Dominant Strategy : Buy
Time series to forecast n: 15 Jun 2023 for 16 Weeks
Methodology : Multi-Instance Learning (ML)
Abstract
Standard Lithium Ltd. prediction model is evaluated with Multi-Instance Learning (ML) and Statistical Hypothesis Testing1,2,3,4 and it is concluded that the SLI:TSXV stock is predictable in the short/long term. Multi-instance learning (MIL) is a machine learning (ML) problem where a dataset consists of multiple instances, and each instance is associated with a single label. The goal of MIL is to learn a model that can predict the label of a new instance based on the labels of the instances that it is similar to. MIL is a challenging problem because the instances in a dataset are not labeled individually. This means that the model cannot simply learn a mapping from the features of an instance to its label. Instead, the model must learn a way to combine the features of multiple instances to predict the label of a new instance. According to price forecasts for 16 Weeks period, the dominant strategy among neural network is: Buy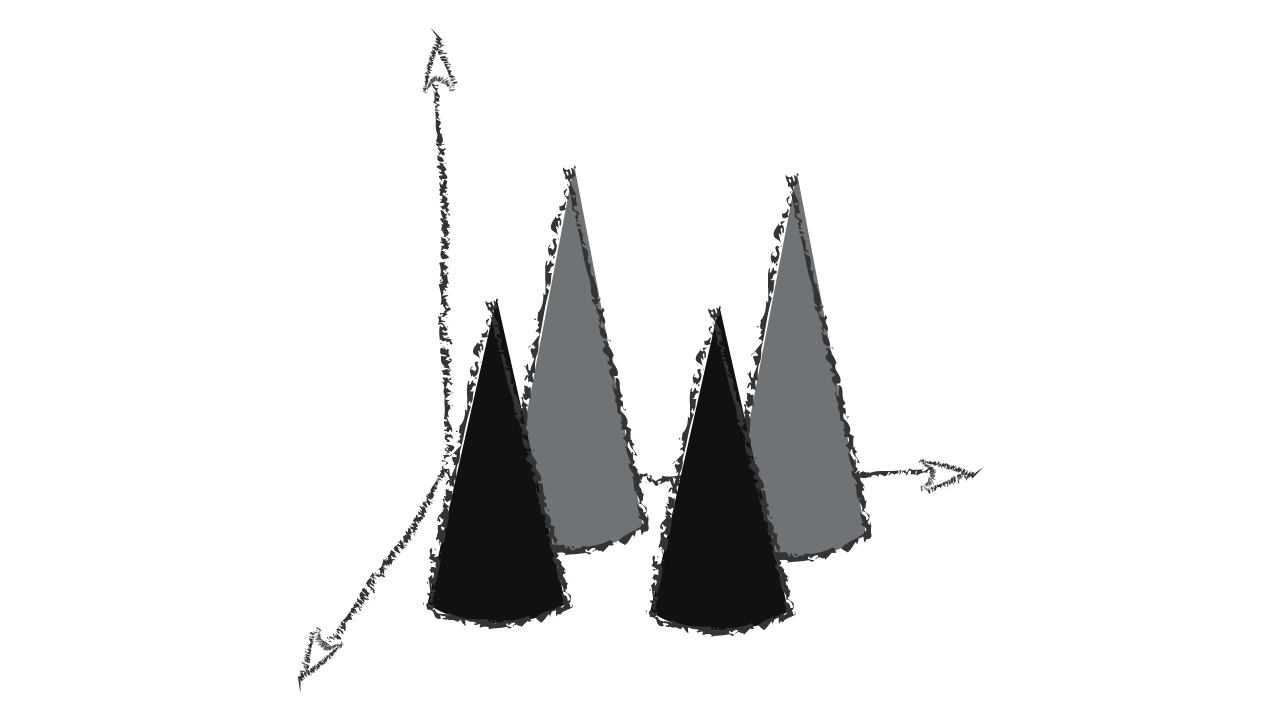
Key Points
- Can neural networks predict stock market?
- Buy, Sell and Hold Signals
- What is prediction in deep learning?
SLI:TSXV Target Price Prediction Modeling Methodology
We consider Standard Lithium Ltd. Decision Process with Multi-Instance Learning (ML) where A is the set of discrete actions of SLI:TSXV stock holders, F is the set of discrete states, P : S × F × S → R is the transition probability distribution, R : S × F → R is the reaction function, and γ ∈ [0, 1] is a move factor for expectation.1,2,3,4
F(Statistical Hypothesis Testing)5,6,7= X R(Multi-Instance Learning (ML)) X S(n):→ 16 Weeks
n:Time series to forecast
p:Price signals of SLI:TSXV stock
j:Nash equilibria (Neural Network)
k:Dominated move
a:Best response for target price
Multi-Instance Learning (ML)
Multi-instance learning (MIL) is a machine learning (ML) problem where a dataset consists of multiple instances, and each instance is associated with a single label. The goal of MIL is to learn a model that can predict the label of a new instance based on the labels of the instances that it is similar to. MIL is a challenging problem because the instances in a dataset are not labeled individually. This means that the model cannot simply learn a mapping from the features of an instance to its label. Instead, the model must learn a way to combine the features of multiple instances to predict the label of a new instance.Statistical Hypothesis Testing
Statistical hypothesis testing is a process used to determine whether there is enough evidence to support a claim about a population based on a sample. The process involves making two hypotheses, a null hypothesis and an alternative hypothesis, and then collecting data and using statistical tests to determine which hypothesis is more likely to be true. The null hypothesis is the statement that there is no difference between the population and the sample. The alternative hypothesis is the statement that there is a difference between the population and the sample. The statistical test is used to calculate a p-value, which is the probability of obtaining the observed data or more extreme data if the null hypothesis is true. A p-value of less than 0.05 is typically considered to be statistically significant, which means that there is less than a 5% chance of obtaining the observed data or more extreme data if the null hypothesis is true.
For further technical information as per how our model work we invite you to visit the article below:
How do AC Investment Research machine learning (predictive) algorithms actually work?
SLI:TSXV Stock Forecast (Buy or Sell) for 16 Weeks
Sample Set: Neural NetworkStock/Index: SLI:TSXV Standard Lithium Ltd.
Time series to forecast n: 15 Jun 2023 for 16 Weeks
According to price forecasts for 16 Weeks period, the dominant strategy among neural network is: Buy
X axis: *Likelihood% (The higher the percentage value, the more likely the event will occur.)
Y axis: *Potential Impact% (The higher the percentage value, the more likely the price will deviate.)
Z axis (Grey to Black): *Technical Analysis%
IFRS Reconciliation Adjustments for Standard Lithium Ltd.
- The definition of a derivative refers to non-financial variables that are not specific to a party to the contract. These include an index of earthquake losses in a particular region and an index of temperatures in a particular city. Non-financial variables specific to a party to the contract include the occurrence or non-occurrence of a fire that damages or destroys an asset of a party to the contract. A change in the fair value of a non-financial asset is specific to the owner if the fair value reflects not only changes in market prices for such assets (a financial variable) but also the condition of the specific non-financial asset held (a non-financial variable). For example, if a guarantee of the residual value of a specific car exposes the guarantor to the risk of changes in the car's physical condition, the change in that residual value is specific to the owner of the car.
- The requirement that an economic relationship exists means that the hedging instrument and the hedged item have values that generally move in the opposite direction because of the same risk, which is the hedged risk. Hence, there must be an expectation that the value of the hedging instrument and the value of the hedged item will systematically change in response to movements in either the same underlying or underlyings that are economically related in such a way that they respond in a similar way to the risk that is being hedged (for example, Brent and WTI crude oil).
- When measuring the fair values of the part that continues to be recognised and the part that is derecognised for the purposes of applying paragraph 3.2.13, an entity applies the fair value measurement requirements in IFRS 13 Fair Value Measurement in addition to paragraph 3.2.14.
- An entity that first applies these amendments at the same time it first applies this Standard shall apply paragraphs 7.2.1–7.2.28 instead of paragraphs 7.2.31–7.2.34.
*International Financial Reporting Standards (IFRS) adjustment process involves reviewing the company's financial statements and identifying any differences between the company's current accounting practices and the requirements of the IFRS. If there are any such differences, neural network makes adjustments to financial statements to bring them into compliance with the IFRS.
Conclusions
Standard Lithium Ltd. is assigned short-term Ba1 & long-term Ba1 estimated rating. Standard Lithium Ltd. prediction model is evaluated with Multi-Instance Learning (ML) and Statistical Hypothesis Testing1,2,3,4 and it is concluded that the SLI:TSXV stock is predictable in the short/long term. According to price forecasts for 16 Weeks period, the dominant strategy among neural network is: Buy
SLI:TSXV Standard Lithium Ltd. Financial Analysis*
Rating | Short-Term | Long-Term Senior |
---|---|---|
Outlook* | Ba1 | Ba1 |
Income Statement | Ba2 | Caa2 |
Balance Sheet | Baa2 | B3 |
Leverage Ratios | Baa2 | Baa2 |
Cash Flow | B2 | B2 |
Rates of Return and Profitability | C | Caa2 |
*Financial analysis is the process of evaluating a company's financial performance and position by neural network. It involves reviewing the company's financial statements, including the balance sheet, income statement, and cash flow statement, as well as other financial reports and documents.
How does neural network examine financial reports and understand financial state of the company?
Prediction Confidence Score
References
- Barkan O. 2016. Bayesian neural word embedding. arXiv:1603.06571 [math.ST]
- A. Tamar and S. Mannor. Variance adjusted actor critic algorithms. arXiv preprint arXiv:1310.3697, 2013.
- Mnih A, Teh YW. 2012. A fast and simple algorithm for training neural probabilistic language models. In Proceedings of the 29th International Conference on Machine Learning, pp. 419–26. La Jolla, CA: Int. Mach. Learn. Soc.
- Abadir, K. M., K. Hadri E. Tzavalis (1999), "The influence of VAR dimensions on estimator biases," Econometrica, 67, 163–181.
- Athey S, Imbens GW. 2017a. The econometrics of randomized experiments. In Handbook of Economic Field Experiments, Vol. 1, ed. E Duflo, A Banerjee, pp. 73–140. Amsterdam: Elsevier
- Efron B, Hastie T. 2016. Computer Age Statistical Inference, Vol. 5. Cambridge, UK: Cambridge Univ. Press
- Scott SL. 2010. A modern Bayesian look at the multi-armed bandit. Appl. Stoch. Models Bus. Ind. 26:639–58
Frequently Asked Questions
Q: What is the prediction methodology for SLI:TSXV stock?A: SLI:TSXV stock prediction methodology: We evaluate the prediction models Multi-Instance Learning (ML) and Statistical Hypothesis Testing
Q: Is SLI:TSXV stock a buy or sell?
A: The dominant strategy among neural network is to Buy SLI:TSXV Stock.
Q: Is Standard Lithium Ltd. stock a good investment?
A: The consensus rating for Standard Lithium Ltd. is Buy and is assigned short-term Ba1 & long-term Ba1 estimated rating.
Q: What is the consensus rating of SLI:TSXV stock?
A: The consensus rating for SLI:TSXV is Buy.
Q: What is the prediction period for SLI:TSXV stock?
A: The prediction period for SLI:TSXV is 16 Weeks