stock?
A: The consensus rating for MITA is Sell.
Q: What is the prediction period for MITA stock?
A: The prediction period for MITA is 16 Weeks